AnoCSR-A Convolutional Sparse Reconstructive Noise-Robust Framework for Industrial Anomaly Detection
PATTERN RECOGNITION AND COMPUTER VISION, PRCV 2023, PT V(2024)
摘要
Industrial anomaly detection involves the identification and localization of abnormal regions in images, of which the core challenge is modeling normal data in appropriate ways. Inspired by dictionary learning, we propose a convolutional sparse reconstructive noise-robust framework, named AnoCSR. The proposed convolutional sparse encoding block (CSE-Block) in AnoCSR treats the convolutional dictionary as learnable parameters, where the convolutional kernels serve as the atoms. By training on normal samples, we optimize the parameters of the CSE-Block to extract optimal sparse codes. The CSE-Block stacks to form a convolutional sparse reconstructive network (CSR-Net) to progressively extract the sparse code and reconstruct the input image inversely. This enables effective reconstruction of normal samples while inadequately reconstructing abnormal regions, thereby facilitating anomaly detection and localization. The CSR-Net is parallelly connected with the downstream Localizer, forming an end-to-end framework. Our experimental results demonstrate that AnoCSR achieves performance comparable to state-of-the-art image reconstruction-based methods on the MVTecAD dataset. Moreover, considering that noise may affect the modeling of normal data and the discrimination of anomalies, we conduct the simulated noise resistance experiment. The result demonstrates that AnoCSR significantly outperforms other similar methods, indicating its strong noise robustness in addition to its outstanding performance.
更多查看译文
关键词
Anomaly Detection,Sparse Code,Dictionary Learning
AI 理解论文
溯源树
样例
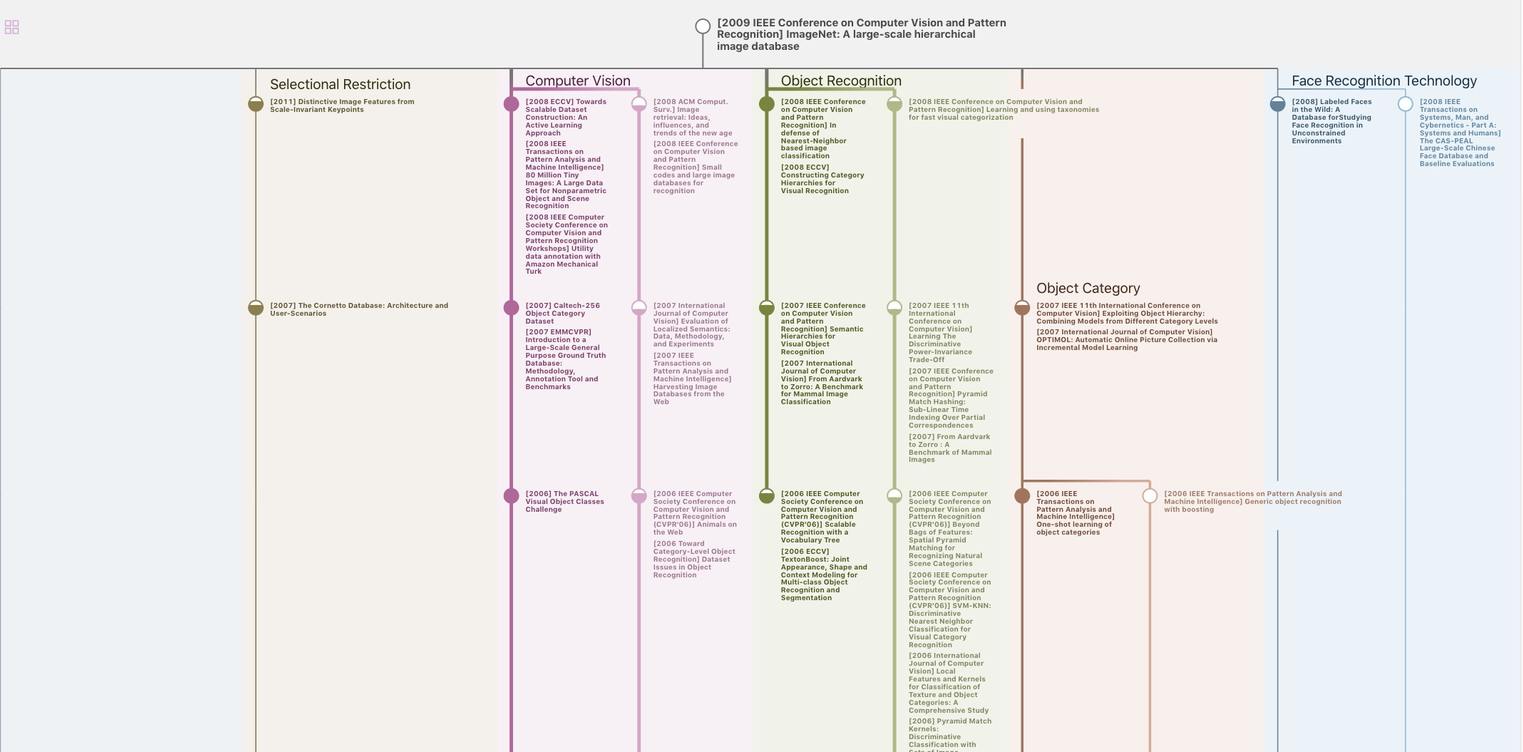
生成溯源树,研究论文发展脉络
Chat Paper
正在生成论文摘要