Generalizable and Accurate 6D Object Pose Estimation Network
PATTERN RECOGNITION AND COMPUTER VISION, PRCV 2023, PT III(2024)
Abstract
6D object pose estimation is an important task in computer vision, and the task of estimating 6D object pose from a single RGB image is even more challenging. Many methods use deep learning to acquire 2D feature points from images to establish 2D-3D correspondences, and further predict 6D object pose with Perspective-n-Points (PnP) algorithm. However, most of these methods have problems with inaccurate acquisition of feature points, poor generality of the network and difficulty in end-to-end training of the network. In this paper, we design an end-to-end differentiable network for 6D object pose estimation. We propose Random Offset Distraction (ROD) and Full Convolution Asymmetric Feature Extractor (FCAFE) with the Probabilistic Perspective-n-Points (ProPnP) algorithm to improve the accuracy and robustness of 6D object pose estimation. Experiments show that our method achieves a new state-of-the-art result on the LineMOD dataset, with an accuracy of 97.42% in the ADD(-S) metric. Our approach is also very competitive on the Occlusion LineMOD dataset.
MoreTranslated text
Key words
6D Object Pose Estimation,Coordinates-based Method,RGB Data
AI Read Science
Must-Reading Tree
Example
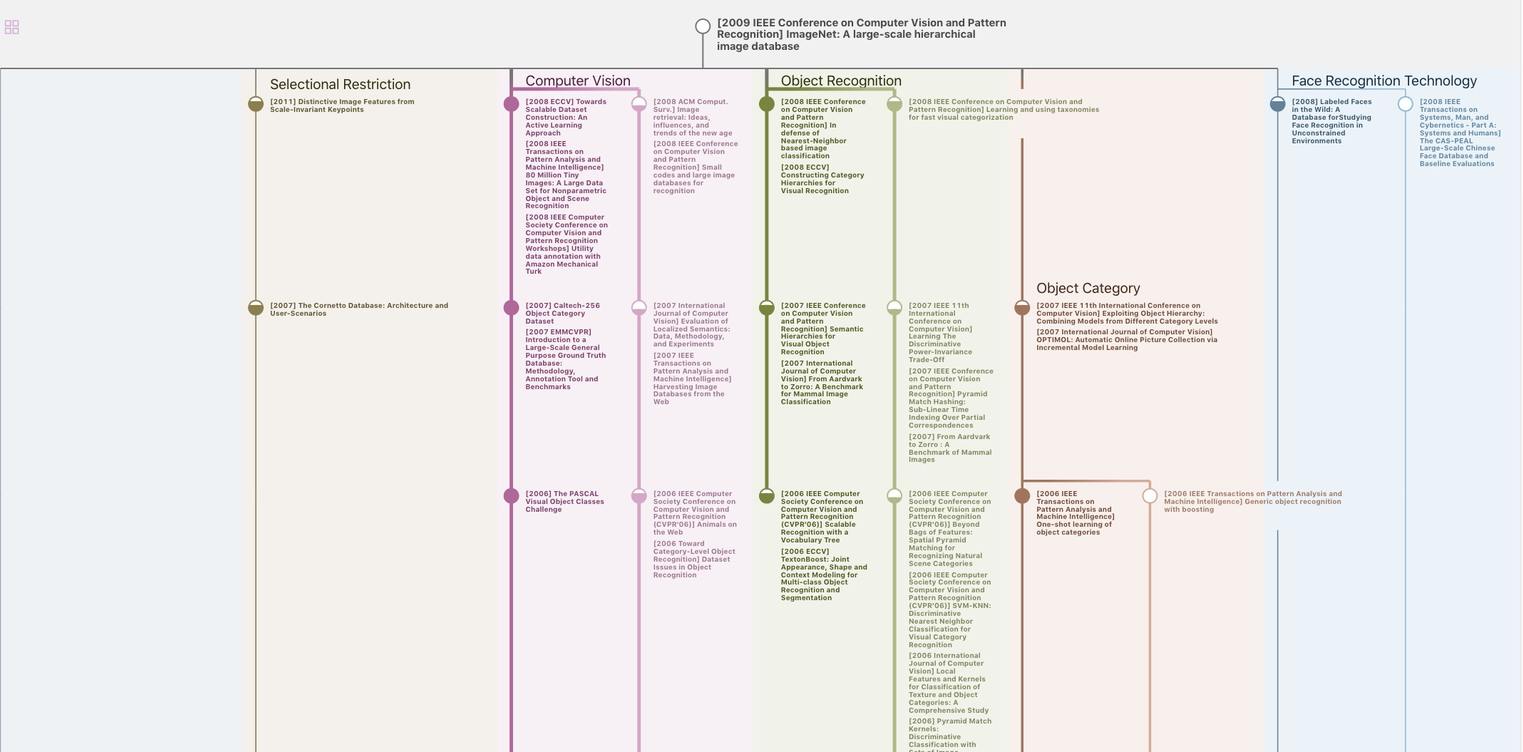
Generate MRT to find the research sequence of this paper
Chat Paper
Summary is being generated by the instructions you defined