TiAM-GAN: Titanium Alloy Microstructure Image Generation Network
PATTERN RECOGNITION AND COMPUTER VISION, PRCV 2023, PT III(2024)
摘要
The generation of titanium alloy microstructure images through mechanical properties is of great value to the research and production of titanium alloy materials. The appearance of GAN provides the possibility for image generation. However, there is currently no work related to handling multiple continuous labels for microstructure images. This paper presents a multi-label titanium alloy microstructure image generation network(TiAM-GAN). The TiAM-GAN proposed in this paper contains two sub-networks, a generation network for simple textures, which is based on the existing generation adversarial network, we reconstruct the loss function for multi-label continuous variables and deduce the error bound. Another microstructure image generation network for complex textures uses a mixture density network to learn the labels-to-noise mapping, and a deep convolution generation adversarial network is used to learn the noise-to-image mapping, then the noise output by the mixture density network will input to the deep convolution generation adversarial network to generate the image. Finally, we compared our method with existing methods qualitatively and quantitatively, which shows our method can achieve better results.
更多查看译文
关键词
Titanium Alloy Microstructure,Multi-Label,Image Generation,Generative Adversarial Network
AI 理解论文
溯源树
样例
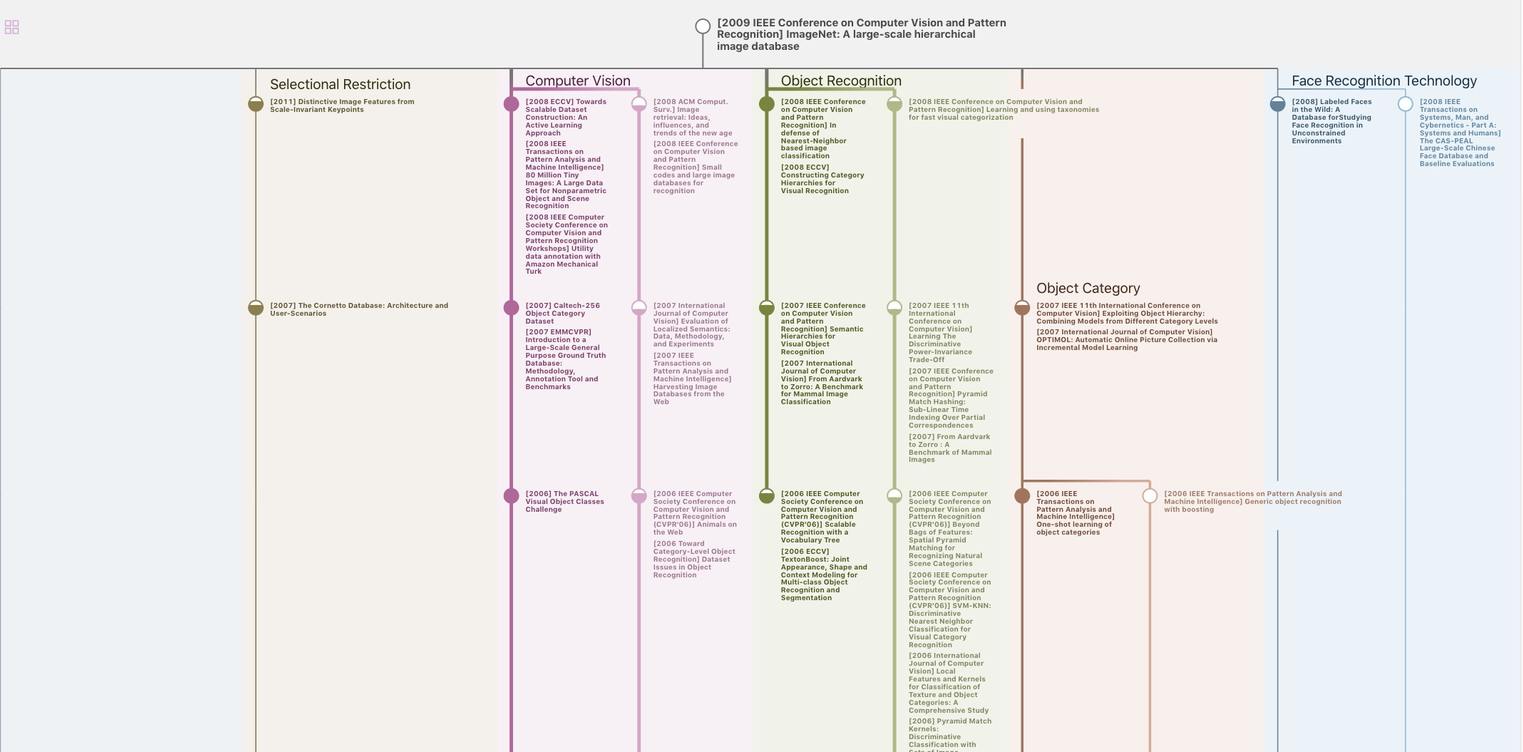
生成溯源树,研究论文发展脉络
Chat Paper
正在生成论文摘要