Unified fair federated learning for digital healthcare
PATTERNS(2024)
摘要
Federated learning (FL) is a promising approach for healthcare institutions to train high -quality medical models collaboratively while protecting sensitive data privacy. However, FL models encounter fairness issues at diverse levels, leading to performance disparities across different subpopulations. To address this, we propose Federated Learning with Unified Fairness Objective (FedUFO), a unified framework consolidating diverse fairness levels within FL. By leveraging distributionally robust optimization and a unified uncertainty set, it ensures consistent performance across all subpopulations and enhances the overall efficacy of FL in healthcare and other domains while maintaining accuracy levels comparable with those of existing methods. Our model was validated by applying it to four digital healthcare tasks using real -world datasets in federated settings. Our collaborative machine learning paradigm not only promotes artificial intelligence in digital healthcare but also fosters social equity by embodying fairness.
更多查看译文
关键词
federated learning,algorithmic fairness,digital healthcare
AI 理解论文
溯源树
样例
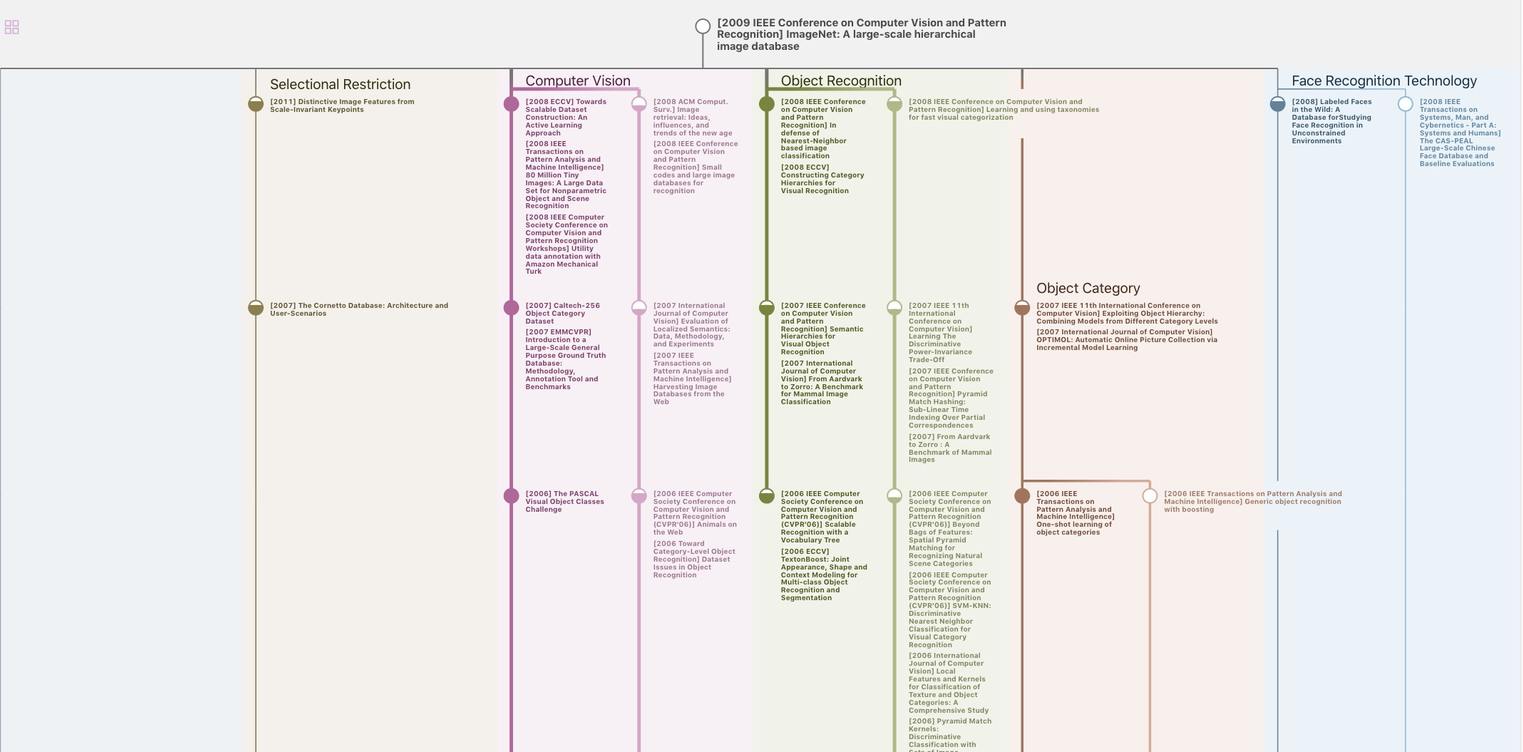
生成溯源树,研究论文发展脉络
Chat Paper
正在生成论文摘要