Efficient Point-Based Single Scale 3D Object Detection from Traffic Scenes
PATTERN RECOGNITION AND COMPUTER VISION, PRCV 2023, PT II(2024)
摘要
In the field of 3D object detection, the point-based method faces significant limitations due to the need to process large-scale collections of irregular point clouds, resulting in reduced inference speed. To address this issue, we propose an efficient single-scale single-stage 3D object detection algorithm called SS-3DSSD. Our method eliminates the time-consuming multi-scale feature extraction module used in PointNet++ and adopts an efficient single-scale feature extraction method based on neighborhood-attention, significantly improving the model's inference speed. Additionally, we introduce a learning-based sampling method to overcome the limited receptive fields of single-scale methods and a multi-level context feature grouping module to meet varying feature requirements at different levels. On the KITTI test set, our method achieves an inference speed of 66.7 frames per second on the RTX 2080Ti, with an average precision of 81.35% for the moderate difficulty car category. This represents a better balance between inference speed and detection accuracy, offering promising implications for real-time 3D object detection applications.
更多查看译文
关键词
3D Object Detection,Neighborhood Attention,Feature Extraction,Deep Learning
AI 理解论文
溯源树
样例
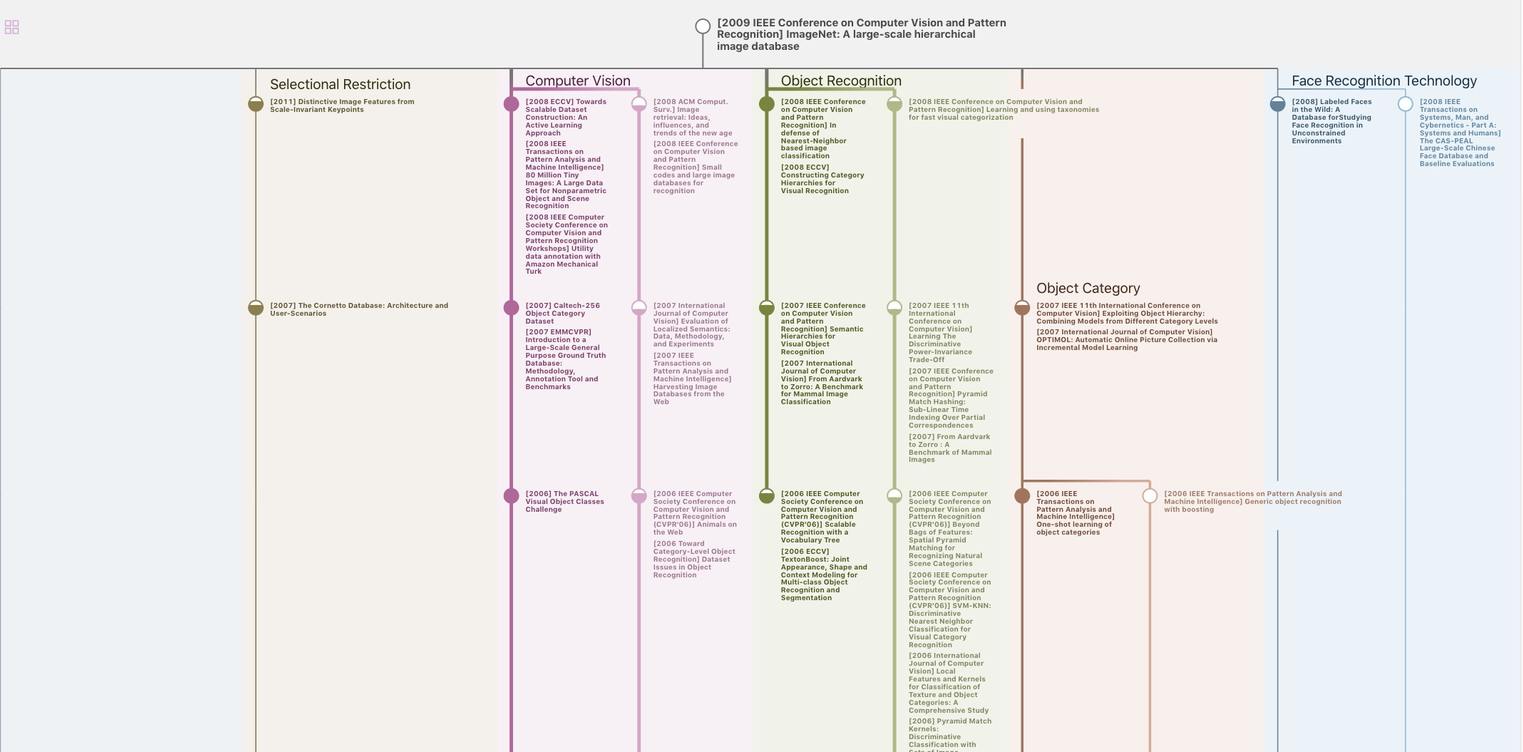
生成溯源树,研究论文发展脉络
Chat Paper
正在生成论文摘要