Deep Stereo Matching with Superpixel Based Feature and Cost
PATTERN RECOGNITION AND COMPUTER VISION, PRCV 2023, PT II(2024)
Abstract
Previous stereo methods achieved state-of-the-art performances but are still difficult to handle the well-known edge-fattening issue at depth discontinuity regions. In this paper, we proposed differentiable superpixel-based feature and cost aggregation (DSFCA) networks for stereo matching. More specifically, we generated the superpixel maps using the simple linear iterative clustering (SLIC) method and used the efficient feature extraction network to extract the dynamic scale unary features of stereo pair images. Next, we exploited the edge or contour feature information of superpixel maps to aggregate the unary features using the differentiable superpixel-based feature aggregation (DSFA) module. The aggregated features better represent the similarity of stereo pair image feature maps and produce high-quality initial cost volumes. Furthermore, the matching cost is also aggregated by the proposed differentiable superpixel-based cost aggregation (DSCA) module. The experimental results demonstrate that the proposed method outperforms previous methods on the KITTI 2012, KITTI 2015, and SceneFlow datasets.
MoreTranslated text
Key words
Stereo Matching,Superpixels,Feature Aggregation,Cost Aggregation
AI Read Science
Must-Reading Tree
Example
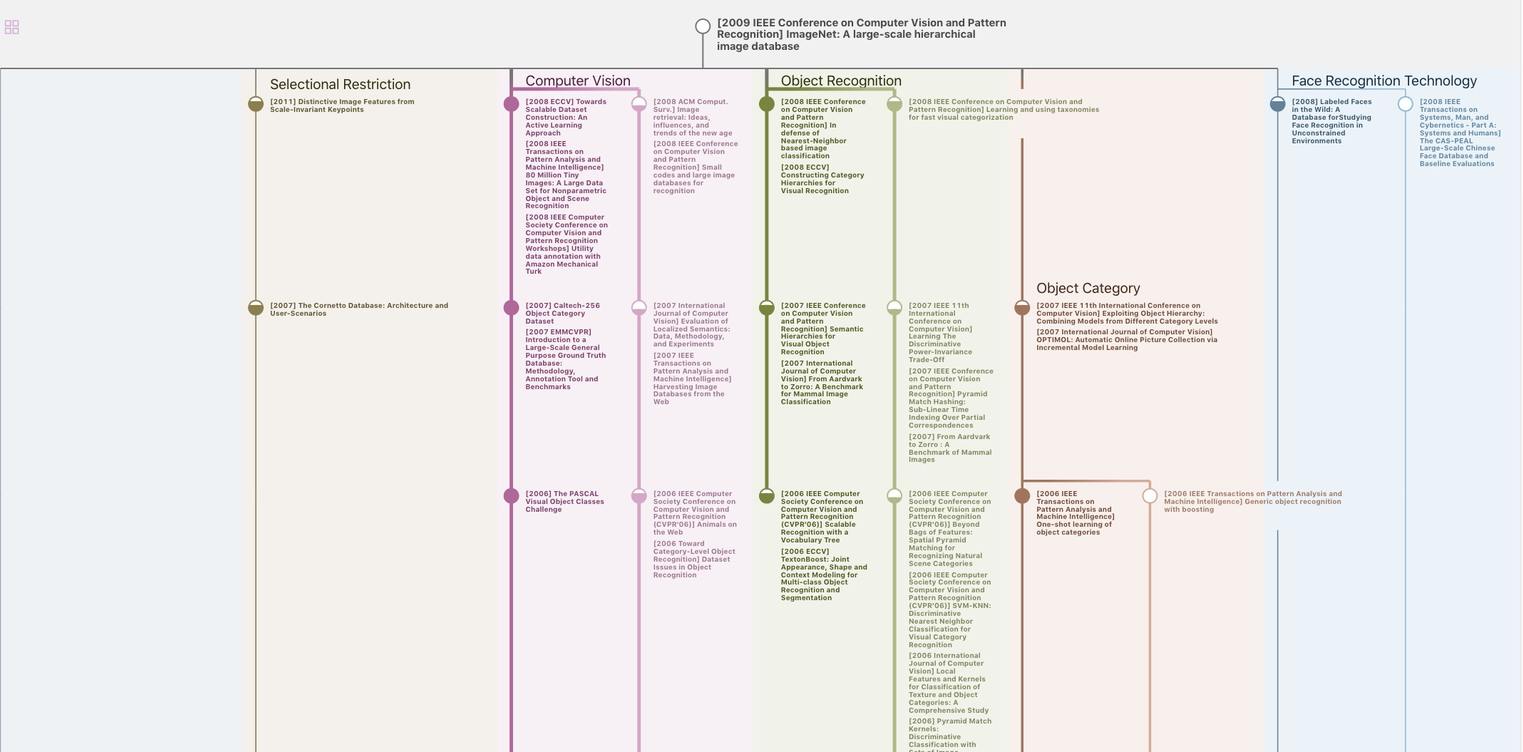
Generate MRT to find the research sequence of this paper
Chat Paper
Summary is being generated by the instructions you defined