Image Priors Assisted Pre-training for Point Cloud Shape Analysis
PATTERN RECOGNITION AND COMPUTER VISION, PRCV 2023, PT I(2024)
Abstract
Self-Supervised Learning (SSL) is a viable technique to unleash the scalability and generalization of the network. Nevertheless, the representations learned by existing 3D SSL are still insufficient for point cloud shape analysis. Compared to point clouds, images have better strength in providing fine-grained semantic information. In this paper, we propose a framework of cross-modal SSL, enabling knowledge transfer across modalities. Specifically, two self-supervised tasks are meticulously designed. The former constructs instance-level consistency, which computes the similarity between objects to discriminate instances of different modalities. The latter constructs cluster-level consistency, which mines category coherence by grouping point clouds and corresponding images into the same semantic regions. By jointly learning feature representations and cluster assignments, the model incorporates the latent category information to reduce the intra-cluster variance as well as increase the inter-cluster variance. Extensive experimental results demonstrate the effectiveness on three representative datasets.
MoreTranslated text
Key words
Image Priors,Instance-Level,Cluster-Level
AI Read Science
Must-Reading Tree
Example
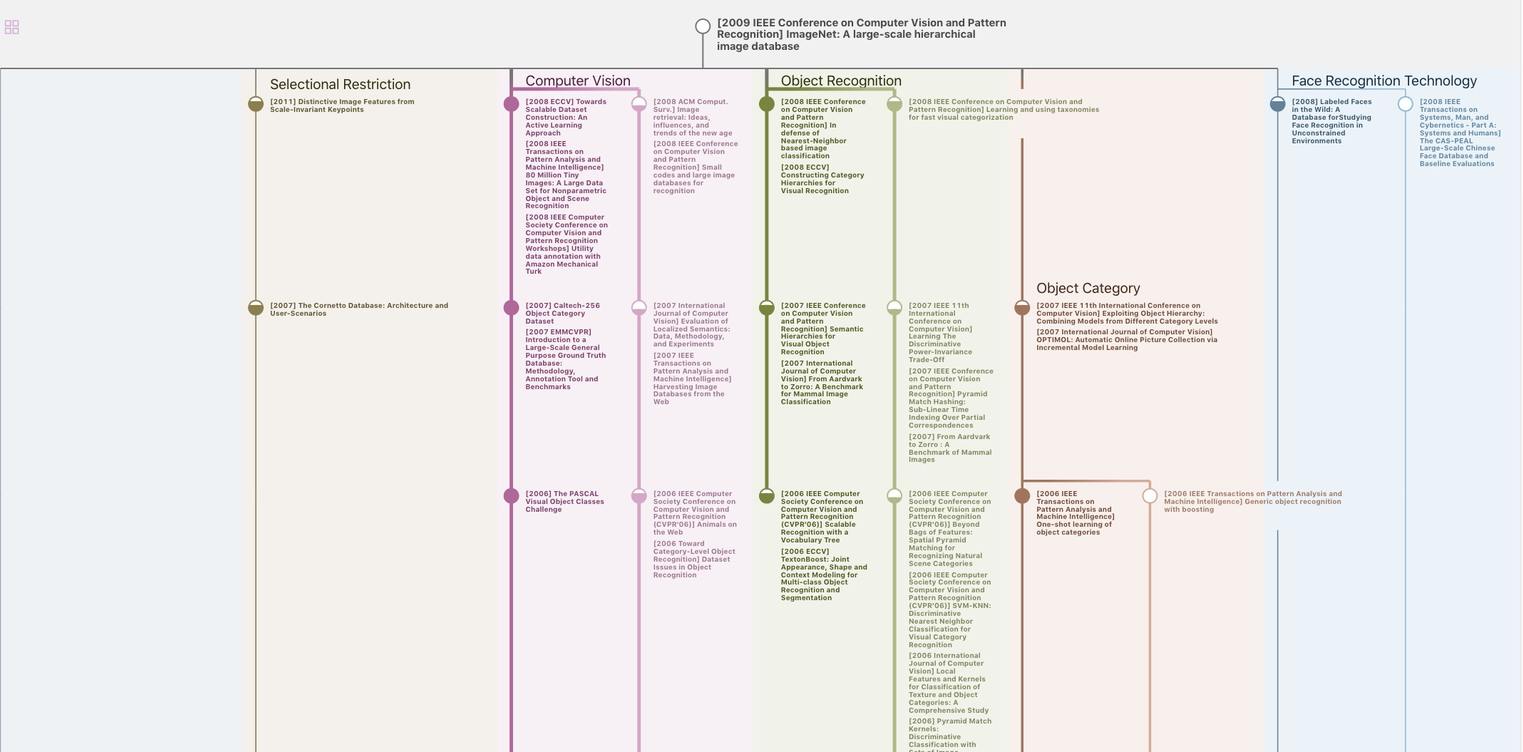
Generate MRT to find the research sequence of this paper
Chat Paper
Summary is being generated by the instructions you defined