A sequential Monte Carlo algorithm for data assimilation problems in ecology
arxiv(2024)
Abstract
1. Temporal trends in species distributions are necessary for monitoring changes in biodiversity, which aids policymakers and conservationists in making informed decisions. Dynamic species distribution models are often fitted to ecological time series data using Markov Chain Monte Carlo algorithms to produce these temporal trends. However, the fitted models can be time-consuming to produce and run, making it inefficient to refit them as new observations become available. 2. We propose an algorithm that updates model parameters and the latent state distribution (e.g. true occupancy) using the saved information from a previously fitted model. This algorithm capitalises on the strength of importance sampling to generate new posterior samples of interest by updating the model output. The algorithm was validated with simulation studies on linear Gaussian state space models and occupancy models, and we applied the framework to Crested Tits in Switzerland and Yellow Meadow Ants in the UK. 3. We found that models updated with the proposed algorithm captured the true model parameters and latent state values as good as the models refitted to the expanded dataset. Moreover, the updated models were much faster to run and preserved the trajectory of the derived quantities. 4. The proposed approach serves as an alternative to conventional methods for updating state-space models (SSMs), and it is most beneficial when the fitted SSMs have a long run time. Overall, we provide a Monte Carlo algorithm to efficiently update complex models, a key issue in developing biodiversity models and indicators.
MoreTranslated text
AI Read Science
Must-Reading Tree
Example
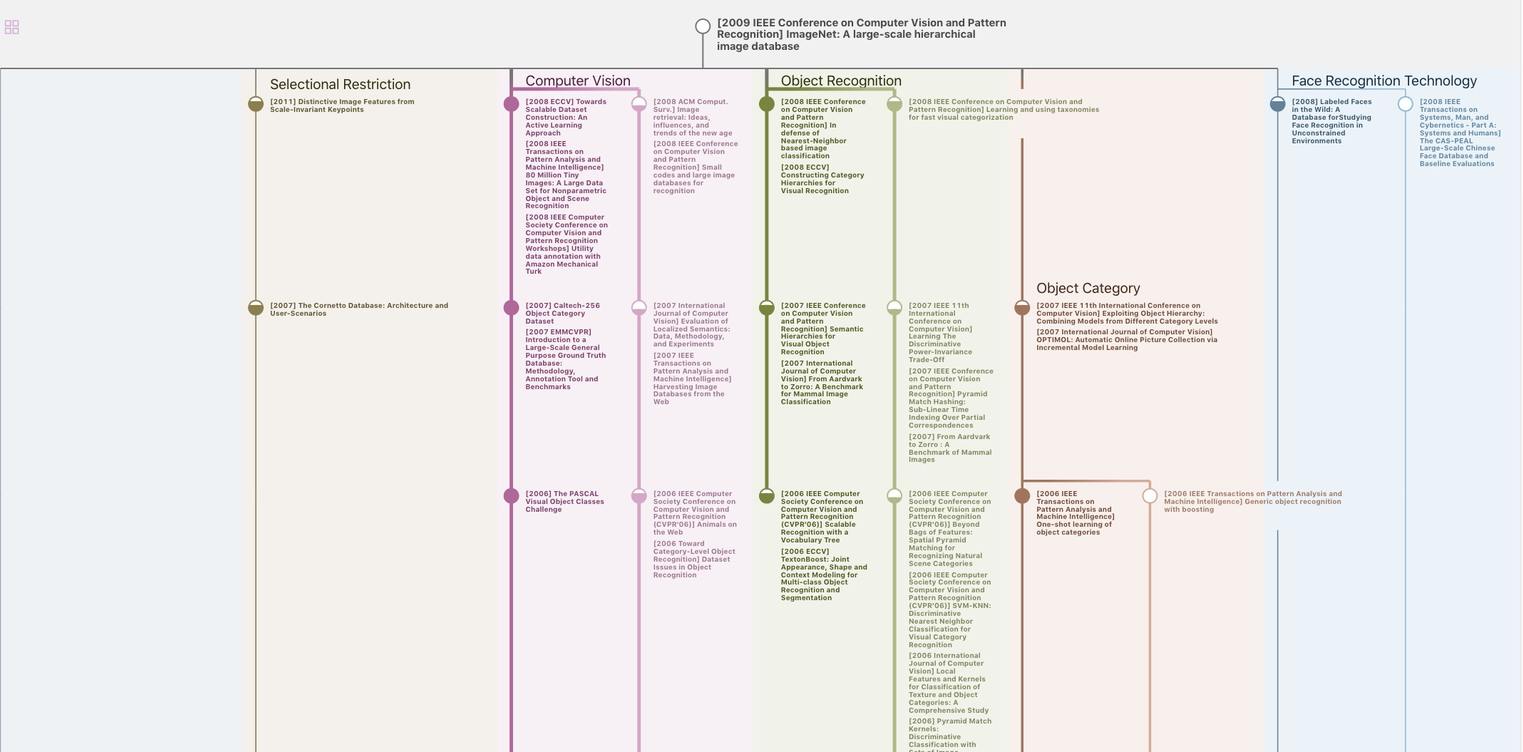
Generate MRT to find the research sequence of this paper
Chat Paper
Summary is being generated by the instructions you defined