Performance evaluation of convolution neural network models for detection of abnormal and ventricular ectopic beat cardiac episodes
Multimedia Tools and Applications(2024)
摘要
The fast and accurate detection of abnormal cardiac episodes is essential for quick diagnosis high-risk patients prone to irregular cardiac arrhythmias. Although various algorithms have been proposed in the literature, there is a need to develop artificial intelligence driven computer aided intelligent models with high detection rate. This specific study focuses on the application of Convolution Neural Network (CNN) approach to classify abnormal and ventricular ectopic beat (VEB) episodes from normal ECG signals. A cross-database approach comprised of open source and a proprietary database has been deployed for training–testing strategies. Single-channel ECG time series was converted into a derivative image stack before applying it to CNN. The current study has proposed extracting derivative image features using a pretrained network and classification using the SVM classifier. The proposed study was tested on 18 pretrained networks such as Resnet18, Resnet50, Resnet101, Densnet201, InceptionV3, Googlenet, Squeeznet, Vgg19, Vgg16, Alexnet, InceptionResnetV2, Shufflenet, MobilenetV2, NASnet-Mobile, Darknet-19, Draknet-53, Xception, and NASnet-Large for optimization. Among the chosen pretrained models, Mobilenetv2 has shown the highest classification accuracy of 92.73% & 99.29%, with relative performance of 1.24 & 0.95 for the normal-abnormal and normal-VEB cardiac episode classifications of opensource datasets. The highest accuracy of 73.66% (using xception) and 99.92% (using Inceptionv3) was achieved for abnormal and VEB cardiac episodes detection from proprietary datasets. In addition, the proposed method showed the highest classification accuracy of 90.14% with Inceptionv3 and 99.34% with darknet19 using the proprietary database for normal-abnormal & N-V cardiac episode classification. Further, inceptionv3 & darknet19 trained models using the proprietary database showed the highest detection rate of 81.67% & 94.66% for abnormal & ventricular ectopic beat cardiac episodes of the open-source database. The study has concluded that the abnormal and VEB cardiac episode detection using CNN-based models could help diagnose and treat cardiac patients.
更多查看译文
关键词
Electrocardiogram (ECG),Abnormal episodes,Ventricular ectopic beat (VEB) episodes,Convolution neural network (CNN),Derivative images
AI 理解论文
溯源树
样例
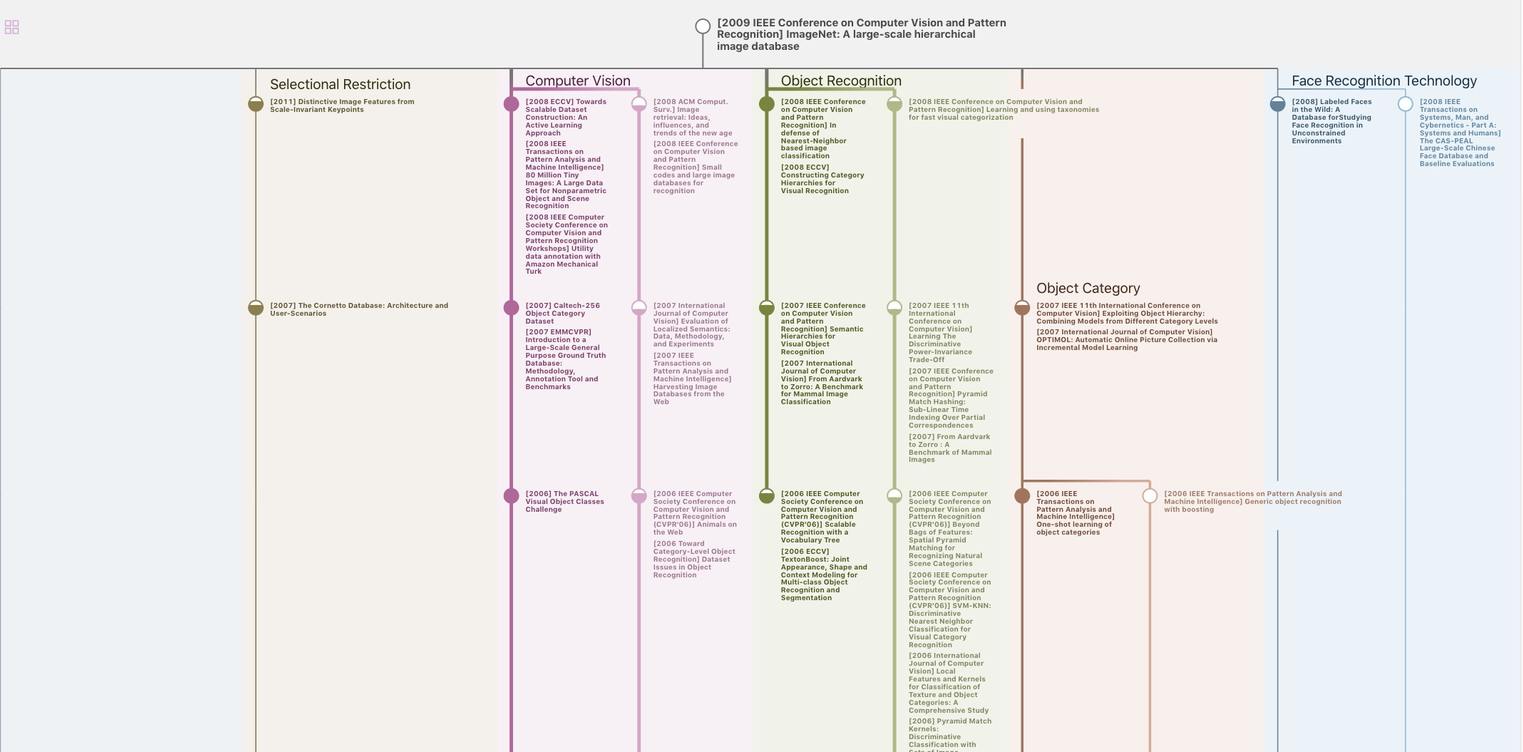
生成溯源树,研究论文发展脉络
Chat Paper
正在生成论文摘要