Machine learning guided rapid discovery of narrow-bandgap inorganic halide perovskite materials
Applied Physics A(2024)
摘要
The bandgap of inorganic halide perovskites plays a crucial role in the efficiency of solar cells. Although density functional theory can be used to calculate the bandgap of materials, the method is time-consuming and requires deep knowledge of theoretical calculations, theoretical calculations are frequently constrained by complex electronic correlations and lattice dynamics, resulting in discrepancies between calculated and experimental results. To address this issue, this study employs machine learning to predict the bandgap of inorganic halide perovskites. The XGBoost classifier classifies ABX 3 -type inorganic halide perovskites into narrow and wide bandgap materials. The study collected a dataset consisting of 447 perovskites and generated material descriptors using the Matminer Python package. The model predicts narrow-bandgap materials with 95% accuracy. Finally, the Shapley analysis revealed that the key factor affecting the bandgap of perovskites is the electronegativity range. As the range of electronegativity increases, so does the possibility of a perovskite with a narrow bandgap. These findings highlight the powerful ability of machine learning to quickly and accurately predict the bandgap of perovskites.
更多查看译文
关键词
Inorganic halide perovskites,Bandgap design,Machine learning,Feature engineering
AI 理解论文
溯源树
样例
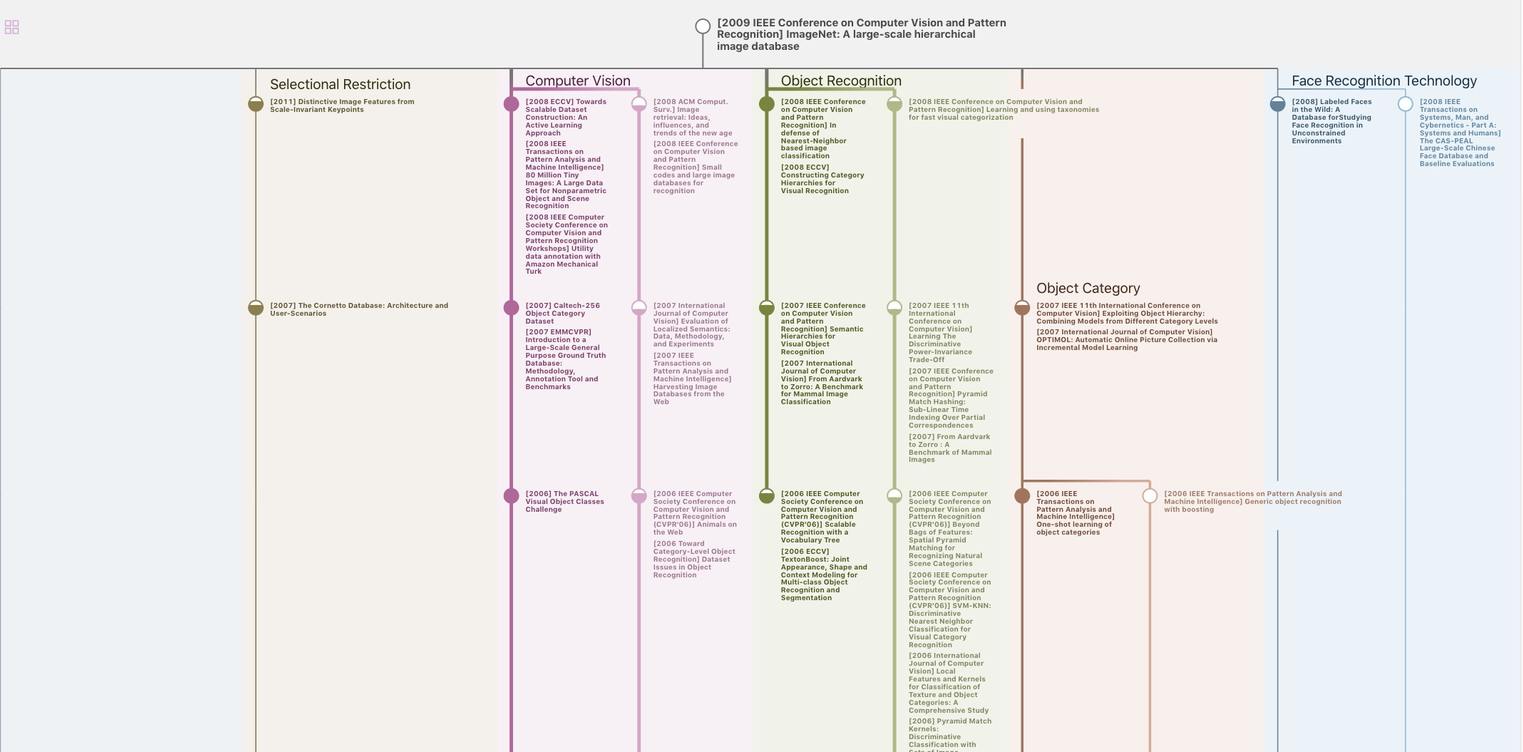
生成溯源树,研究论文发展脉络
Chat Paper
正在生成论文摘要