VW-PINNs: A Volume Weighting Method for PDE Residuals in Physics-Informed Neural Networks
ACTA MECHANICA SINICA(2025)
关键词
Physics-informed neural networks,Partial differential equations,Nonuniform sampling,Residual balancing,Deep learning
AI 理解论文
溯源树
样例
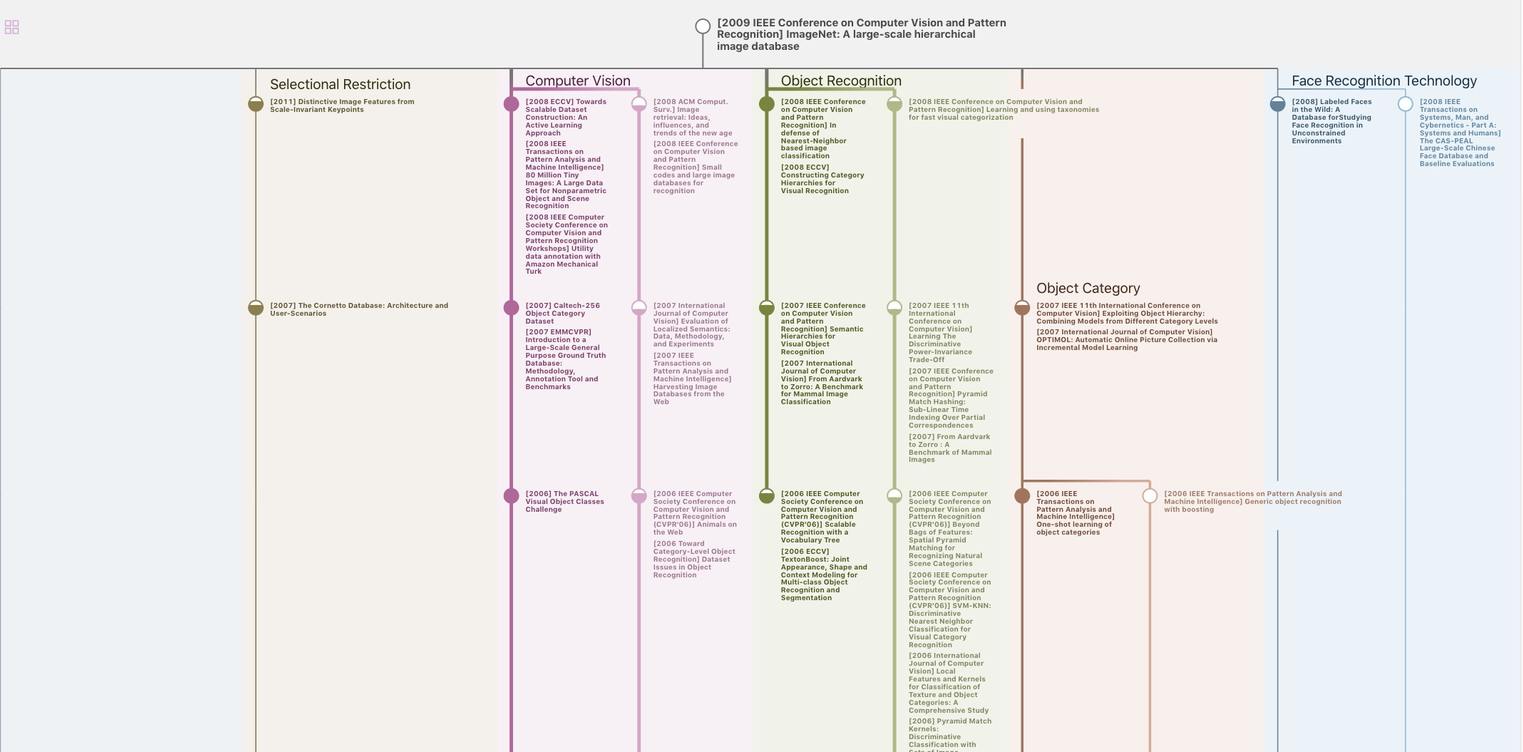
生成溯源树,研究论文发展脉络
Chat Paper
正在生成论文摘要