Editorial Commentary: Artificial Intelligence Models Using Machine Learning Can Improve Preoperative Identification of Subscapularis Pathology
ARTHROSCOPY-THE JOURNAL OF ARTHROSCOPIC AND RELATED SURGERY(2024)
Abstract
Subscapularis pathology is difficult to diagnose, in part because of decreased sensitivity and accuracy in identifying tears with magnetic resonance imaging (MRI) when compared to other cuff tendons. Artificial intelligence evaluation of patient physical examination and MRI data using a machine learning model shows that arthroscopically confirmed partial- or full -thickness subscapularis tears are highly associated with abnormal subscapularis tendon length, long head of the biceps tears, and subscapularis fatty atrophy, and on physical examination, with weakness with internal rotation and positive lift-off, belly press, and bear hug tests. Today, physicians may use machine learning as a tool, but this model may not currently be sufficient to drastically change practice. However, with continued research and development, which is occurring rapidly, similar models could aid physicians in timely identification of pathology and optimization of preoperative planning, as well as physician training and education.
MoreTranslated text
AI Read Science
Must-Reading Tree
Example
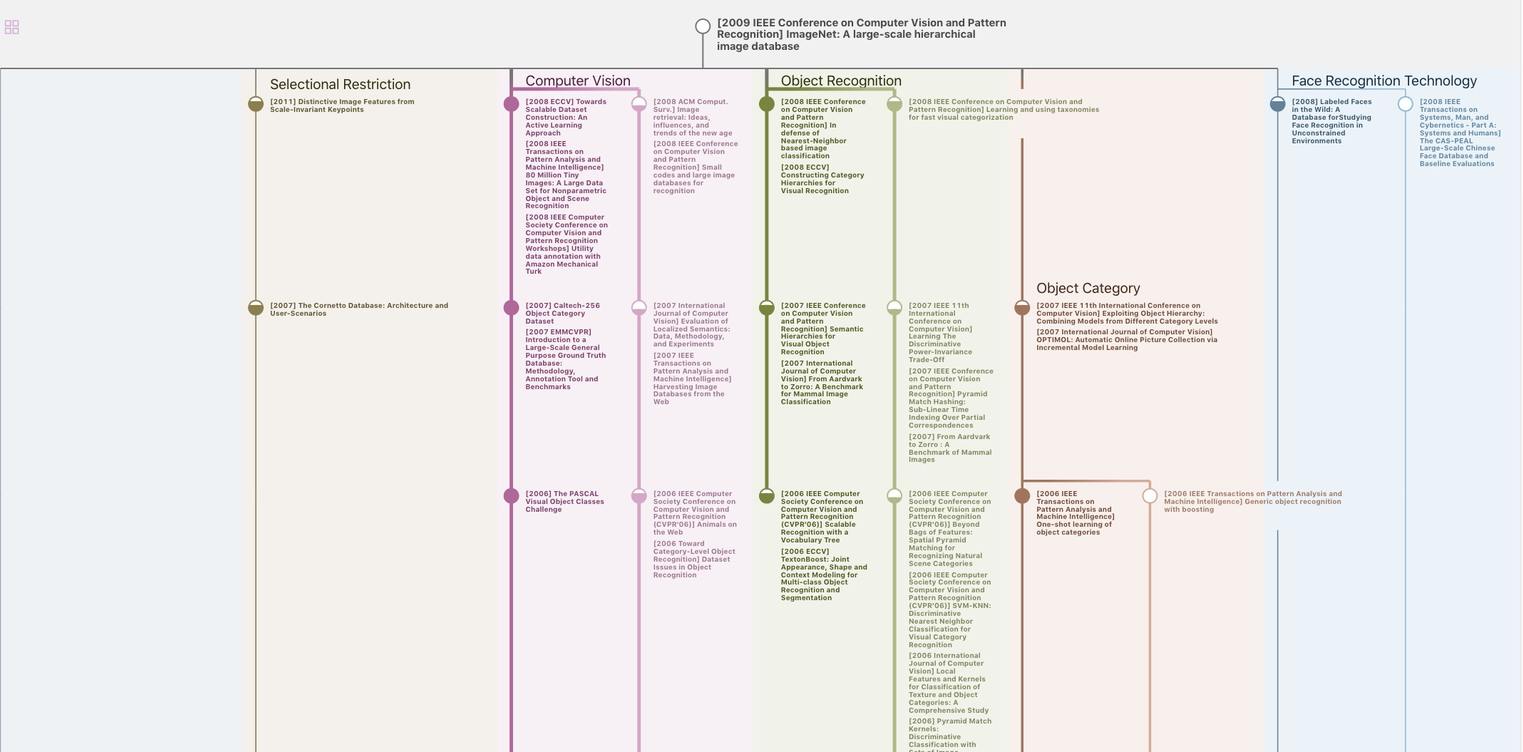
Generate MRT to find the research sequence of this paper
Chat Paper
Summary is being generated by the instructions you defined