Data-Driven Battery Characterization and Prognosis: Recent Progress, Challenges, and Prospects
SMALL METHODS(2024)
摘要
Battery characterization and prognosis are essential for analyzing underlying electrochemical mechanisms and ensuring safe operation, especially with the assistance of superior data-driven artificial intelligence systems. This review provides a unique perspective on recent progress in data-driven battery characterization and prognosis methods. First, recent informative image characterization and impedance spectrum as well as high-throughput screening approaches on revealing battery electrochemical mechanisms at multiple scales are summarized. Thereafter, battery prognosis tasks and strategies are described, with the comparison of various physics-informed modeling strategies. Considering unlocking mechanisms from tremendous battery data, the dominant role of physics-informed interpretable learning in accelerating energy device development is presented. Finally, challenges and prospects on data-driven characterization and prognosis are discussed toward accelerating energy device development with much-enhanced electrochemical transparency and generalization. This review is hoped to supply new ideas and inspirations to the next-generation battery development. The data-driven characterization and prognosis methods for batteries, including multiscale informative characterization and physics-informed machine learning developed in recent years, are reviewed in this article. This review proposes promising research directions of multimodal fusion and unified modeling for accelerating next-generation battery development.image
更多查看译文
关键词
battery characterization,battery prognosis,data-driven methods,explainable artificial intelligence,physics-informed learning
AI 理解论文
溯源树
样例
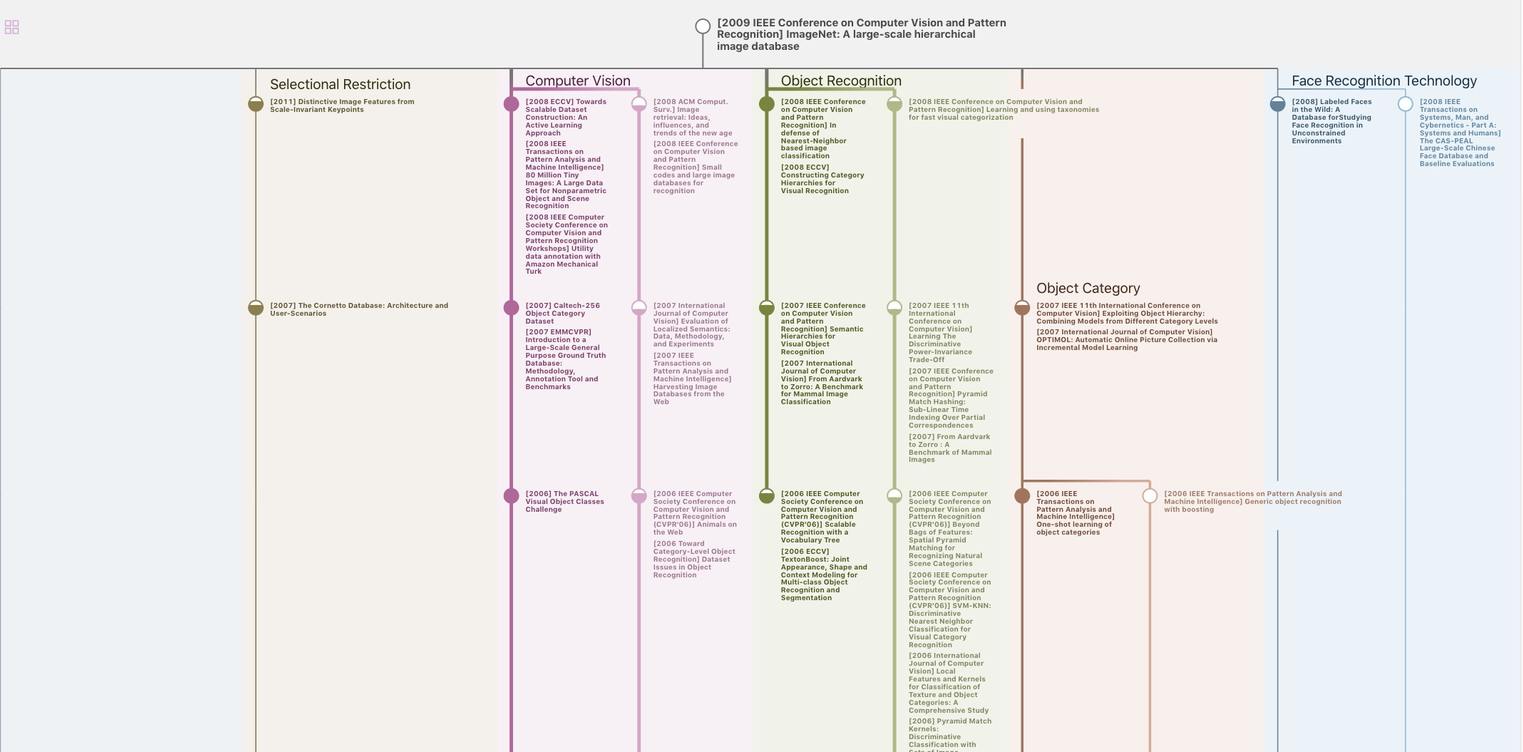
生成溯源树,研究论文发展脉络
Chat Paper
正在生成论文摘要