CorrDiff: Corrective Diffusion Model for Accurate MRI Brain Tumor Segmentation
IEEE JOURNAL OF BIOMEDICAL AND HEALTH INFORMATICS(2024)
摘要
Accurate segmentation of brain tumors in MRI images is imperative for precise clinical diagnosis and treatment. However, existing medical image segmentation methods exhibit errors, which can be categorized into two types: random errors and systematic errors. Random errors, arising from various unpredictable effects, pose challenges in terms of detection and correction. Conversely, systematic errors, attributable to systematic effects, can be effectively addressed through machine learning techniques. In this paper, we propose a corrective diffusion model for accurate MRI brain tumor segmentation by correcting systematic errors. This marks the first application of the diffusion model for correcting systematic segmentation errors. Additionally, we introduce the Vector Quantized Variational Autoencoder (VQ-VAE) to compress the original data into a discrete coding codebook. This not only reduces the dimensionality of the training data but also enhances the stability of the correction diffusion model. Furthermore, we propose the Multi-Fusion Attention Mechanism, which can effectively enhances the segmentation performance of brain tumor images, and enhance the flexibility and reliability of the corrective diffusion model. Our model is evaluated on the BRATS2019, BRATS2020, and Jun Cheng datasets. Experimental results demonstrate the effectiveness of our model over state-of-the-art methods in brain tumor segmentation.
更多查看译文
关键词
Diffusion Model,Brain Tumor Segmentation,Corrective Learning
AI 理解论文
溯源树
样例
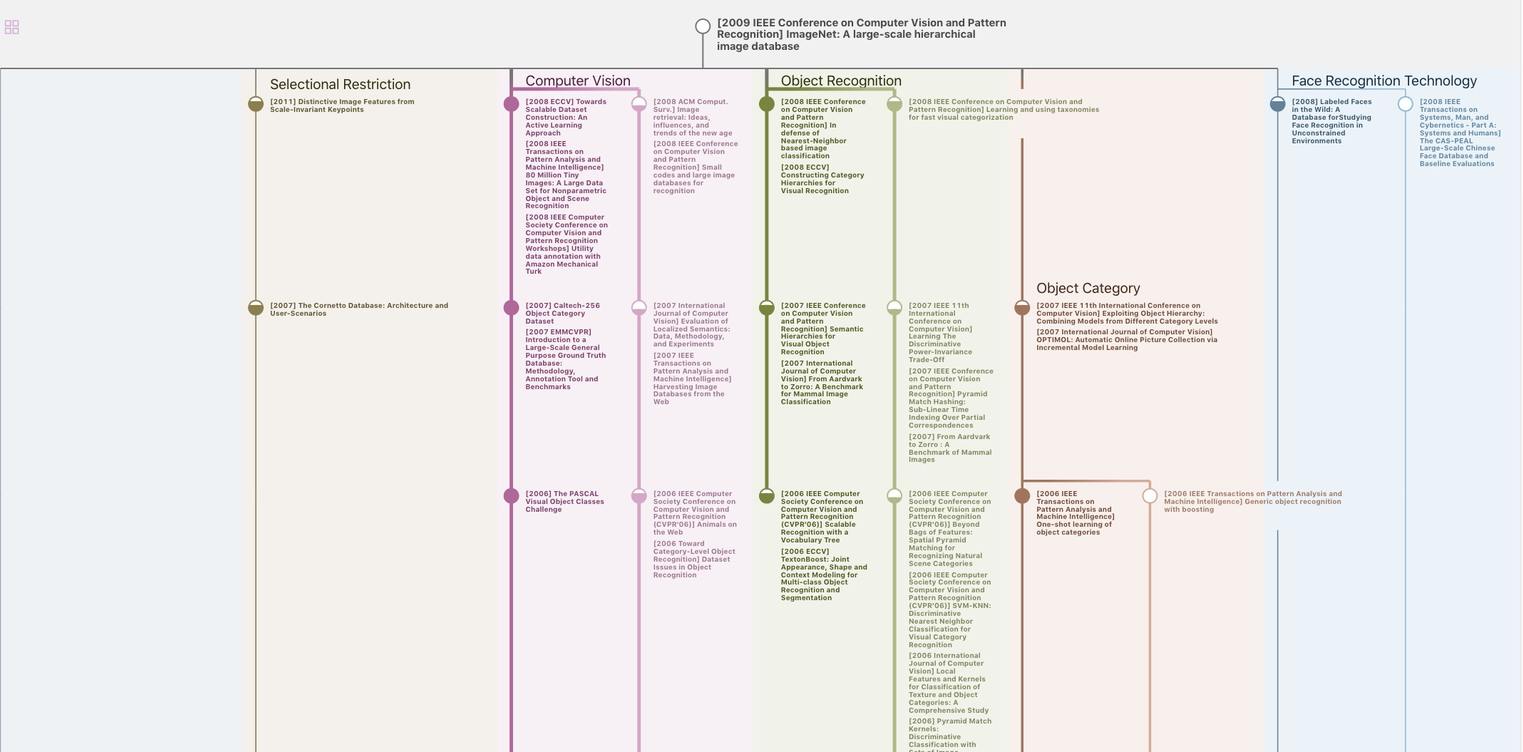
生成溯源树,研究论文发展脉络
Chat Paper
正在生成论文摘要