ZSL-SLCNN: Zero-Shot Learning with Semantic Label CNN for Malware Classification.
International Conference on Control, Automation and Information Sciences(2023)
摘要
Malware is becoming increasingly sophisticated, with new malware constantly emerging, posing challenges for cybersecurity teams to classify them accurately. Traditional supervised learning methods have achieved impressive results recently, thanks to the contributions of various novel techniques and models. However, a notable drawback of this approach is its inability to classify malicious code it has never encountered, in other words, with untrained labels. Zero-shot Learning has been explored in diverse fields to address this limitation, but its performance still needs to improve. Notably, most current studies only focus on one-layer mapping, with few outstanding achievements, and studies using zero-shot learning in malware classification need to be improved. In this paper, we propose a novel method utilizing Semantic Labels with two-layer mapping to enhance the efficiency of traditional Zero-Shot Learning. This study explores how zero-shot learning can be leveraged to detect image-based malware using merely simple CNN and pre-trained word embedding as semantic labels. Through experimental results, we demonstrate the effectiveness of our proposed method for classifying both seen and unseen malware families.
更多查看译文
关键词
Malware Classification,Semantic Label,CNN,Zero-shot Learning,Information Security
AI 理解论文
溯源树
样例
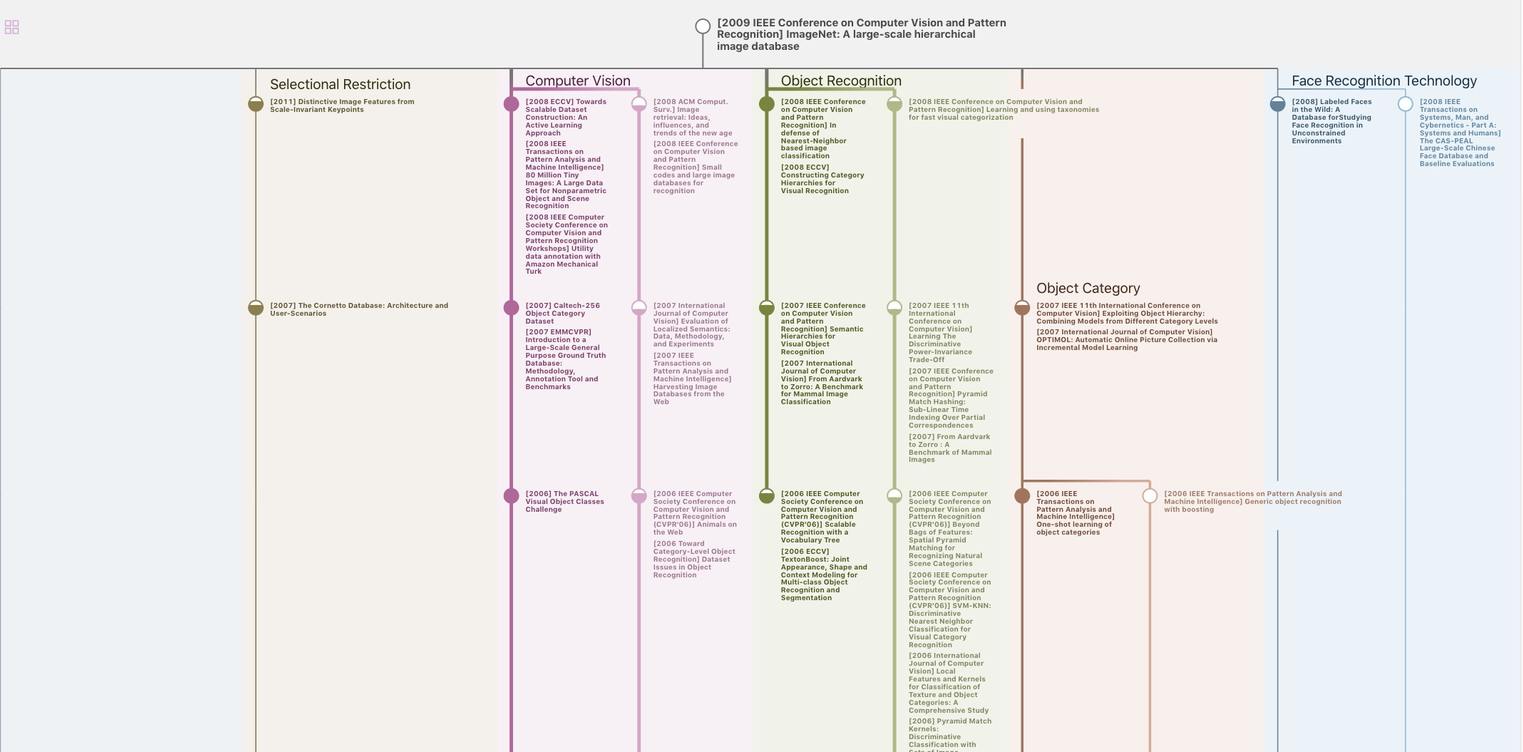
生成溯源树,研究论文发展脉络
Chat Paper
正在生成论文摘要