Improving Land Cover Segmentation Using Multispectral Dataset
2023 Eighth International Conference on Informatics and Computing (ICIC)(2023)
摘要
Computer vision has been used in many areas such as medical, transportation, military, geography, etc. The fast development of sensor devices inside camera and satellite provides not only red-greed-blue (RGB) images but also multispectral dataset with some channels including RGB, infrared, short-wave, and thermal wave. Most of the dataset is panchromatic (black and white) and RGB, for example Google Map and other satellite-based map applications. This study examines the effects of multispectral dataset for semantic segmentation of land cover. The comparison between RGB with band 2 to band 7 of Landsat 8 Satellite shows an improvement of accuracy from 90.283 to 94.473 for U-Net and from 91.76 to 95.183 for DeepLabV3+. In addition, this research also compares two well-known semantic segmentation methods, namely U-Net and DeepLabV3+, that shown that DeepLabV3+ outperformed U-Net regarding to speed and accuracy. Testing was conducted in the Karawang Regency area, West Java, Indonesia.
更多查看译文
关键词
DeepLabV3+,Semantic Segmentation,Landsat,MATLAB,Deep Learning
AI 理解论文
溯源树
样例
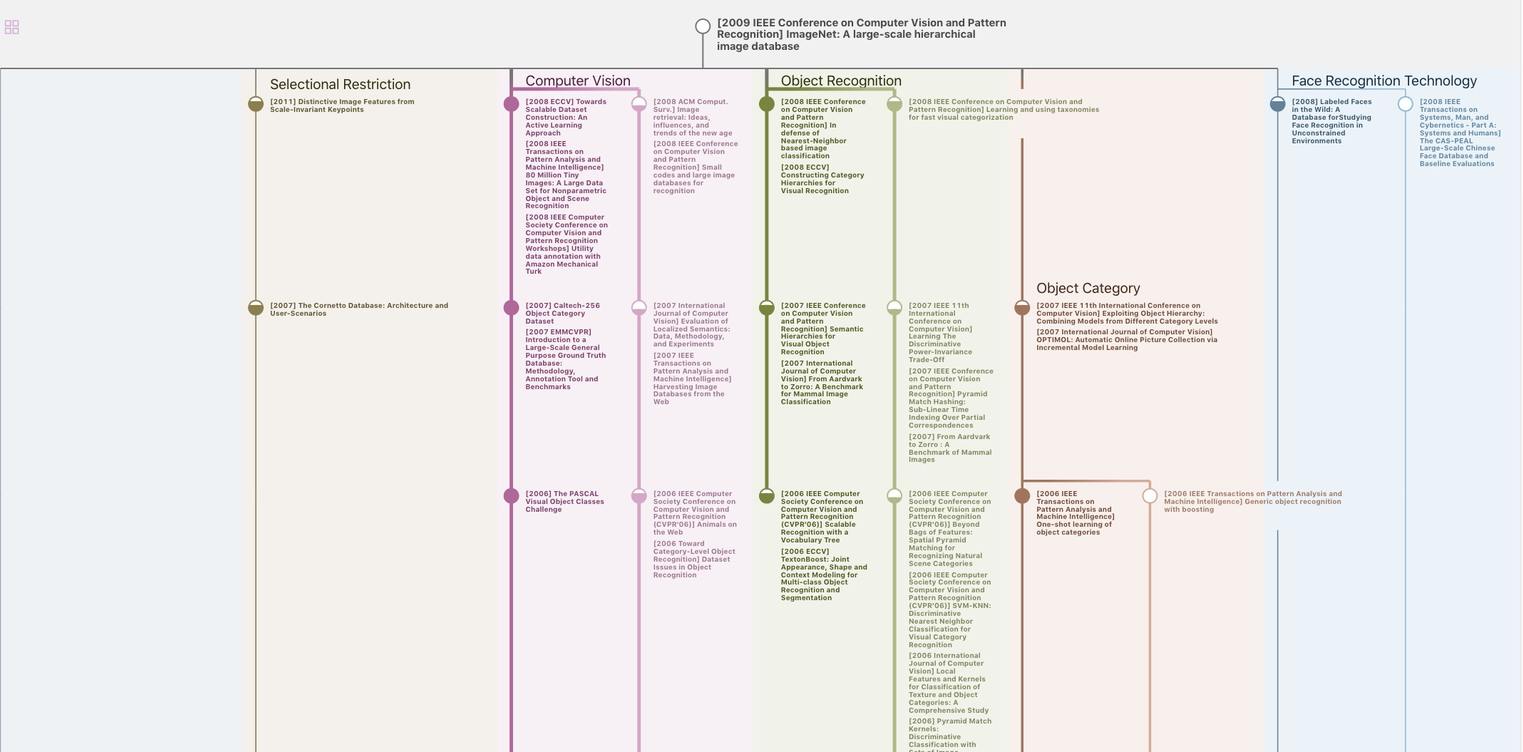
生成溯源树,研究论文发展脉络
Chat Paper
正在生成论文摘要