A Deep Learning and Image Processing Pipeline for Object Characterization in Firm Operations
INFORMS JOURNAL ON COMPUTING(2023)
摘要
Given the abundance of images related to operations that are being captured and stored, it behooves firms to innovate systems using image processing to improve operational performance that refers to any activity that can save labor cost. In this paper, we use deep learning techniques, combined with classic image/signal processing methods, to propose a pipeline to solve certain types of object counting and layer characterization problems in firm operations. Using data obtained by us through a collaborative effort with real manufacturers, we demonstrate that the proposed pipeline method is able to achieve higher than 93% accuracy in layer and log counting. Theoretically, our study conceives, constructs, and evaluates proof of concept of a novel pipeline method in characterizing and quantifying the number of defined items with images, which overcomes the limitations of methods based only on deep learning or signal processing. Practically, our proposed method can help firms significantly reduce labor costs and/or improve quality and inventory control by recording the number of products in real time, more accurately and with minimal up-front technological investment. The codes and data are made publicly available online through the INFORMS Journal on Computing GitHub site.
更多查看译文
关键词
image processing,layer and object counting,machine learning,operational efficiency
AI 理解论文
溯源树
样例
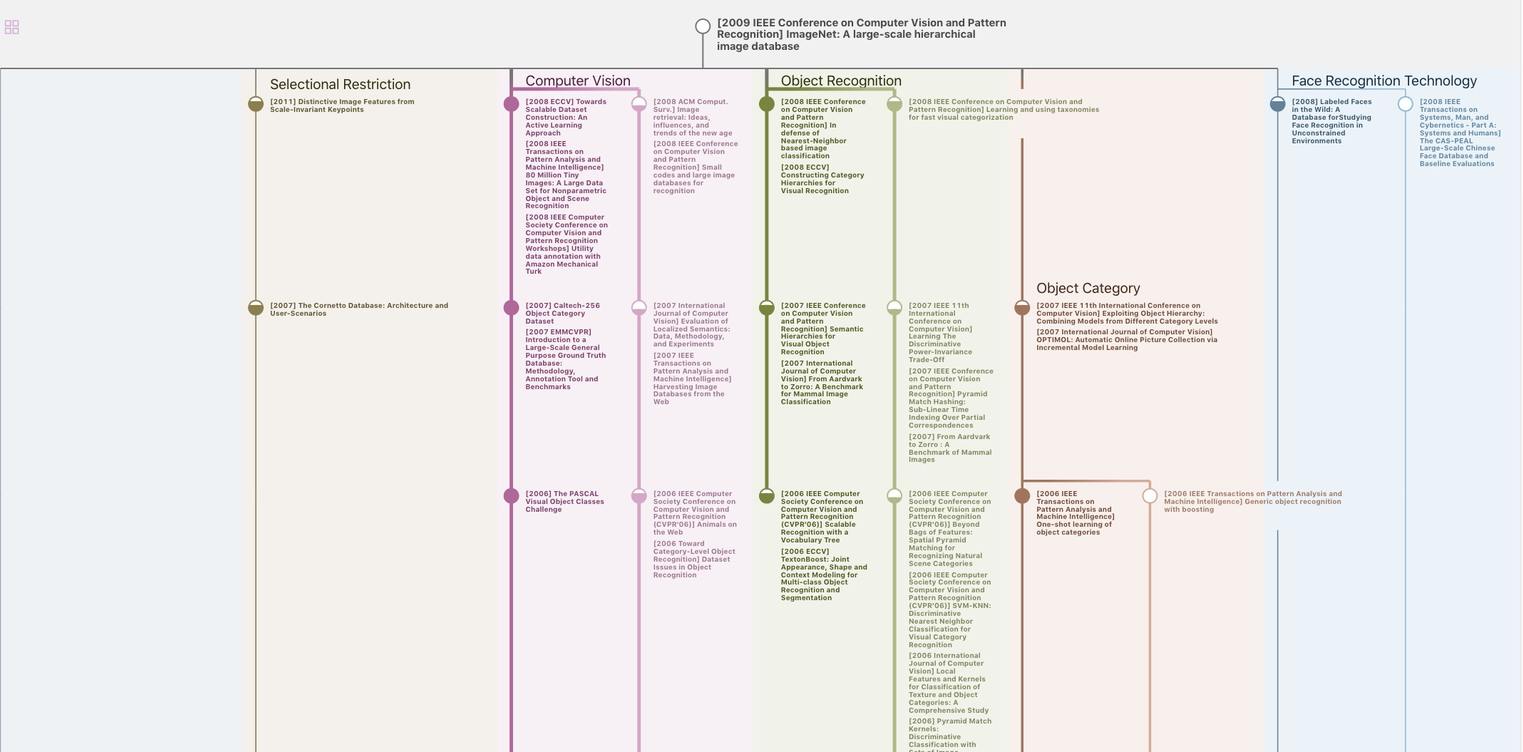
生成溯源树,研究论文发展脉络
Chat Paper
正在生成论文摘要