Multiple Imputation of Missing Data with Skip-Pattern Covariates: a Comparison of Alternative Strategies
JOURNAL OF STATISTICAL COMPUTATION AND SIMULATION(2024)
Key words
Multiple imputation,missing skip-pattern variables,RANDS survey
AI Read Science
Must-Reading Tree
Example
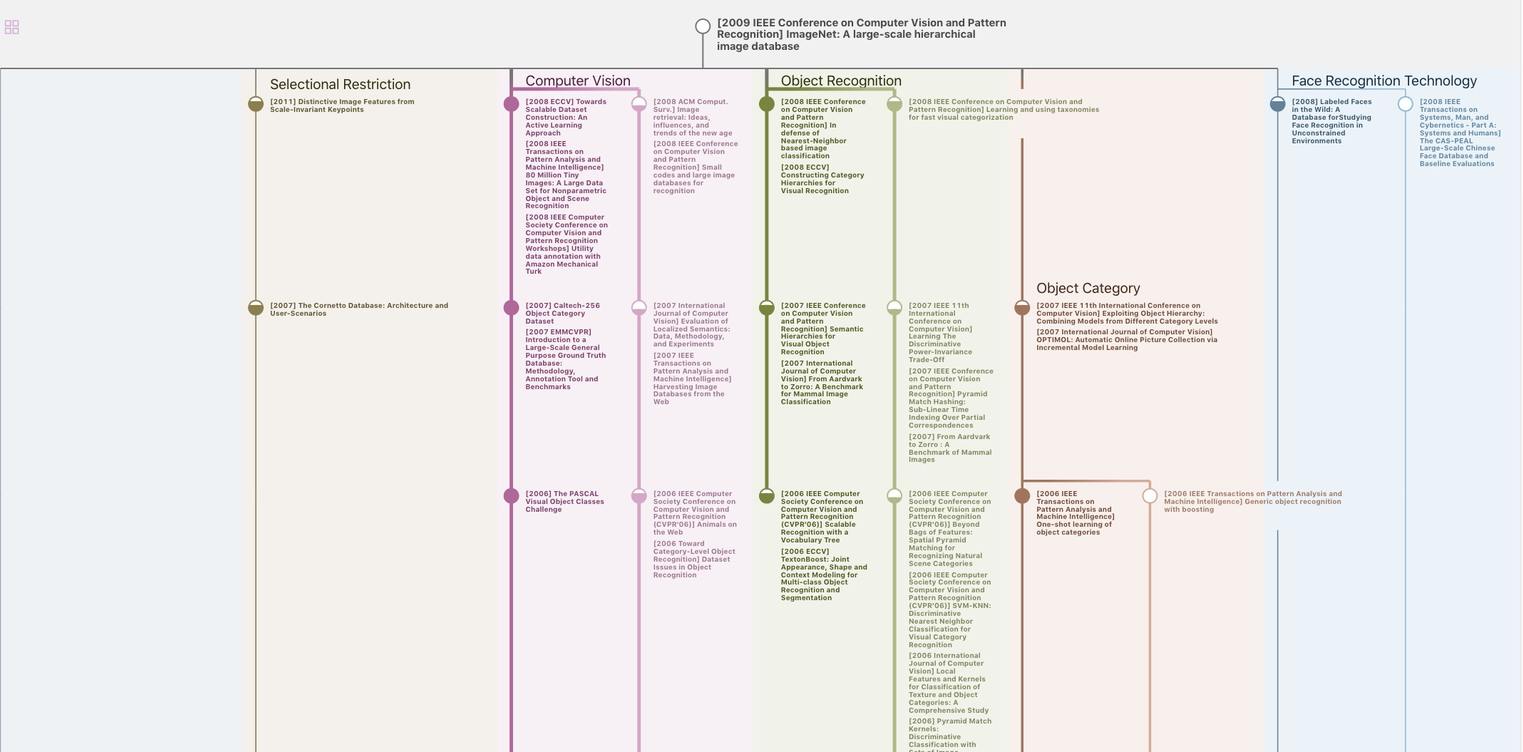
Generate MRT to find the research sequence of this paper
Chat Paper
Summary is being generated by the instructions you defined