I3: Interactive Iterative Improvement for Few-Shot Action Segmentation
2023 32ND IEEE INTERNATIONAL CONFERENCE ON ROBOT AND HUMAN INTERACTIVE COMMUNICATION, RO-MAN(2023)
Abstract
Extracting modular segments from raw video demonstrations of high-level actions is important to understand the underlying building blocks for different tasks in humanrobot interaction. While (data-hungry) supervised learning approaches for Action Segmentation show good performance when the underlying segments are predefined, their performance degrades when unseen actions are introduced on-thego as new data samples are scarce. In this regard, Zero- and Few-Shot Learning approaches have shown good performance in generalizing to unseen examples. In Action Segmentation, where each frame needs to be labeled, annotating new data even for a few tasks can become tedious as the number of tasks scale. In this work, we propose Interactive Iterative Improvement (I 3) for Few-Shot Action Segmentation, a Semi-Supervised Interactive Meta-Learning approach for Zero-Shot Learning on unlabeled videos and Few-Shot Learning on small amounts of labeled videos. I 3 consists of a Prototypical Network model for frame-wise prediction coupled with a Hidden-Semi-Markov-Model to prevent over-segmentation. The model is iteratively improved in an interactive manner through users' annotations provided via a webinterface. This is done in a taskagnostic manner that, in theory, can be reused for a number of different actions. Our model provides sequentially accurate segmentations using only a limited amount of labeled data which shows the efficacy of our learning approach. A lower edit distance compared to baselines indicates a lower number of required user edits making it well suited for non-expert users to smoothly provide annotations enabling them to have more control over the learned model.
MoreTranslated text
Key words
Interactive Learning,Action Segmentation,Few-Shot Learning,Human-In-The-Loop
AI Read Science
Must-Reading Tree
Example
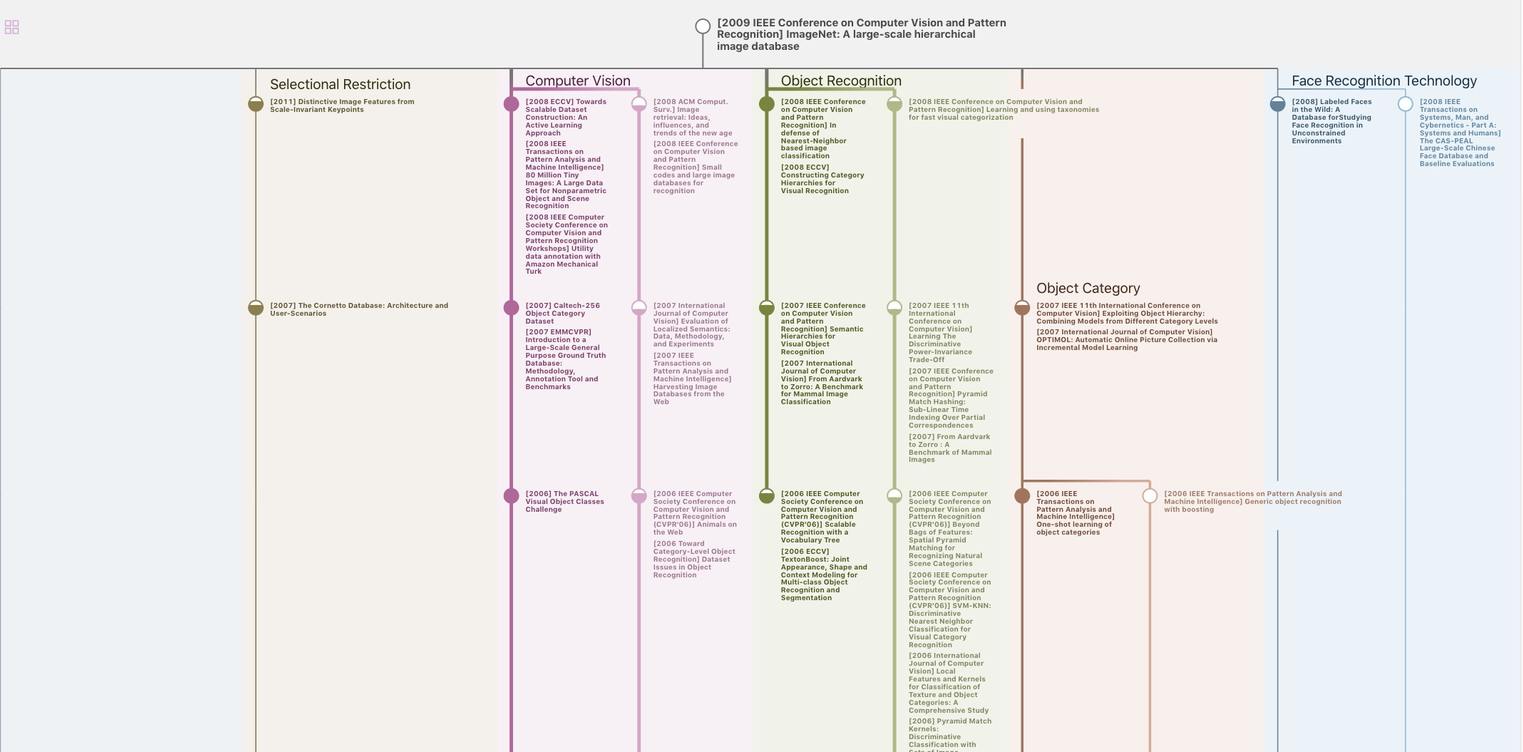
Generate MRT to find the research sequence of this paper
Chat Paper
Summary is being generated by the instructions you defined