Machine learning-based approach for maritime target classification and anomaly detection using millimetre wave radar Doppler signatures
IET RADAR SONAR AND NAVIGATION(2024)
摘要
The authors present multiple machine learning-based methods for distinguishing maritime targets from sea clutter. The main goal for this classification framework is to aid future millimetre wave radar system design for marine autonomy. Availability of empirical data at this frequency range in the literature is scarce. The classification and anomaly detection techniques reported here use experimental data collected from three different field trials from three different millimetre wave radars. Two W-band radars operating at 77 and 94 GHz and a G-band radar operating at 207 GHz were used for the field trial data collection. The dataset encompasses eight classes including sea clutter returns. The other targets are boat, stand up paddleboard/kayak, swimmer, buoy, pallet, stationary solid object (i.e. rock) and sea lion. The Doppler signatures of the targets have been investigated to generate feature values. Five feature values have been extracted from Doppler spectra and four feature values from Doppler spectrograms. The features were trained on a supervised learning model for classification as well as an unsupervised model for anomaly detection. The supervised learning was performed for both multi-class and 2-class (sea clutter and target) classification. The classification based on spectrum features provided an 84.3% and 80.1% validation and test accuracy respectively for the multi-class classification. For the spectrogram feature-based learning, the validation and test accuracy for multi-class increased to 93.3% and 88.7% respectively. For the 2-class classification, the spectrum feature-based training accuracies are 88.1% and 86.8%, whereas with the spectrogram feature-based model, the values are 95% and 94.1% for validation and test accuracies respectively. A one class support vector machine was also applied to an unlabelled dataset for anomaly detection training, with 10% outlier data. The cross-validation accuracy has shown very good agreement with the expected anomaly detection rate. The authors present multiple machine learning-based methods for distinguishing maritime targets from sea clutter. The main goal for this Doppler signature-based classification framework is to aid future millimetre wave radar system design for marine autonomy.image
更多查看译文
关键词
Doppler measurement,feature extraction,marine navigation,marine radar,sea clutter,support vector machines
AI 理解论文
溯源树
样例
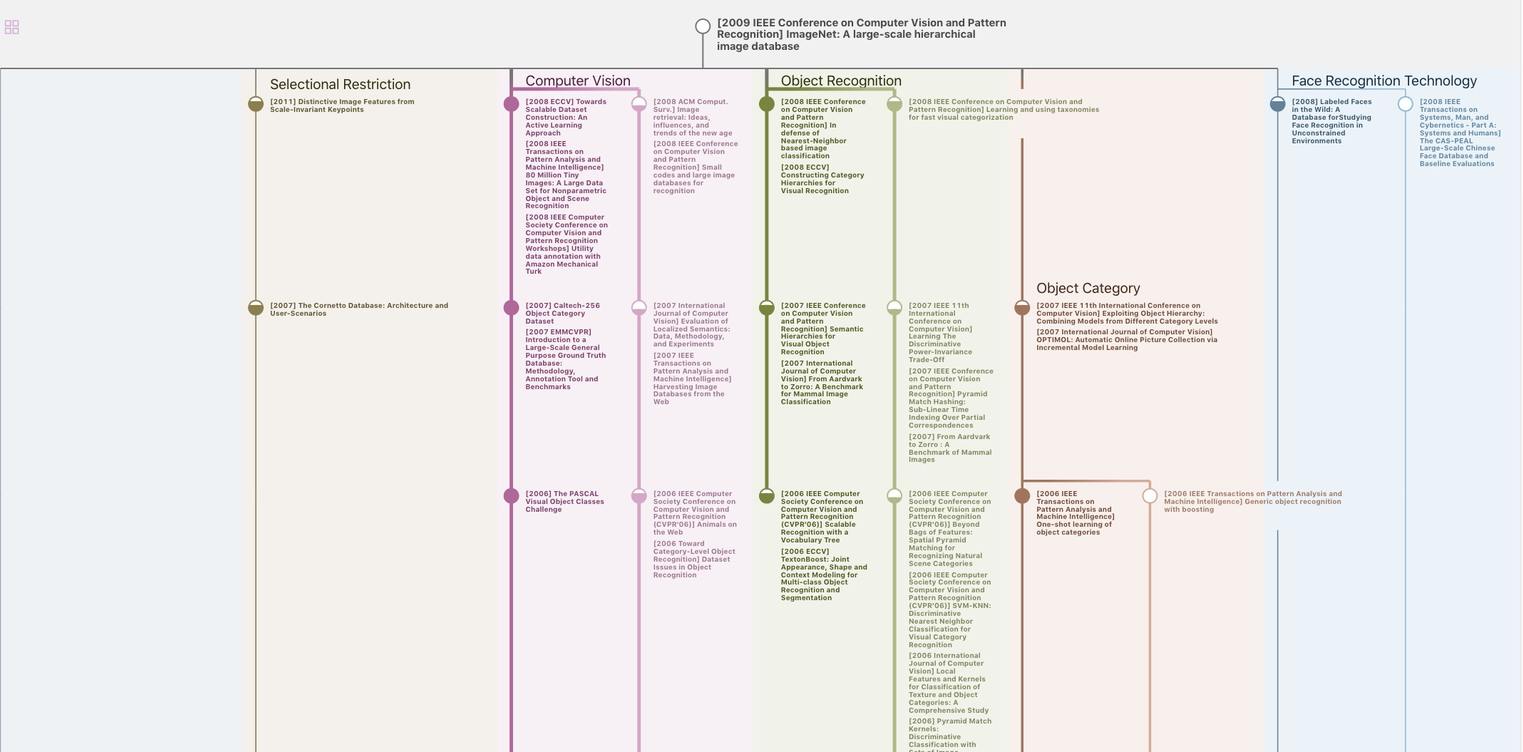
生成溯源树,研究论文发展脉络
Chat Paper
正在生成论文摘要