Performance Comparison of Deep Learning Models for Damage Identification of Aging Bridges
APPLIED SCIENCES-BASEL(2023)
摘要
Currently, damage in aging bridges is assessed visually, leading to significant personnel, time, and cost expenditures. Moreover, the results depend on the subjective judgment of the inspector. Machine-learning-based approaches, such as deep learning, can solve these problems. In particular, instance-segmentation models have been used to identify different types of bridge damage. However, the value of deep-learning-based damage identification may be reduced by insufficient training data, class imbalance, and model-reliability issues. To overcome these limitations, this study utilized photographic data from real bridge-management systems for the inspection and assessment of bridges as the training dataset. Six types of damage were considered. Moreover, the performances of three representative deep learning models-Mask R-CNN, BlendMask, and SWIN-were compared in terms of loss-function values. SWIN showed the best performance, achieving a loss value of 0.000005 after 269,939 training iterations. This shows that bridge-damage-identification performance can be maximized by setting an appropriate learning rate and using a deep learning model with a minimal loss value.
更多查看译文
关键词
deep learning,aging-bridge management,instance segmentation,damage identification,loss-functions
AI 理解论文
溯源树
样例
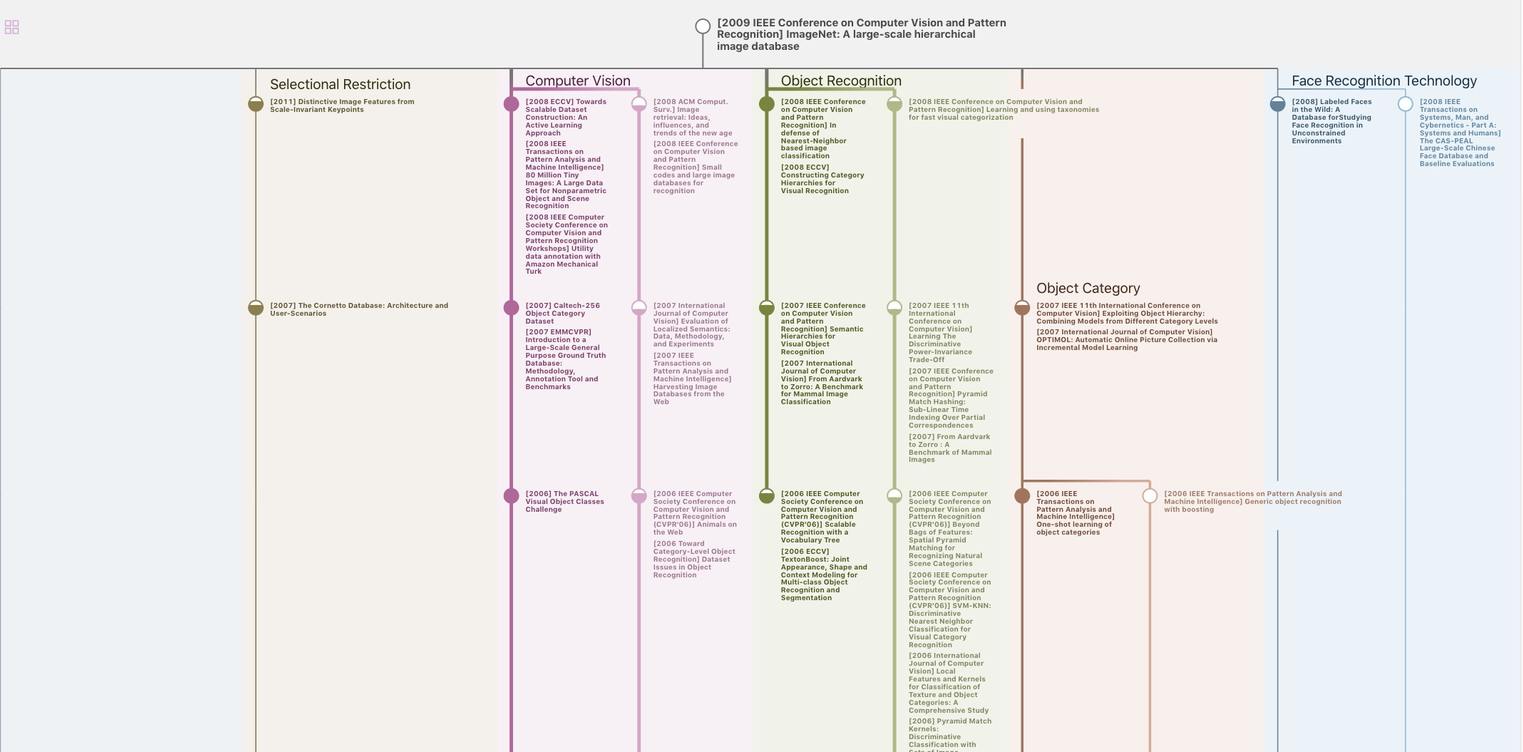
生成溯源树,研究论文发展脉络
Chat Paper
正在生成论文摘要