Comprehensive and Accurate Prediction of Band Gap for Lead-Free Double Perovskites through Self-Modified Machine Learning Strategy
JOURNAL OF PHYSICAL CHEMISTRY C(2023)
Abstract
Lead-free double perovskites attract enormous attention owing to their nontoxic, unique properties and superior stability toward various applications. Nevertheless, a strategy to accurately predict the band gap for the double perovskites remains largely undeveloped in current research for its application in the photoelectric community. Herein, a self-modified machine learning (ML) strategy is constructed with a data set containing 186 examples from the available experimental literature and density functional theory (DFT) calculations, by which six different machine learning models are employed to predict the band gap of double perovskites. Among the six ML models, XGBoost provides the best predictive performance with a root mean square error (RMSE) of 0.154 and a coefficient of determination (R-2) of 0.952. Likewise, we give an in-depth elucidation concerning the effect of the feature on the band gap by using SHapley Additive exPlanations (SHAP), which points out that the shift of VBM/CBM and electron-withdrawing ability between B and X, have the largest influence on the band gaps of the halide double perovskites. The self-modified ML strategy allows the realization of accurate prediction of the band gap for the lead-free double perovskites for optoelectrical applications.
MoreTranslated text
AI Read Science
Must-Reading Tree
Example
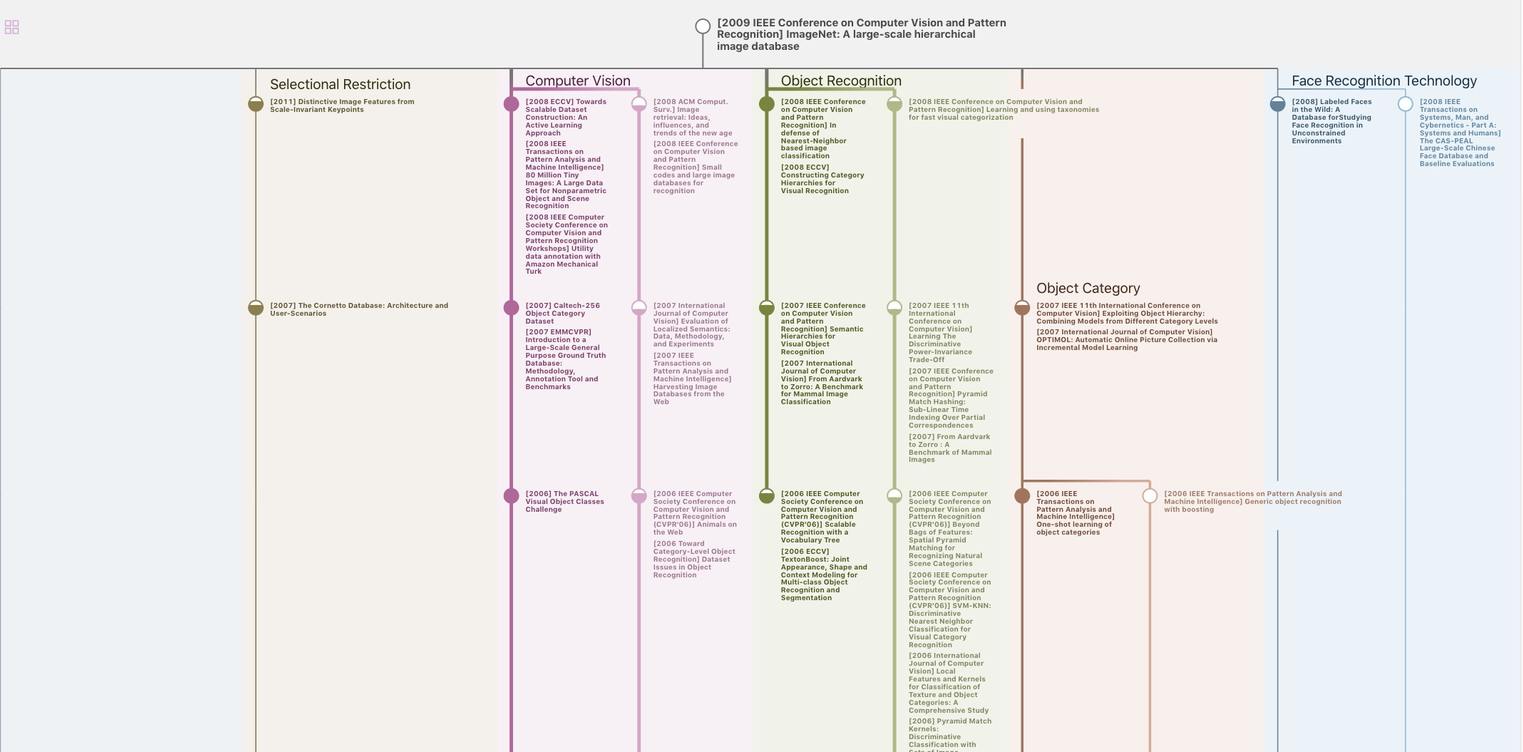
Generate MRT to find the research sequence of this paper
Chat Paper
Summary is being generated by the instructions you defined