Automatic road crack detection and analysis system based on deep feature fusion and edge structure extraction
INTERNATIONAL JOURNAL OF PAVEMENT ENGINEERING(2023)
摘要
In order to solve the problem that the traditional crack image segmentation method cannot accurately extract the edge structure of road cracks, a flexible crack detection and identification system are provided. Firstly, a road crack dataset is built by processing the acquired images using the sliding window technique. A model called R2-AENet is proposed for training and testing. The model structure uses the recurrent residual convolutional neural network (RRCNN) to improve the convolutional structure and drive the accumulation of fracture features. The model decoder combines the attention-guided filtering module and the edge residuals module (ERB) better to extract the edge features of the feature map. As a result, it improves the crack segmentation performance. Finally, the research results are validated on the upgraded software platform. The experimental results demonstrate that the R2-AENet model achieves better segmentation results compared to the popular deep learning algorithm models. Evidently, the model achieves accuracy and recall of 0.982 and 0.849 on the collected dataset. Besides, the area under the curve (AUC) metric value can reach 0.992. In addition, the detection results in different data sets perform well, verifying that the model has exceptional robustness and can be applied to various environmental road inspection and maintenance projects.
更多查看译文
关键词
Road crack,U-net,recurrent residual block,attention guided filter block,edge residual block,semantic segmentation
AI 理解论文
溯源树
样例
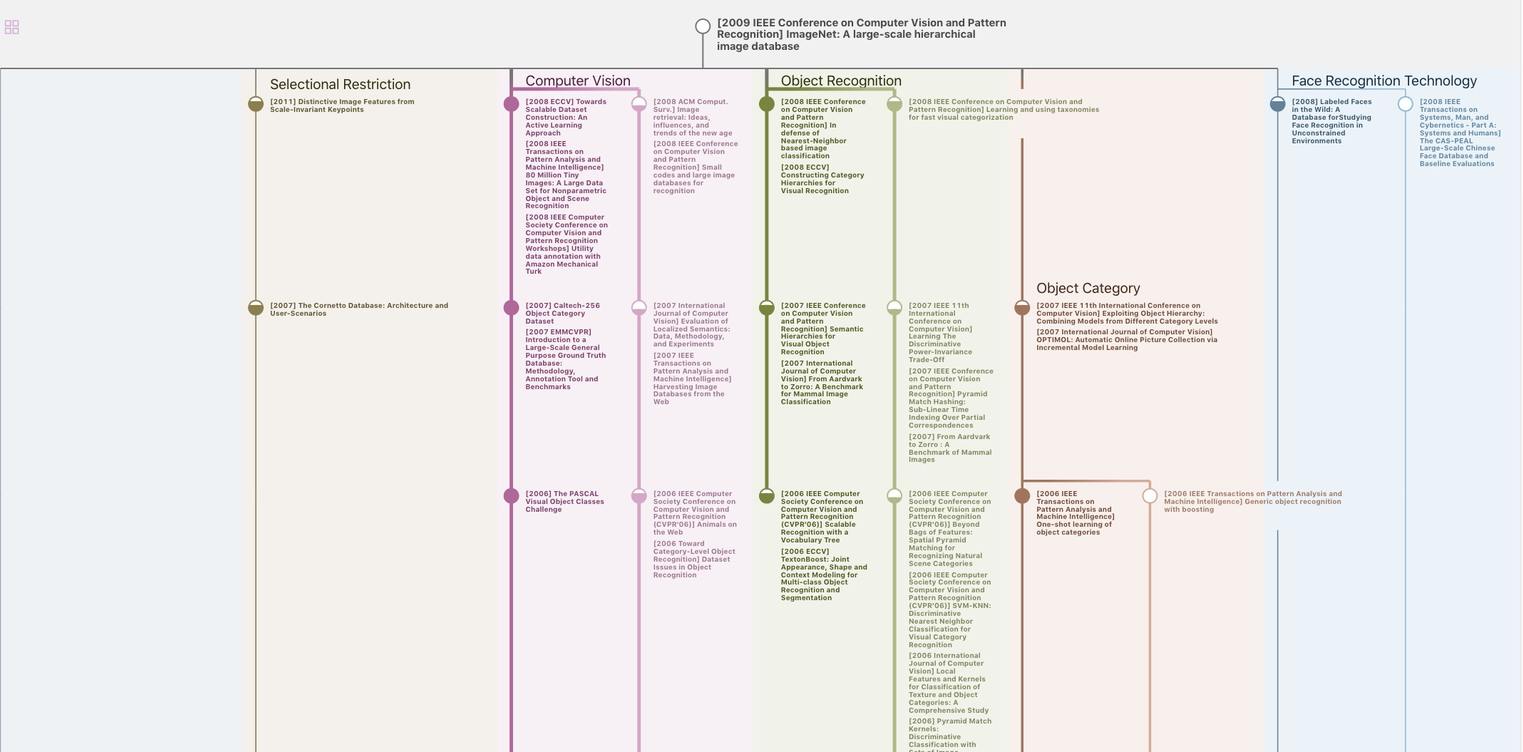
生成溯源树,研究论文发展脉络
Chat Paper
正在生成论文摘要