A 0.61-W Fully Integrated Keyword-Spotting ASIC With Real-Point Serial FFT-Based MFCC and Temporal Depthwise Separable CNN
IEEE JOURNAL OF SOLID-STATE CIRCUITS(2023)
摘要
A fully integrated near-microphone keyword spotting (KWS) chip is proposed to directly interact with a passive microphone and achieve submicrowatt power for the Internet of Things (IoT) devices. First, an on-chip analog frontend (AFE) is designed to avoid the inclusion of power-intensive off-chip active microphones. Second, a real-point serial fast Fourier transform (FFT)-based Mel-frequency cepstral coefficient (MFCC) feature extractor, cooperating with a genetic algorithm (GA) optimized bit-width quantization, is specifically customized to reduce the MFCC power by 67.4%. Finally, a binarized temporal depthwise separable CNN (TDSCN) is proposed, featuring hardware optimization through a parallel adder tree (PAT)-based PE with near-memory computing. This results in a 78.9% reduction in computation as compared to the traditional depthwise separable convolutional neural networks (CNNs). Fabricated in a 28-nm CMOS process, the proposed KWS chip consumes the lowest power of 0.61 mu W at 0.36-V neural network (NN), 0.9-V AFE, and 8-KHz frequency, while keeping 95.8% accuracy for two-KWS on Google speech command dataset (GSCD).
更多查看译文
关键词
Keyword spotting (KWS),Mel frequency cepstral coefficients (MFCC),near-threshold voltage (NTV) design,neural network (NN) accelerator,real-point serial fast Fourier transform (FFT)
AI 理解论文
溯源树
样例
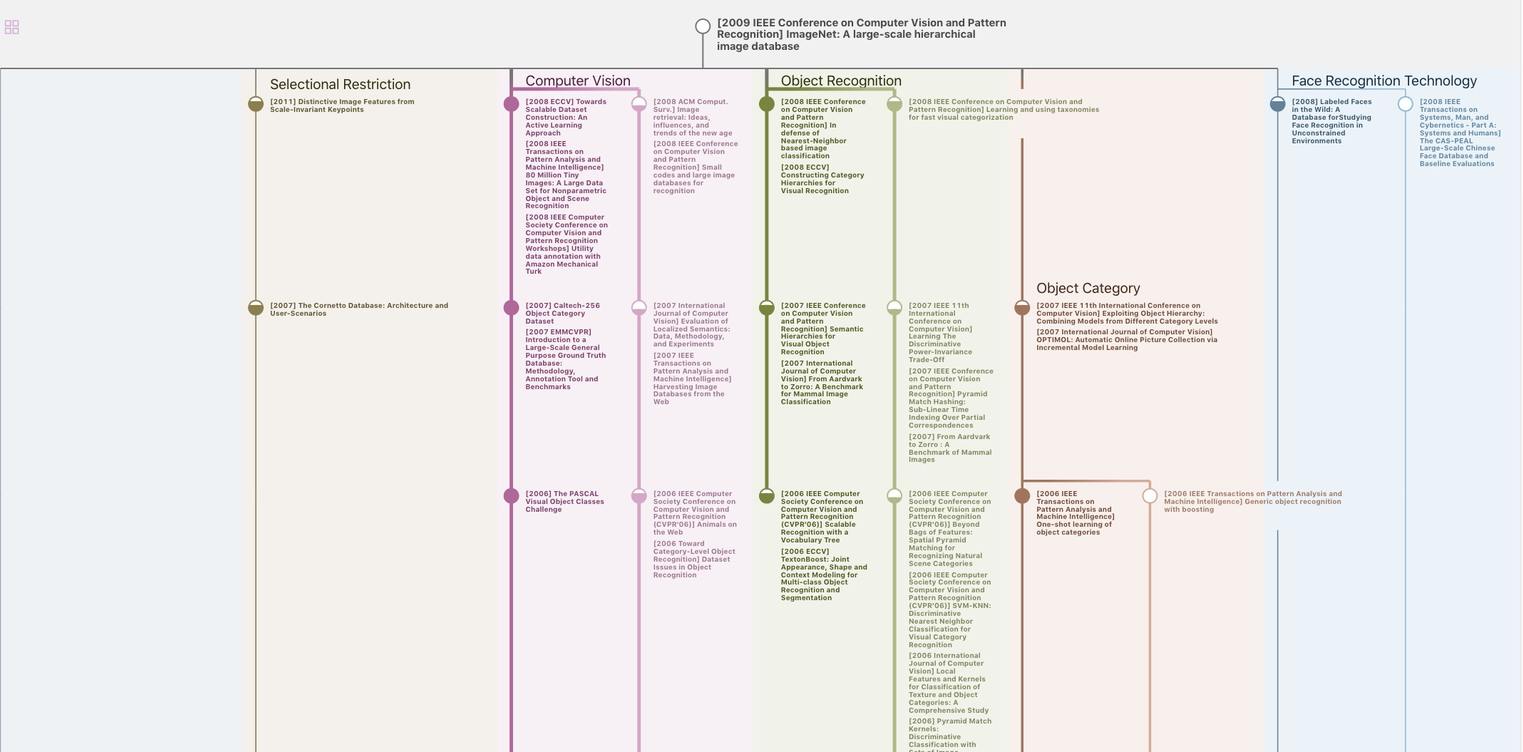
生成溯源树,研究论文发展脉络
Chat Paper
正在生成论文摘要