Erratum: Risk Bounds for the Majority Vote: From a PAC-Bayesian Analysis to a Learning Algorithm
JOURNAL OF MACHINE LEARNING RESEARCH(2023)
摘要
This work shows that the demonstration of Proposition 15 of Germain et al. (2015) is flawed and the proposition is false in a general setting. This proposition gave an inequal-ity that upper-bounds the variance of the margin of a weighted majority vote classifier. Even though this flaw has little impact on the validity of the other results presented in Germain et al. (2015), correcting it leads to a deeper understanding of the C-bound, which is a key inequality that upper-bounds the risk of a majority vote classifier by the moments of its margin, and to a new result, namely a lower-bound on the C-bound. Notably, Ger-main et al.'s statement that "the C-bound can be arbitrarily small" is invalid in presence of irreducible error in learning problems with label noise. In this erratum, we pinpoint the mistake present in the demonstration of the said proposition, we give a corrected version of the proposition, and we propose a new theoretical lower bound on the C-bound.
更多查看译文
关键词
majority vote,ensemble methods,learning theory,PAC-Bayesian theory,statistical learning
AI 理解论文
溯源树
样例
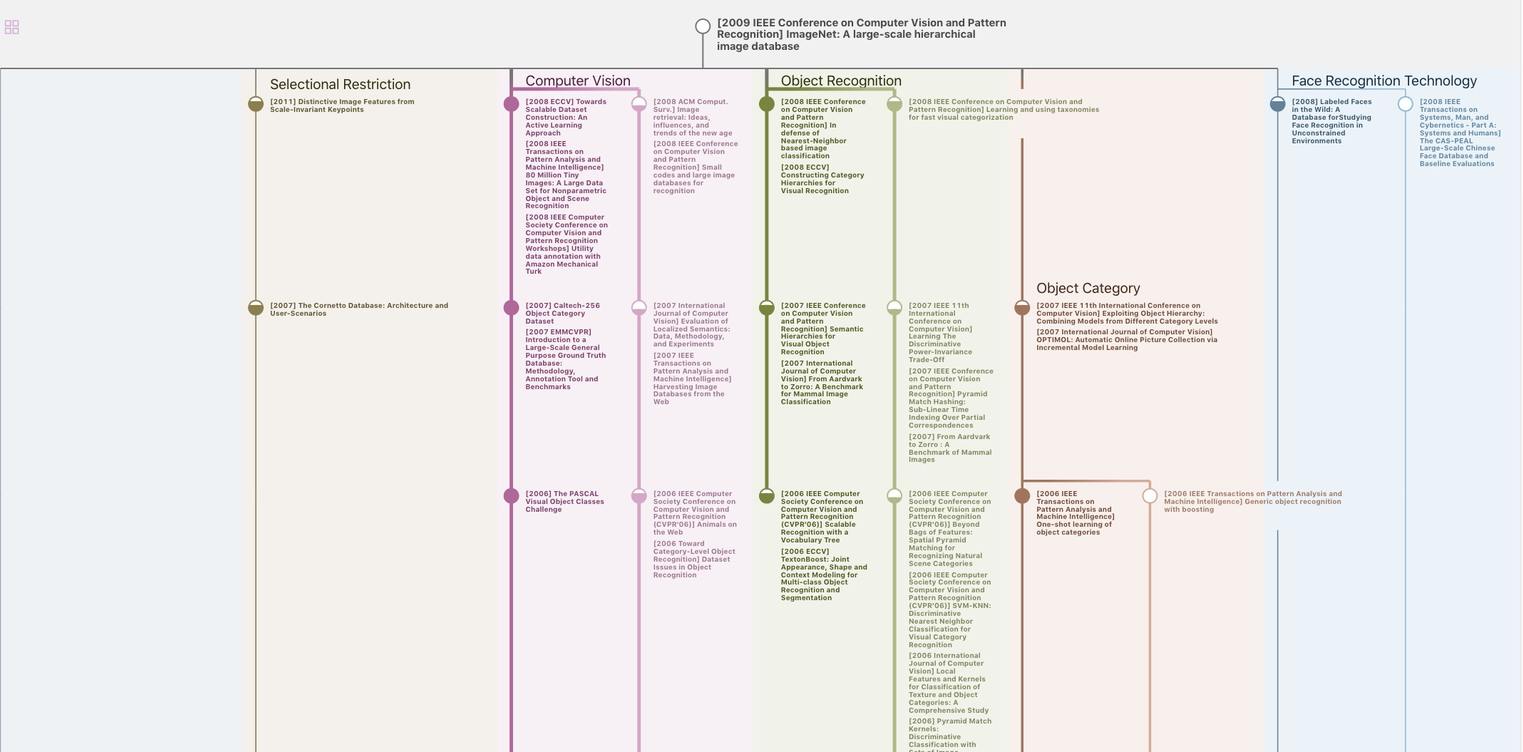
生成溯源树,研究论文发展脉络
Chat Paper
正在生成论文摘要