Prediction of geological composition using recurrent neural networks and shield tunnel boring machine data
CIVIL ENGINEERING AND ENVIRONMENTAL SYSTEMS(2023)
摘要
Tunnel Boring Machines (TBMs) are large-scale excavation tools used commonly in transportation tunnel construction. While tunnelling, TBMs generate data at large scales, often at levels difficult to parse using traditional statistical techniques. Utilising this data can be highly beneficial to obtain a better understanding of TBM excavation conditions, and such understanding can be applied to machine and technique selection. This paper presents a novel method for sequential estimation of geological composition using advanced machine learning algorithms and the data collected from TBMs. In this approach, we use Recurrent Neural Networks and Long Short-Term Memory (RNN-LSTM) models as a hybrid machine learning algorithm for processing sequential and time-series data. The results from an excavation case study demonstrate that the proposed method is an effective approach for sequential estimation of geological composition as encountered by TBM during operation. It is worth noting that TBM data captures the signature of the ground, and the developed model in this study was successful in predicting the ground geological composition even without using the borehole data.
更多查看译文
关键词
Tunnel boring machine,machine learning,recurrent neural networks,underground infrastructure
AI 理解论文
溯源树
样例
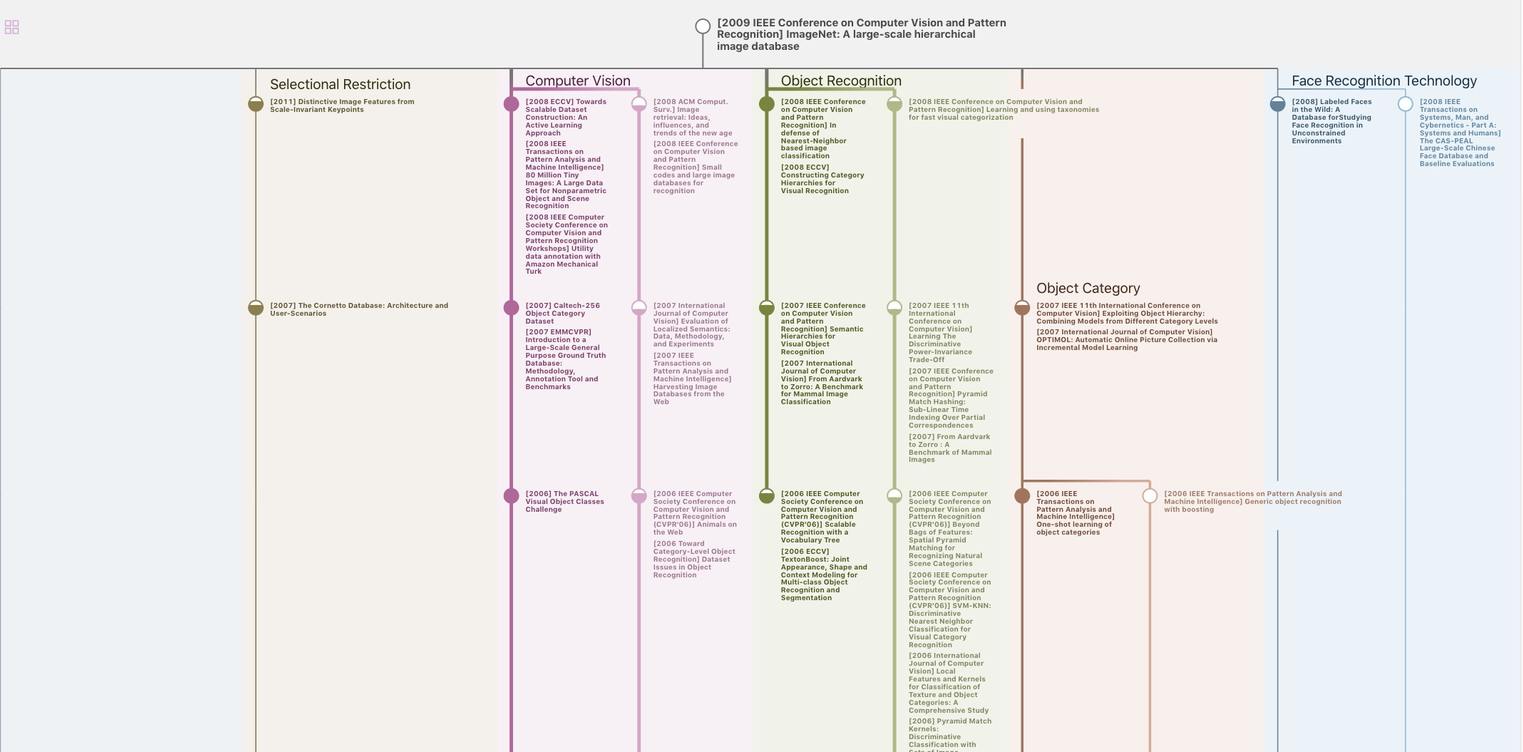
生成溯源树,研究论文发展脉络
Chat Paper
正在生成论文摘要