Game learning-based system identification with binary-valued observations under DoS attacks
INTERNATIONAL JOURNAL OF ADAPTIVE CONTROL AND SIGNAL PROCESSING(2024)
摘要
The rapid progress in computer, communication, and sensor technology has led to the proliferation of cyber-physical systems (CPSs) which have become integral to various sectors. However, their heavy dependence on open communication networks makes them vulnerable to network-based attacks. To tackle these security concerns, this paper delves into game learning-based system identification with binary-valued observations in the presence of Denial-of-Service (DoS) attacks. We first formulate a game model to capture interactions between the attacker and defender. Focusing on piecewise constant DoS attacks, we then devise a defense strategy grounded in game learning principles. This strategy paves the way for crafting estimation algorithms for both the attack strategy and system parameters, with their performance scrutinized in specific stages. Through meticulous analysis and comprehensive numerical simulations, we have observed that the game learning approach outperforms the randomly selected defense strategy in terms of parameter estimation. This provides a novel and reliable approach to address security challenges within CPSs.
更多查看译文
关键词
binary-valued observations,DoS attacks,game learning,system identification
AI 理解论文
溯源树
样例
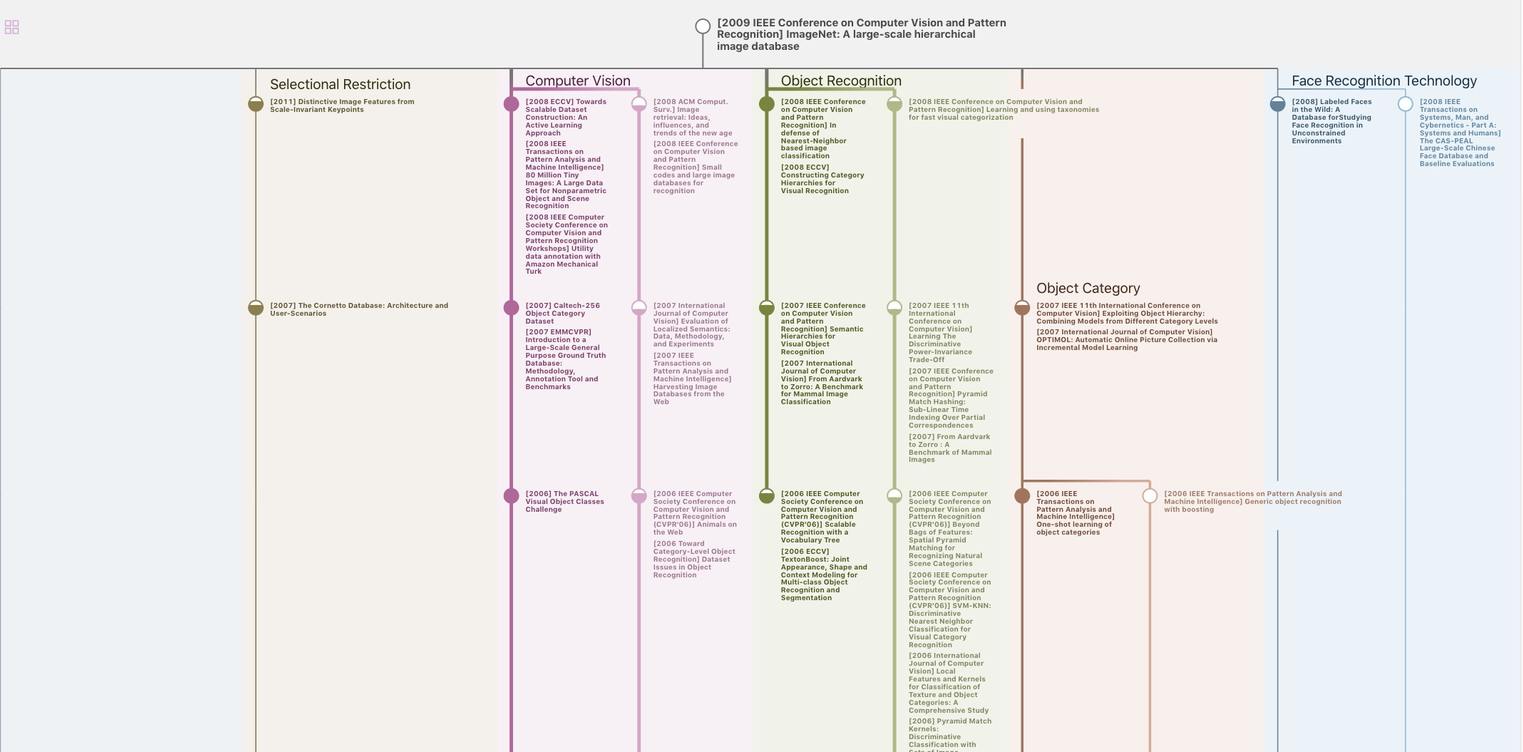
生成溯源树,研究论文发展脉络
Chat Paper
正在生成论文摘要