Enhancing explainability in brain tumor detection: A novel DeepEBTDNet model with LIME on MRI images
INTERNATIONAL JOURNAL OF IMAGING SYSTEMS AND TECHNOLOGY(2024)
摘要
Early detection of brain tumors is vital for improving patient survival rates, yet the manual analysis of the extensive 3D MRI images can be error-prone and time-consuming. This study introduces the Deep Explainable Brain Tumor Deep Network (DeepEBTDNet), a novel deep learning model for binary classification of brain MRIs as tumorous or normal. Employing sub-image dualistic histogram equalization (DSIHE) for enhanced image quality, DeepEBTDNet utilizes 12 convolutional layers with leaky ReLU (LReLU) activation for feature extraction, followed by a fully connected classification layer. Transparency and interpretability are emphasized through the application of the Local Interpretable Model-Agnostic Explanations (LIME) method to explain model predictions. Results demonstrate DeepEBTDNet's efficacy in brain tumor detection, even across datasets, achieving a validation accuracy of 98.96% and testing accuracy of 94.0%. This study underscores the importance of explainable AI in healthcare, facilitating precise diagnoses and transparent decision-making for early brain tumor identification and improved patient outcomes.
更多查看译文
关键词
brain-tumor detection,deep learning,explainable AI,LIME,MRI
AI 理解论文
溯源树
样例
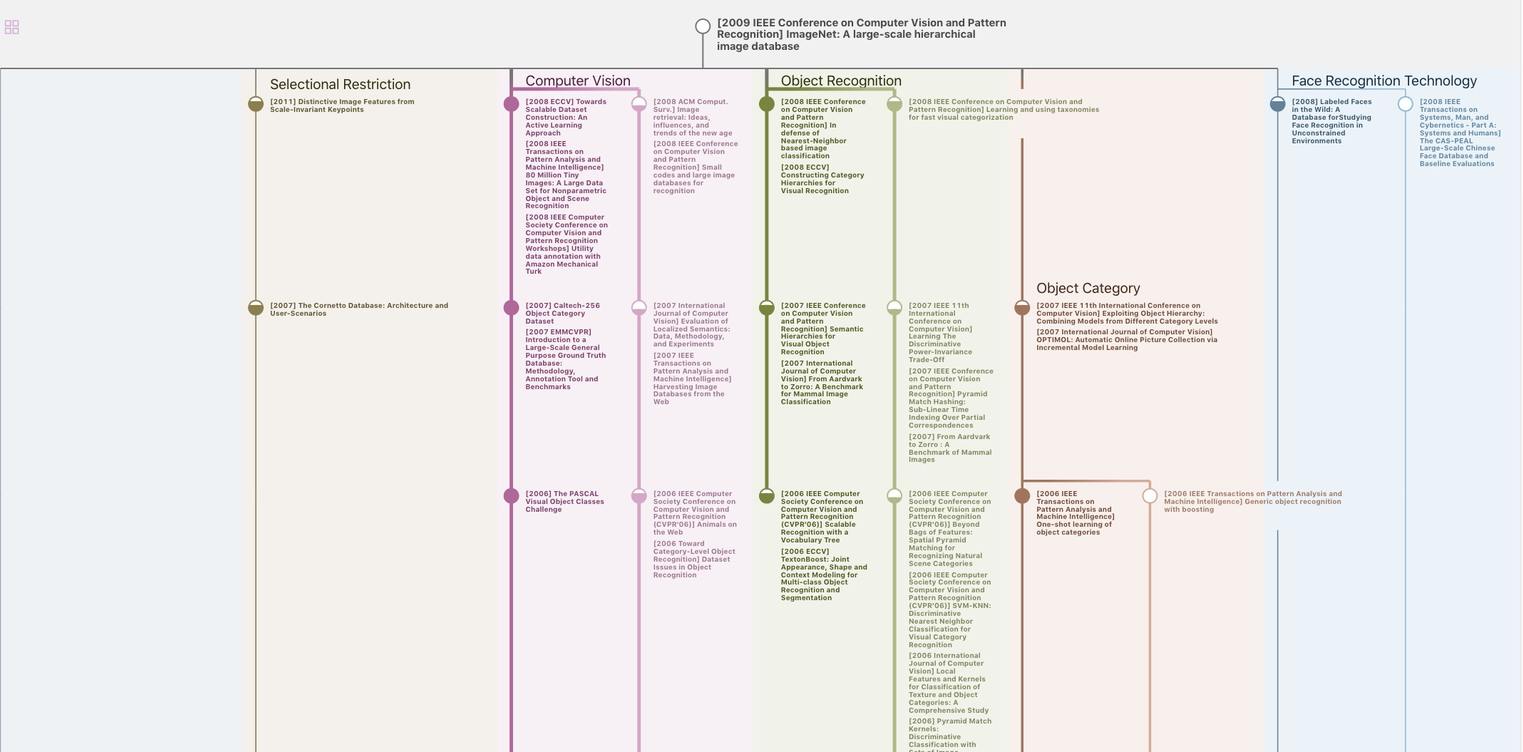
生成溯源树,研究论文发展脉络
Chat Paper
正在生成论文摘要