Degradation Aware Unfolding Network for Spectral Super-Resolution
IEEE GEOSCIENCE AND REMOTE SENSING LETTERS(2024)
摘要
Currently, leading methods for spectral super-resolution (SSR) depend heavily on constructing diverse network architectures in a heuristic manner, in order to learn a full mapping from the RGB image to its corresponding hyperspectral image (HSI). Despite promising results in reconstruction performance, significant challenges remain with respect to model interpretation and the capture of long-range dependencies. In response to these issues, based on a comprehensive exploration of the physical imaging mechanism between spectral response curve (SRC) and HSI, we have developed a novel model-driven degradation-aware unfolding network (DAUNet) in an iterative way. Besides, the learning process is explicitly integrated with the intrinsic generation mechanism of the SSR task. To be specific, we unfold each step into a degradation-aware gradient decent (DAGD) module and a proximal mapping module (PMM), using the framework of maximum a posteriori (MAP) theory. Additionally, to introduce more discriminative learning capabilities to our network, we have further enhanced the PMM architecture by incorporating a fine-grained multihead spectral-wise transformer (FMST) block, which improves global feature representation compared to the channel-wise transformer block. Extensive experiments over several spectral datasets finely demonstrate the superior performance of our method beyond the current representative state-of-the-art (SOTA) SSR methods.
更多查看译文
关键词
Deep convolutional neural network,model-driven,physical generation mechanism,spectral super-resolution (SSR)
AI 理解论文
溯源树
样例
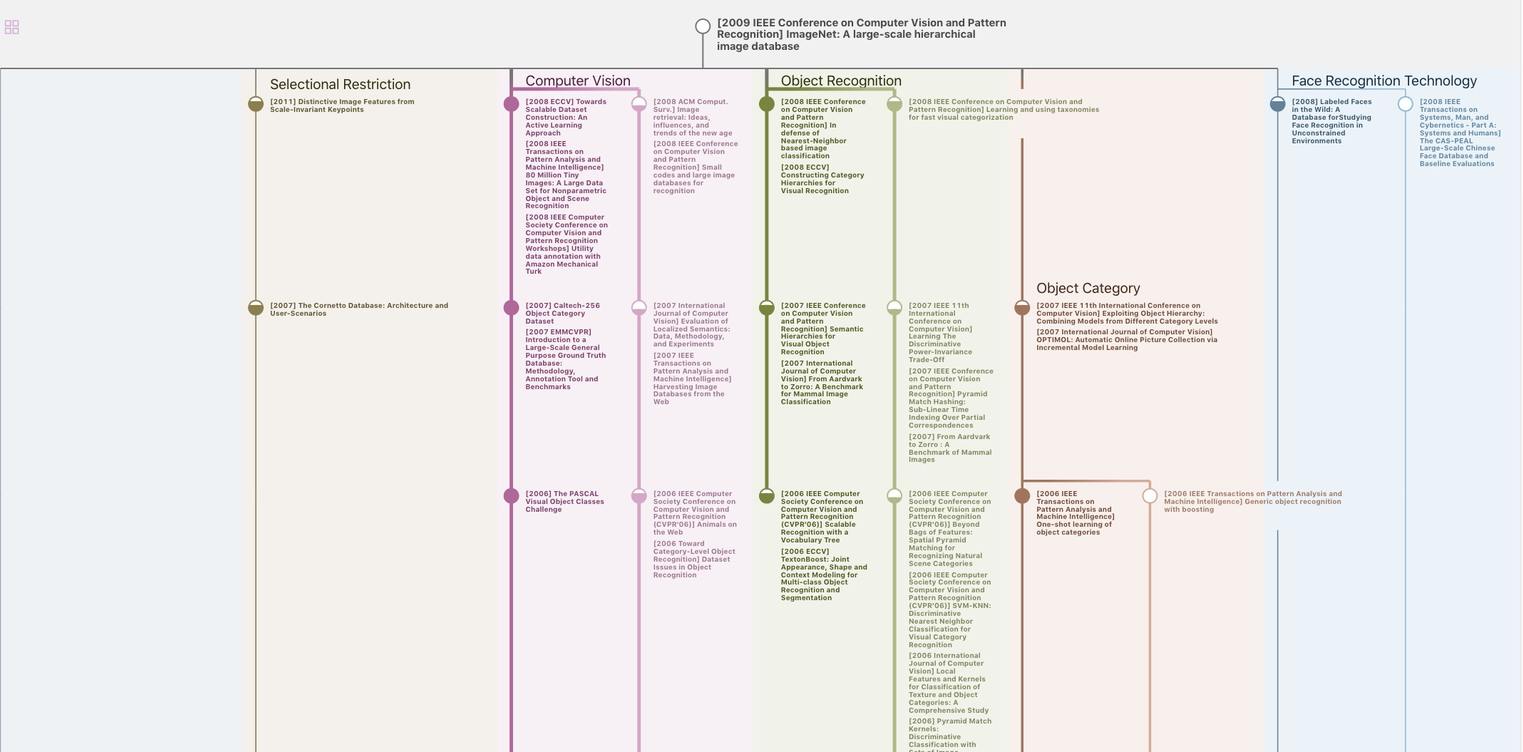
生成溯源树,研究论文发展脉络
Chat Paper
正在生成论文摘要