Novel deep recurrent neural structure with Bayesian distributed backpropagation for biomaterial model involving ferro–copper/blood nanofluids
The European Physical Journal Plus(2024)
摘要
The aim of the presented study is to portray the numerical treatment for biomaterial fluid flow models (BFFMs) involving magnetized ferro and copper nanoparticles dispersed in blood between two rotating disks by exploiting the knacks of layered recurrent neural networks with Bayesian distributed backpropagation (LRNN-BDB). Mathematical formulation of BFFMs is governed with PDEs that are renovated into nonlinear ordinary differential system by operating suitable transformations. The synthetic data are acquired numerically by simulated the implicit backward differentiation formulas to measure the impact of porosity permeability, Darcy–Forchheimer, and Joule heating for BFFMs involving both ferro and copper nanomaterial mixed with blood as a base fluid. The supervised computing paradigm of LRNN-BDB is executed on acquired datasets, and outcomes of LRNN-BDB are regularly overlapping with numerical observations for each variant of BFFMs with negligible error. The stupendous knacks of LRNN-BDB procedures are efficaciously depicted through mean squared error-based iterative convergence plots, adaptive controlling metrics of learning, error distribution statistics on histogram illustrations, and regression measures for exhaustive experimentation on BFFMs.
更多查看译文
AI 理解论文
溯源树
样例
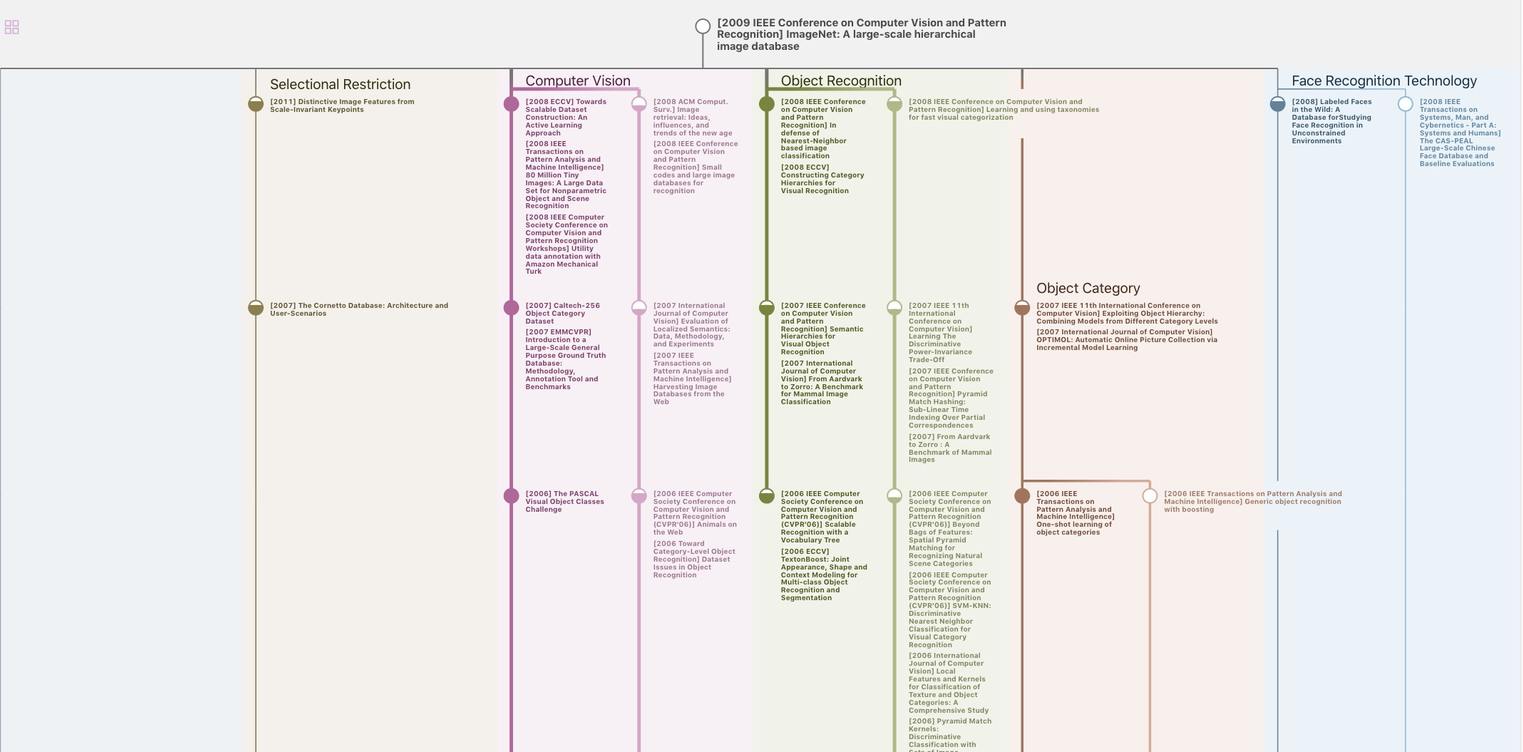
生成溯源树,研究论文发展脉络
Chat Paper
正在生成论文摘要