Tracking treatment effect heterogeneity in evolving environments
Machine Learning(2024)
Abstract
Heterogeneous treatment effect (HTE) estimation plays a crucial role in developing personalized treatment plans across various applications. Conventional approaches assume that the observed data are independent and identically distributed (i.i.d.). In some real applications, however, the assumption does not hold: the environment may evolve, which leads to variations in HTE over time. To enable HTE estimation in evolving environments, we introduce and formulate the online HTE estimation problem. We propose an online ensemble-based HTE estimation method called ETHOS, which is capable of adapting to unknown evolving environments by ensembling the outputs of multiple base estimators that track environmental changes at different scales. Theoretical analysis reveals that ETHOS achieves an optimal expected dynamic regret O(√(T(1+P_T))) , where T denotes the number of observed examples and P_T characterizes the intensity of environment changes. The achieved dynamic regret ensures that our method consistently approaches the optimal online estimators as long as the evolution of the environment is moderate. We conducted extensive experiments on three common benchmark datasets with various environment evolving mechanisms. The results validate the theoretical analysis and the effectiveness of our proposed method.
MoreTranslated text
Key words
Causal inference,Heterogeneous treatment effects,Treatment effect estimation,Evolving environments
AI Read Science
Must-Reading Tree
Example
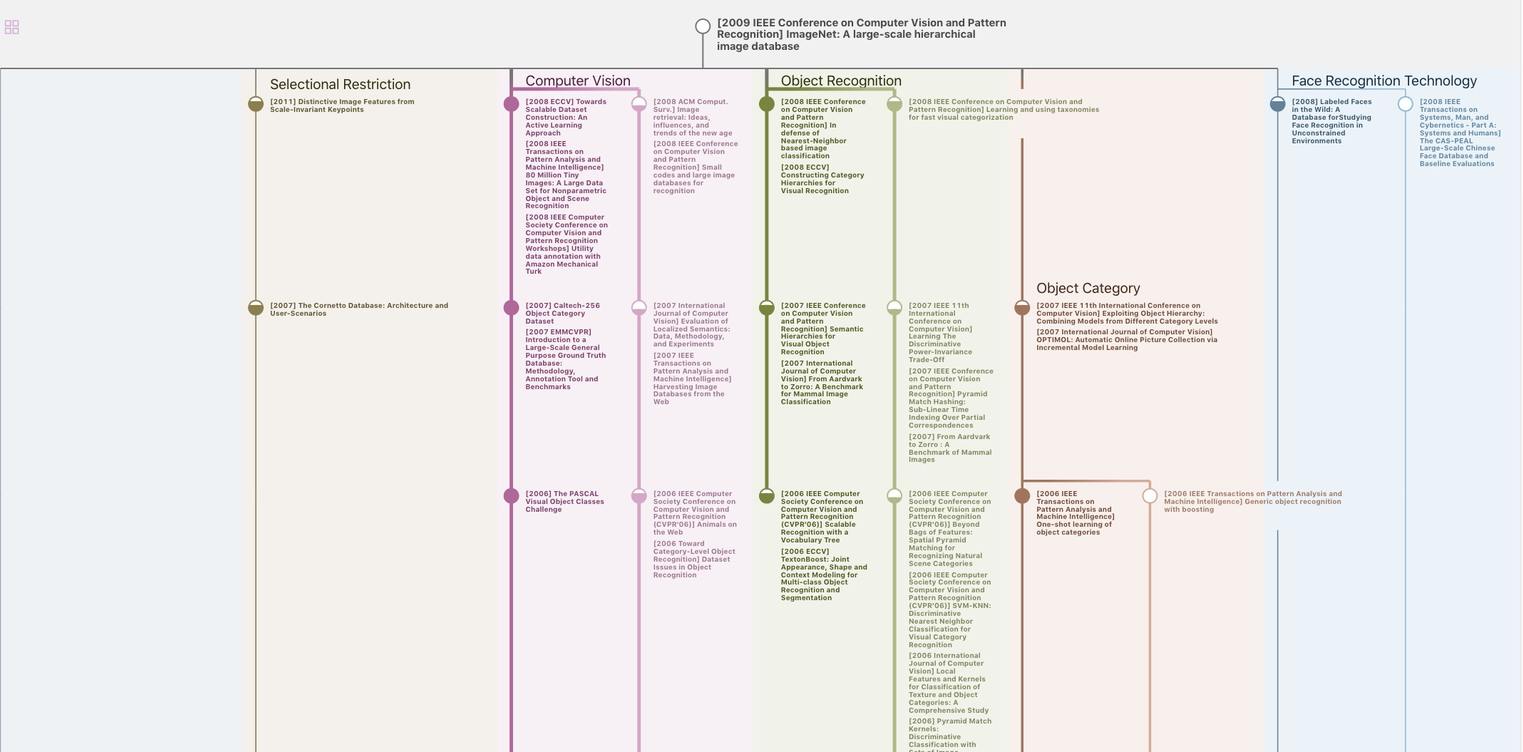
Generate MRT to find the research sequence of this paper
Chat Paper
Summary is being generated by the instructions you defined