Fast High Dynamic Range Radiance Fields for Dynamic Scenes
CoRR(2024)
摘要
Neural Radiances Fields (NeRF) and their extensions have shown great success
in representing 3D scenes and synthesizing novel-view images. However, most
NeRF methods take in low-dynamic-range (LDR) images, which may lose details,
especially with nonuniform illumination. Some previous NeRF methods attempt to
introduce high-dynamic-range (HDR) techniques but mainly target static scenes.
To extend HDR NeRF methods to wider applications, we propose a dynamic HDR NeRF
framework, named HDR-HexPlane, which can learn 3D scenes from dynamic 2D images
captured with various exposures. A learnable exposure mapping function is
constructed to obtain adaptive exposure values for each image. Based on the
monotonically increasing prior, a camera response function is designed for
stable learning. With the proposed model, high-quality novel-view images at any
time point can be rendered with any desired exposure. We further construct a
dataset containing multiple dynamic scenes captured with diverse exposures for
evaluation. All the datasets and code are available at
.
更多查看译文
AI 理解论文
溯源树
样例
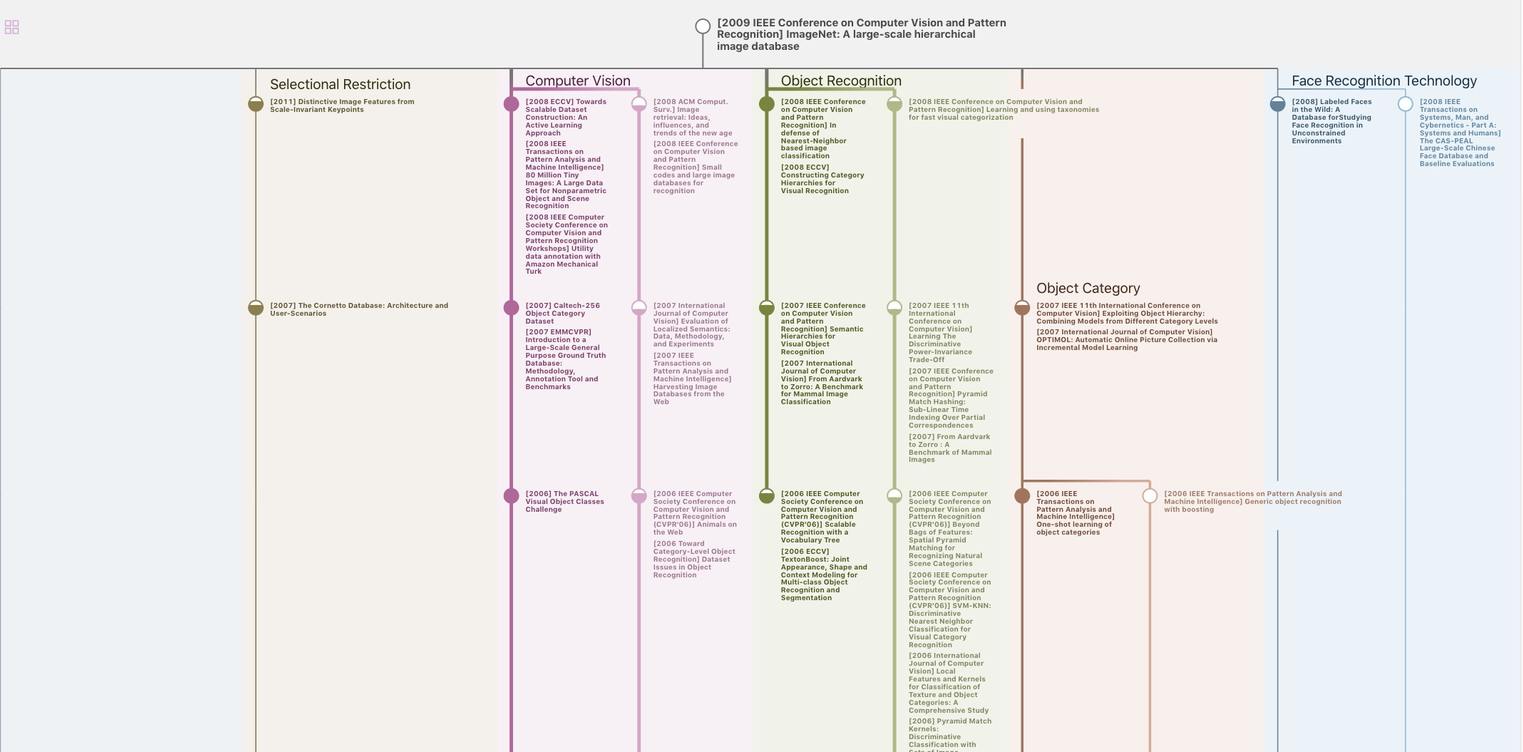
生成溯源树,研究论文发展脉络
Chat Paper
正在生成论文摘要