Eating Speed Measurement Using Wrist-Worn IMU Sensors in Free-Living Environments
CoRR(2023)
摘要
Eating speed is an important indicator that has been widely scrutinized in
nutritional studies. The relationship between eating speed and several
intake-related problems such as obesity, diabetes, and oral health has received
increased attention from researchers. However, existing studies mainly use
self-reported questionnaires to obtain participants' eating speed, where they
choose options from slow, medium, and fast. Such a non-quantitative method is
highly subjective and coarse in individual level. In this study, we propose a
novel approach to measure eating speed in free-living environments
automatically and objectively using wrist-worn inertial measurement unit (IMU)
sensors. Specifically, a temporal convolutional network combined with a
multi-head attention module (TCN-MHA) is developed to detect bites (including
eating and drinking gestures) from free-living IMU data. The predicted bite
sequences are then clustered to eating episodes. Eating speed is calculated by
using the time taken to finish the eating episode to divide the number of
bites. To validate the proposed approach on eating speed measurement, a 7-fold
cross validation is applied to the self-collected fine-annotated full-day-I
(FD-I) dataset, and a hold-out experiment is conducted on the full-day-II
(FD-II) dataset. The two datasets are collected from 61 participants in
free-living environments with a total duration of 513 h, which are publicly
available. Experimental results shows that the proposed approach achieves a
mean absolute percentage error (MAPE) of 0.110 and 0.146 in the FD-I and FD-II
datasets, respectively, showcasing the feasibility of automated eating speed
measurement. To the best of our knowledge, this is the first study
investigating automated eating speed measurement.
更多查看译文
AI 理解论文
溯源树
样例
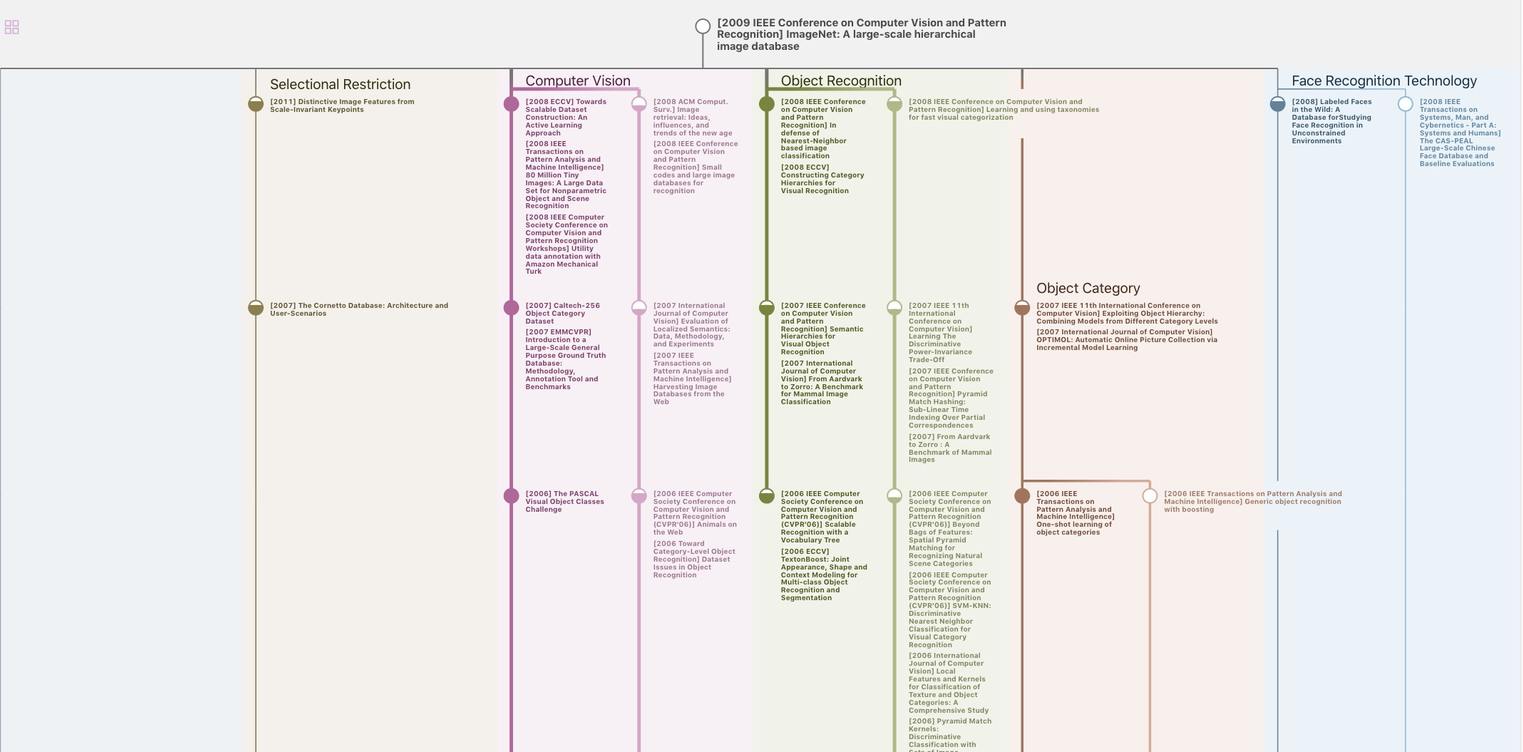
生成溯源树,研究论文发展脉络
Chat Paper
正在生成论文摘要