Enhancing Essay Scoring with Adversarial Weights Perturbation and Metric-specific AttentionPooling
CoRR(2024)
摘要
The objective of this study is to improve automated feedback tools designed for English Language Learners (ELLs) through the utilization of data science techniques encompassing machine learning, natural language processing, and educational data analytics. Automated essay scoring (AES) research has made strides in evaluating written essays, but it often overlooks the specific needs of English Language Learners (ELLs) in language development. This study explores the application of BERT-related techniques to enhance the assessment of ELLs' writing proficiency within AES. To address the specific needs of ELLs, we propose the use of DeBERTa, a state-of-the-art neural language model, for improving automated feedback tools. DeBERTa, pretrained on large text corpora using self-supervised learning, learns universal language representations adaptable to various natural language understanding tasks. The model incorporates several innovative techniques, including adversarial training through Adversarial Weights Perturbation (AWP) and Metric-specific AttentionPooling (6 kinds of AP) for each label in the competition. The primary focus of this research is to investigate the impact of hyperparameters, particularly the adversarial learning rate, on the performance of the model. By fine-tuning the hyperparameter tuning process, including the influence of 6AP and AWP, the resulting models can provide more accurate evaluations of language proficiency and support tailored learning tasks for ELLs. This work has the potential to significantly benefit ELLs by improving their English language proficiency and facilitating their educational journey.
更多查看译文
关键词
English Language Learners (ELLs),DeBERTa (Decoding-enhanced BERT),Metric-specific Attention Pooling,Adversarial Weights Perturbation
AI 理解论文
溯源树
样例
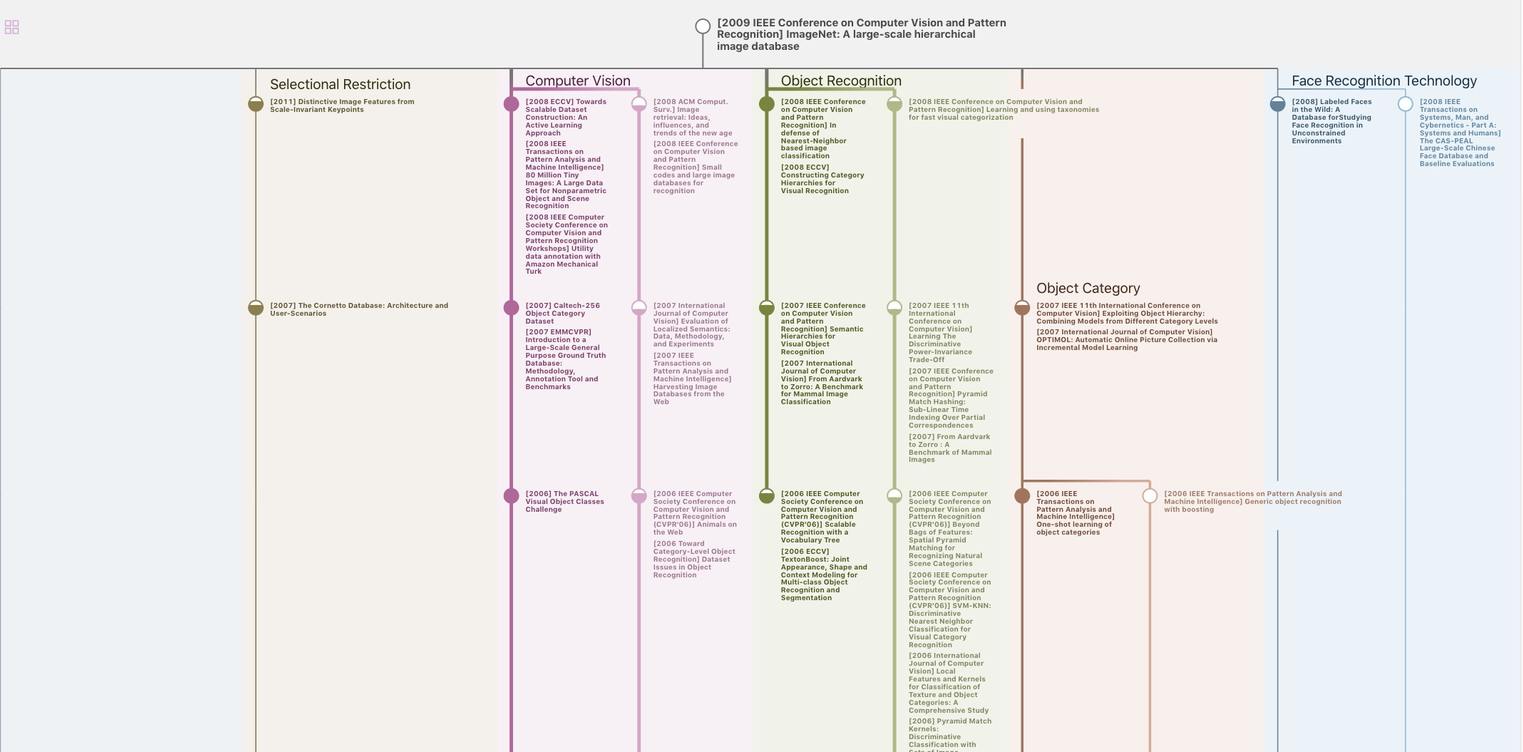
生成溯源树,研究论文发展脉络
Chat Paper
正在生成论文摘要