Ensemble Transfer Learning for Time Series Forecasting: A Comparative Sensitivity Analysis of Two Shallow Networks.
2023 30th IEEE International Conference on Electronics, Circuits and Systems (ICECS)(2023)
摘要
Given the success that transfer learning has recorded in various domains, the interest in its application to time series forecasting is growing. This paper contributes to tackling the need for empirical studies by providing practical guidelines for TL methods and approaches for time series forecasting. To that effect, using a set of four novel TL metrics, we conduct a comparative sensitivity analysis on two shallow networks for transfer learning, namely the MLP and the LSTM. While the empirical results show the impact of the width of the neural network on transfer learning performance, an interesting question is raised concerning the dimensioning of the neural network for optimal performance when designing the transfer learning process.
更多查看译文
关键词
Transfer learning,Time series forecasting,Sensitivity Analysis,Deep Learning,Ensemble learning
AI 理解论文
溯源树
样例
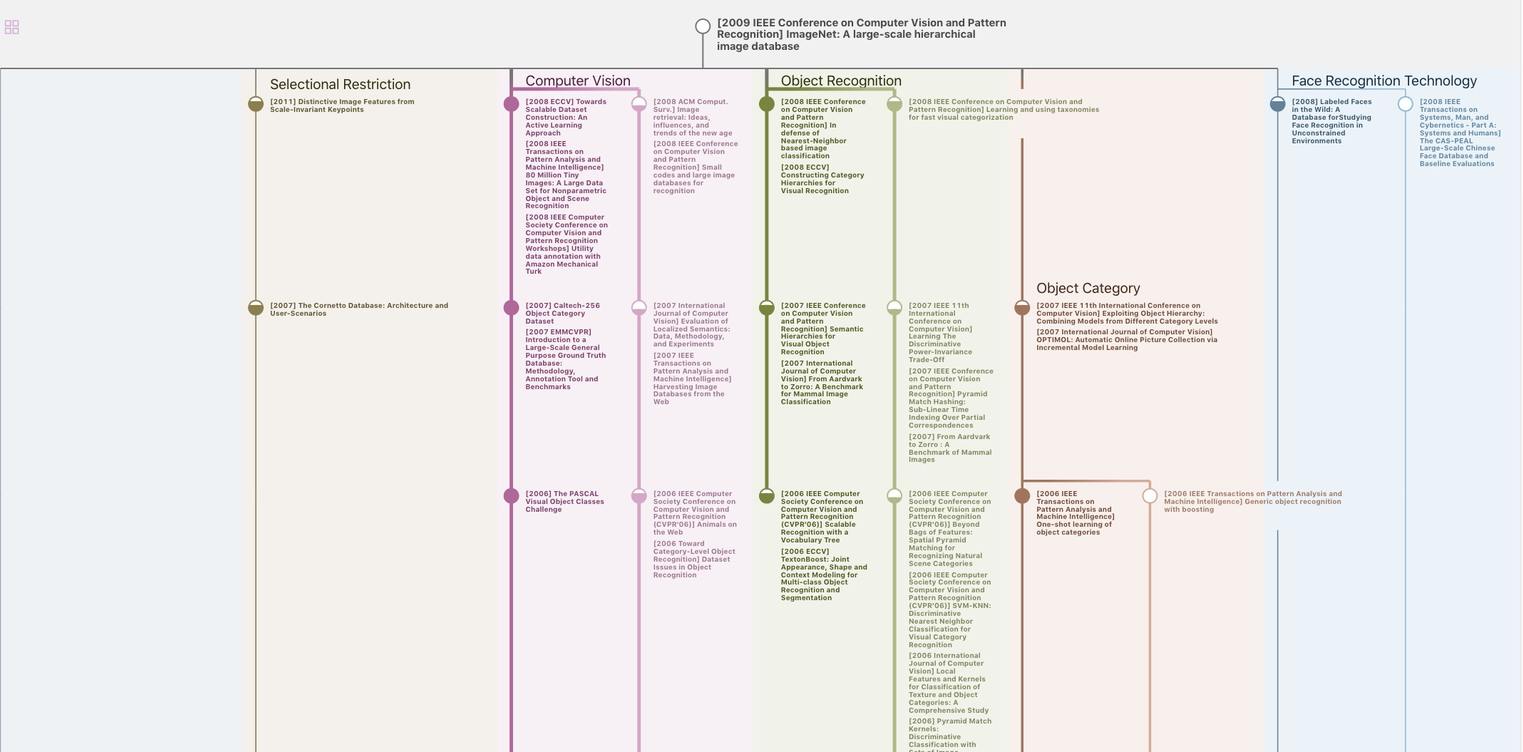
生成溯源树,研究论文发展脉络
Chat Paper
正在生成论文摘要