Advanced Mathematics Exercise Recommendation Based on Automatic Knowledge Extraction and Multilayer Knowledge Graph
IEEE TRANSACTIONS ON LEARNING TECHNOLOGIES(2024)
Abstract
Higher education is rapidly growing in the online learning landscape. However, current personalized recommendation techniques struggle with the precise extraction of complex mathematical semantics, hindering accurate perception of learners' cognitive states and relevance of recommendations. This article proposes a framework for extracting complex mathematical semantics and providing personalized exercise recommendations. We design a tree-based position encoding method to enhance the accuracy of positional representation for mathematical expressions in the pretrained model, aiming to improve the performance of downstream tasks. We propose an automatic method for extracting knowledge attributes based on expert annotations, enabling interpretable cognitive diagnosis. Furthermore, we employ sequential pattern mining to discover the knowledge usage patterns in exercises, generate learning paths using a multilayer knowledge graph, and leverage cognitive diagnostic results to enhance the relevance of recommendations. Experimental results show a 2.0% improvement in mathematical symbol embedding on mathematical formula retrieval tasks and knowledge attribute extraction accuracy ranging from 66.5% to 81.7%. Learners' posttest scores significantly improve during group testing with good consistency between online cognitive diagnosis and self-diagnosis.
MoreTranslated text
Key words
Feature extraction,Mathematical models,Matrix decomposition,Data mining,Ontologies,Knowledge graphs,Analytical models,Cognitive diagnosis,personalized recommendation,text analysis,word embedding
AI Read Science
Must-Reading Tree
Example
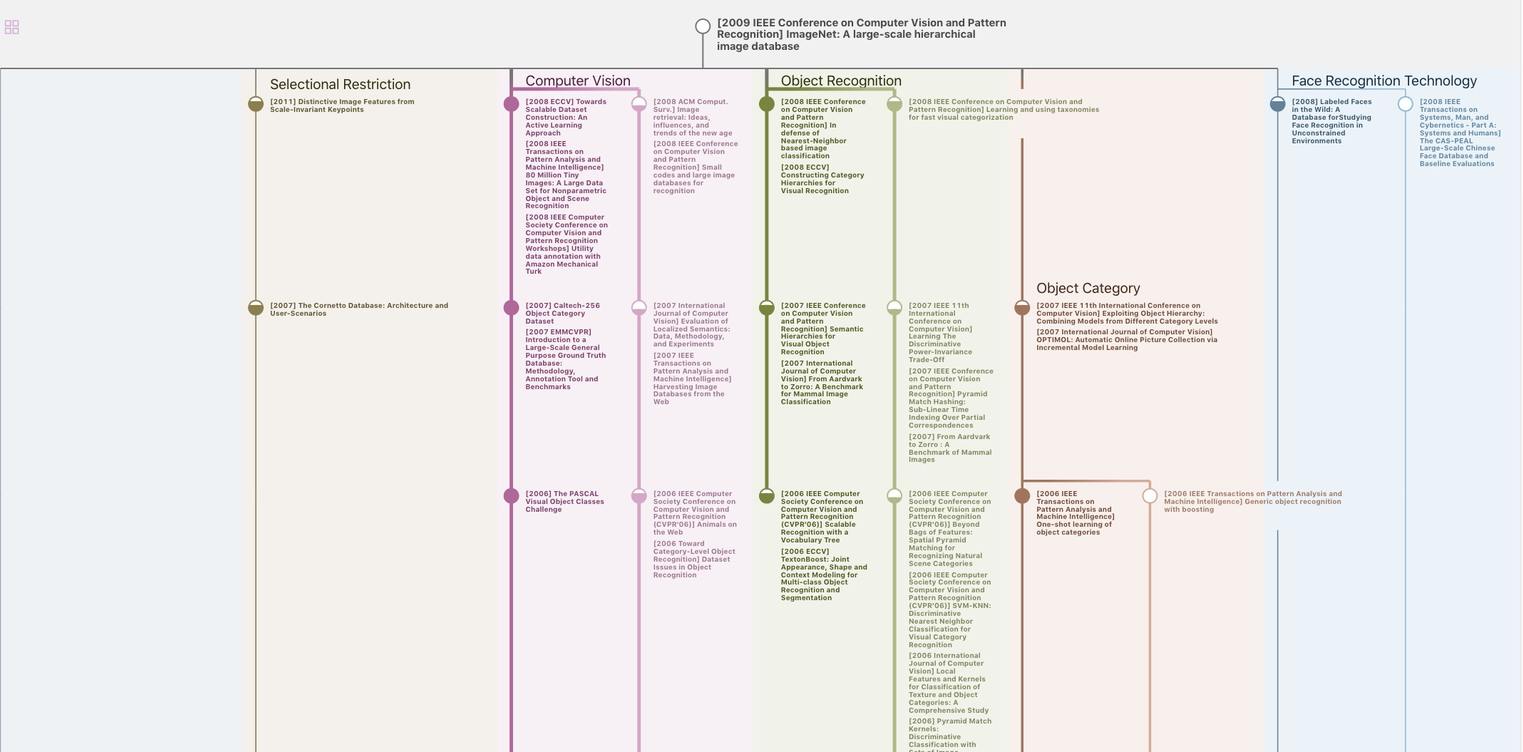
Generate MRT to find the research sequence of this paper
Chat Paper
Summary is being generated by the instructions you defined