Vision Transformer-based Decision Support for Neurosurgical Intervention in Acute Traumatic Brain Injury: Automated Surgical Intervention Support Tool
RADIOLOGY-ARTIFICIAL INTELLIGENCE(2024)
摘要
Purpose: To develop an automated triage tool to predict neurosurgical intervention for patients with traumatic brain injury (TBI). Materials and Methods: A provincial trauma registry was reviewed to retrospectively identify patients with TBI from 2005 to 2022 treated at a specialized Canadian trauma center. Model training, validation, and testing were performed using head CT scans with binary reference standard patient -level labels corresponding to whether the patient received neurosurgical intervention. Performance and accuracy of the model, the Automated Surgical Intervention Support Tool for TBI (ASIST-TBI), were also assessed using a held -out consecutive test set of all patients with TBI presenting to the center between March 2021 and September 2022. Results: Head CT scans from 2806 patients with TBI (mean age, 57 years +/- 22 [SD]; 1955 [70%] men) were acquired between 2005 and 2021 and used for training, validation, and testing. Consecutive scans from an additional 612 patients (mean age, 61 years +/- 22; 443 [72%] men) were used to assess the performance of ASIST-TBI. There was accurate prediction of neurosurgical intervention with an area under the receiver operating characteristic curve (AUC) of 0.92 (95% CI: 0.88, 0.94), accuracy of 87% (491 of 562), sensitivity of 87% (196 of 225), and specificity of 88% (295 of 337) on the test dataset. Performance on the held -out test dataset remained robust with an AUC of 0.89 (95% CI: 0.85, 0.91), accuracy of 84% (517 of 612), sensitivity of 85% (199 of 235), and specificity of 84% (318 of 377). Conclusion: A novel deep learning model was developed that could accurately predict the requirement for neurosurgical intervention using acute TBI CT scans.
更多查看译文
AI 理解论文
溯源树
样例
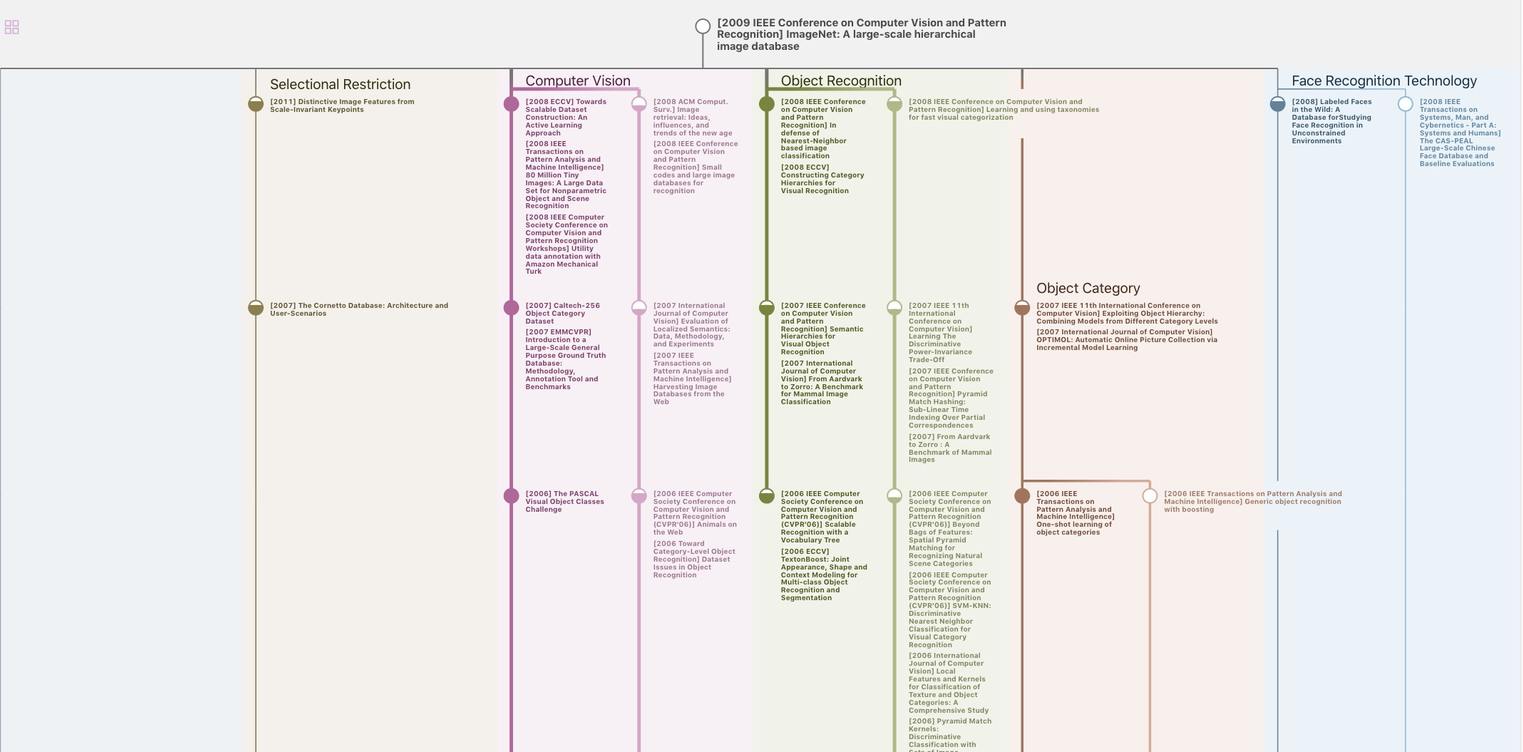
生成溯源树,研究论文发展脉络
Chat Paper
正在生成论文摘要