The Synergistic Effect between Metal and Sulfur Vacancy to Boost CO2 Reduction Efficiency: A Study on Descriptor Transferability and Activity Prediction
JACS Au(2024)
Abstract
Both metal center active sites and vacancies can influence the catalytic activity of a catalyst. A quantitative model to describe the synergistic effect between the metal centers and vacancies is highly desired. Herein, we proposed a machine learning model to evaluate the synergistic index, P-Syn, which is learned from the possible pathways for CH4 production from CO2 reduction reaction (CO2RR) on 26 metal-anchored MoS2 with and without sulfur vacancy. The data set consists of 1556 intermediate structures on metal-anchored MoS2, which are used for training. The 2028 structures from the literature, comprising both single active site and dual active sites, are used for external test. The XGBoost model with 3 features, including electronegativity, d-shell valence electrons of metal, and the distance between metal and vacancy, exhibited satisfactory prediction accuracy on limiting potential. Fe@Sv-MoS2 and Os@MoS2 are predicted to be promising CO2RR catalysts with high stability, low limiting potential, and high selectivity against hydrogen evolution reactions (HER). Based on some easily accessible descriptors, transferability can be achieved for both porous materials and 2D materials in predicting the energy change in the CO2RR and nitrogen reduction reaction (NRR). Such a predictive model can also be applied to predict the synergistic effect of the CO2RR in other oxygen and tungsten vacancy systems.
MoreTranslated text
Key words
CO2 reduction,metal-vacancy synergisticeffect,MoS2,machine learning,DFT calculations
AI Read Science
Must-Reading Tree
Example
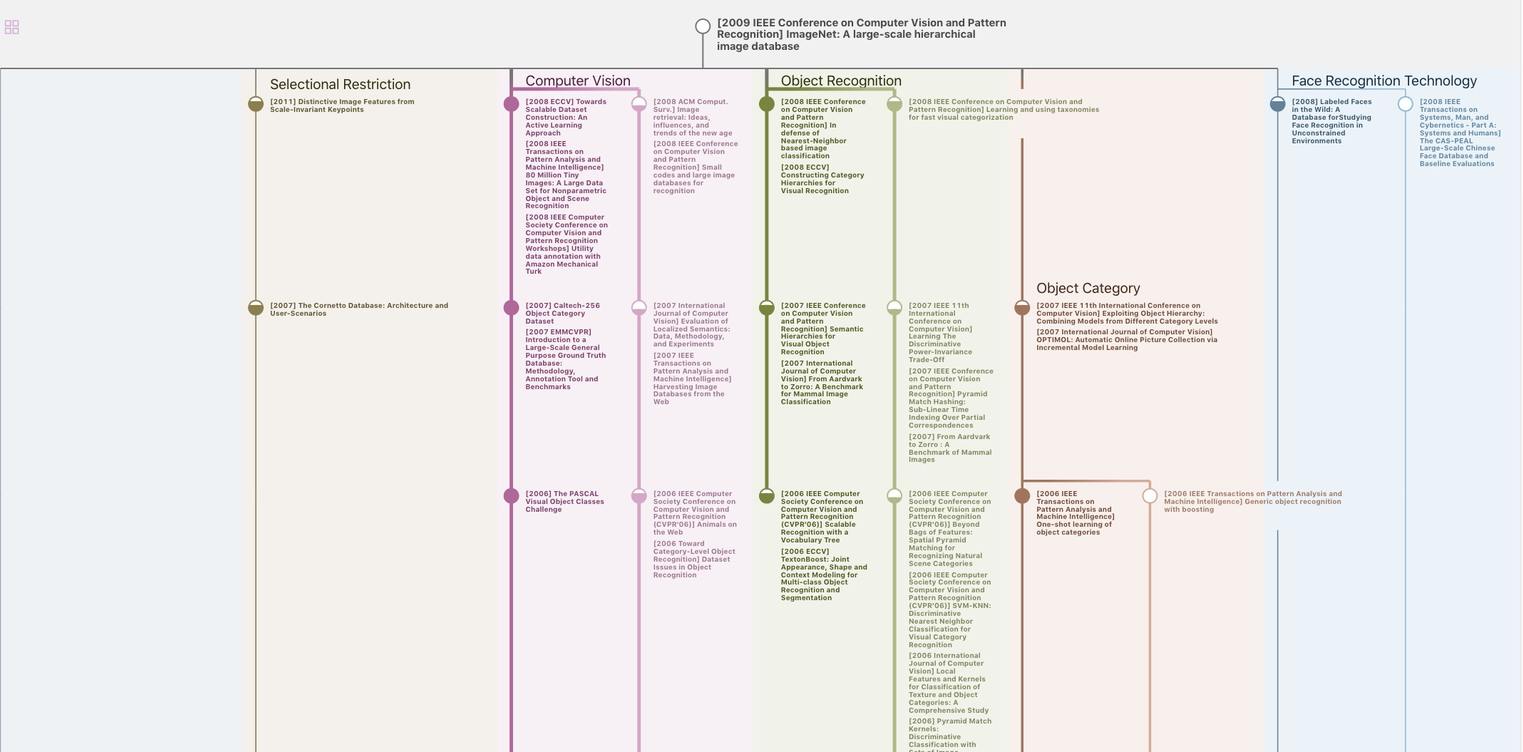
Generate MRT to find the research sequence of this paper
Chat Paper
Summary is being generated by the instructions you defined