An efficient EEG signal fading processing framework based on the cognitive limbic system and deep learning
Applied Intelligence(2024)
摘要
Non-invasive electroencephalography (EEG) is a technique for monitoring brain activity that is valuable in the diagnosis and study of the brain. However, due to factors such as brain-computer interface (BCI) devices deficiency, dynamic network limitations, and subject issues, EEG signals may fade throughout the entire process from signal generation to acquisition. The fading of EEG signals can cause changes in the data distribution, blur the information and have a negative impact on subsequent research and application. In order to reduce the adverse effects of data fading, this paper proposes a hierarchical bidirectional long-short term memory (LSTM)-Attention network based on cognitive brain limbic system (HBLANet). HBLANet classifies randomly fading signals multiple times by way of a dimensionality-reducing classification algorithm in order to narrow down the feature interval processed by a single neural network. Then the different types of EEG signals acquired from the classification are processed in a more focused manner using a bidirectional LSTM-Attention network. In this paper, the overall performance of the network is greatly improved by decomposing complex signal processing tasks into smaller tasks. The model is evaluated on the EEG-denoisenet dataset and compared with competitive networks such as feature pyramid network (FPN), UNet, MultiResUNet, 1D-ResCNN etc., and the results show that the proposed network achieves better fading processing outcomes. In the overall experiment, the processed EEG signals achieve the relative root mean squared error (RRMSE) value of 0.009, the signal-to-noise ratio (SNR) of 32.78, and the correlation coefficient (CC) of 0.98. Furthermore, the denoising task in the overall experiment achieves even more exciting results, with a SNR of 40.48 and a CC of 0.991 for the processed EEG signal in the case of processing high SNR signals (the range of the SNR is from -2 to 2). Therefore, we believe that the framework has an important reference value for future research on signal quality restoration.
更多查看译文
关键词
Signal fading,Self-attention,Long-short term memory (LSTM),Support vector machine (SVM),Cognitive limbic system,Brain-computer interface (BCI)
AI 理解论文
溯源树
样例
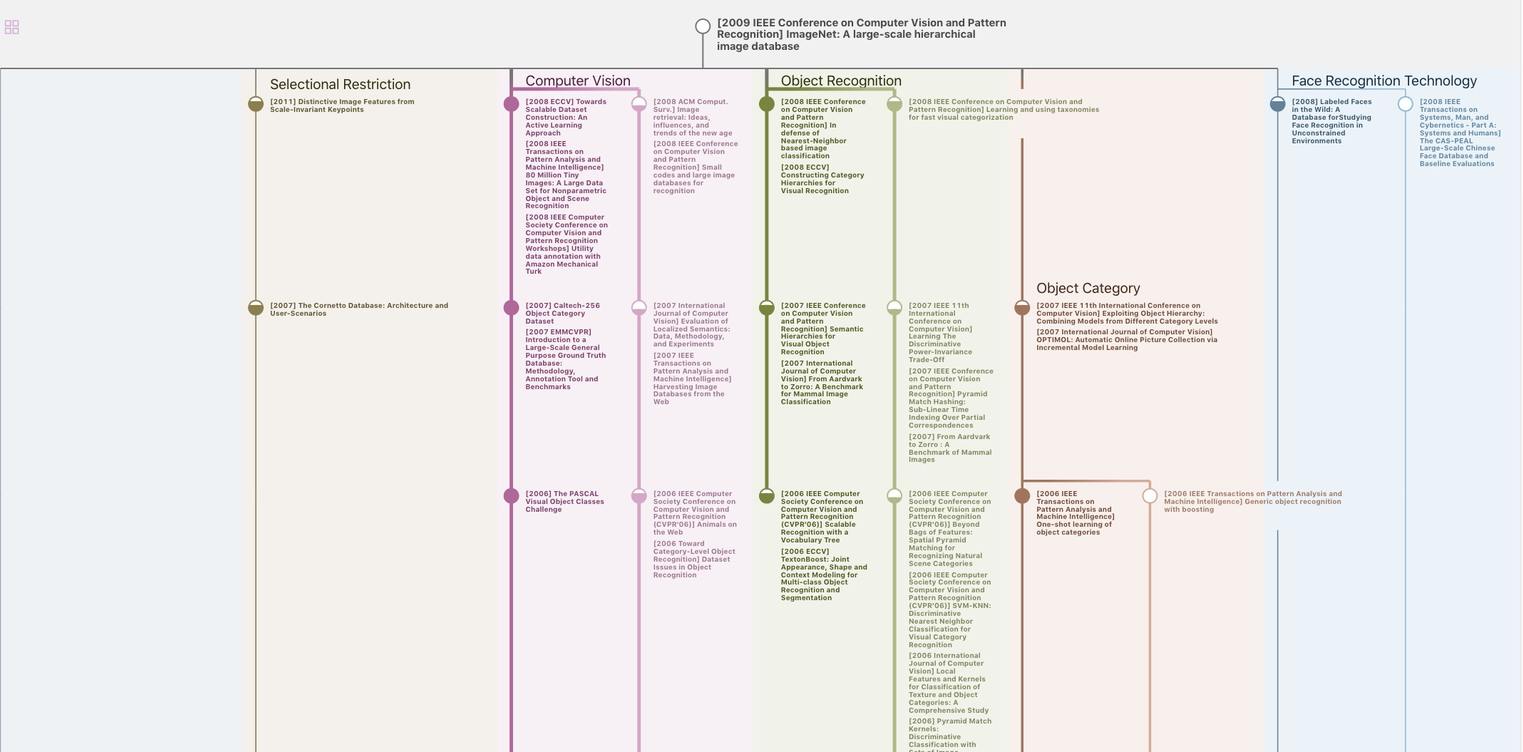
生成溯源树,研究论文发展脉络
Chat Paper
正在生成论文摘要