High dimensional mislabeled learning
NEUROCOMPUTING(2024)
摘要
High-dimensional mislabeled learning is essential in AI theory and applications but rarely investigated. In this study, we present a novel learning technique to detect and rectify high-dimensional mislabeled data using proposed Feature Self-Organizing Map (fSOM) along with relevant theoretical findings. When combined with the reproducible multi-class SVM learning approach, this method forms the backbone of our proposed psychiatric map (pMAP) diagnosis algorithm. This algorithm specifically addresses the real-world challenge in psychiatry of differentiating between Schizophrenia and Bipolar disorder using SNP data. The pMAP diagnosis not only offers a more precise and dependable mechanism for identifying misdiagnoses compared to state-of-the-art deep learning and machine learning models but also unveils previously unreported latent psychiatry subtypes. Furthermore, this research sheds new light on the pathology of psychiatric disorders. By mapping the evolution and internal transitions of psychiatric states and analyzing the relative entropies between various psychiatric maps, we unveil avenues to both augment and refine traditional psychiatric research. To our knowledge, this represents the inaugural study in high-dimensional mislabeled learning, poised to inspire further exploration in the domain.
更多查看译文
关键词
Mislabeled learning,High -dimensional data,Feature self -organizing learning,Psychiatric disorders
AI 理解论文
溯源树
样例
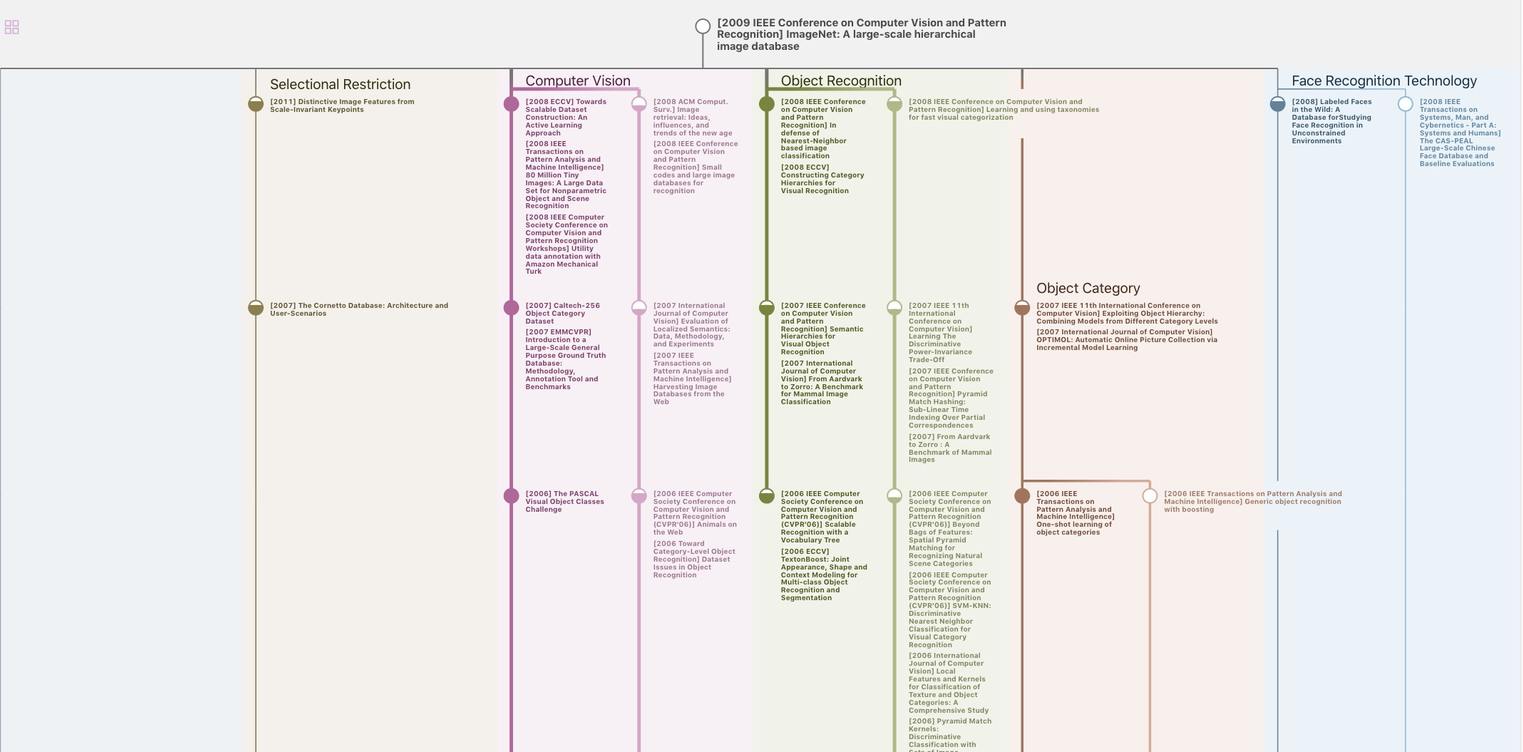
生成溯源树,研究论文发展脉络
Chat Paper
正在生成论文摘要