Information Flow Rate for Cross-Correlated Stochastic Processes
IEEE TRANSACTIONS ON SIGNAL PROCESSING(2024)
Abstract
Causal inference seeks to identify cause-and-effect interactions in coupled systems. A recently proposed method by Liang detects causal relations by quantifying the direction and magnitude of information flow between time series. The theoretical formulation of information flow for stochastic dynamical systems provides a general expression and a data-driven statistic for the rate of entropy transfer between different system units. To advance understanding of information flow rate in terms of intuitive concepts and physically meaningful parameters, we investigate statistical properties of the data-driven information flow rate between coupled stochastic processes. We derive relations between the expectation of the information flow rate statistic and properties of the auto- and cross-correlation functions. Thus, we elucidate the dependence of the information flow rate on the analytical properties and characteristic times of the correlation functions. Our analysis provides insight into the influence of the sampling step, the strength of cross-correlations, and the temporal delay of correlations on information flow rate. We support the theoretical results with numerical simulations of correlated Gaussian processes.
MoreTranslated text
Key words
Kernel,Time series analysis,Correlation,Mathematical models,Entropy,Gaussian processes,Time measurement,Information flow,causality analysis,Gaussian process,entropy,covariance kernel
AI Read Science
Must-Reading Tree
Example
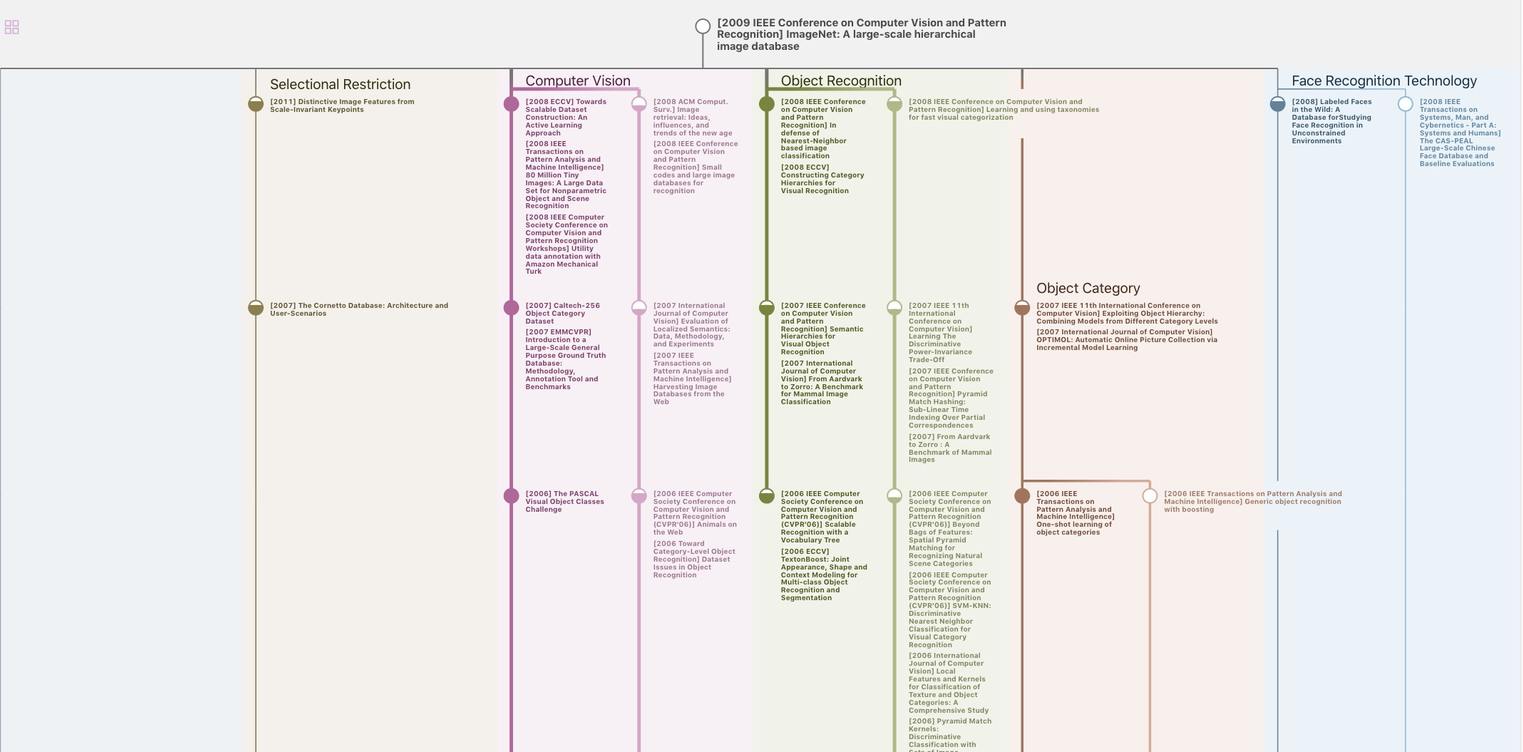
Generate MRT to find the research sequence of this paper
Chat Paper
Summary is being generated by the instructions you defined