An Improved YOLOv5 Algorithm for Drowning Detection in the Indoor Swimming Pool
APPLIED SCIENCES-BASEL(2024)
关键词
YOLOv5,drowning detection,self-made dataset,coordinate attention,drone
AI 理解论文
溯源树
样例
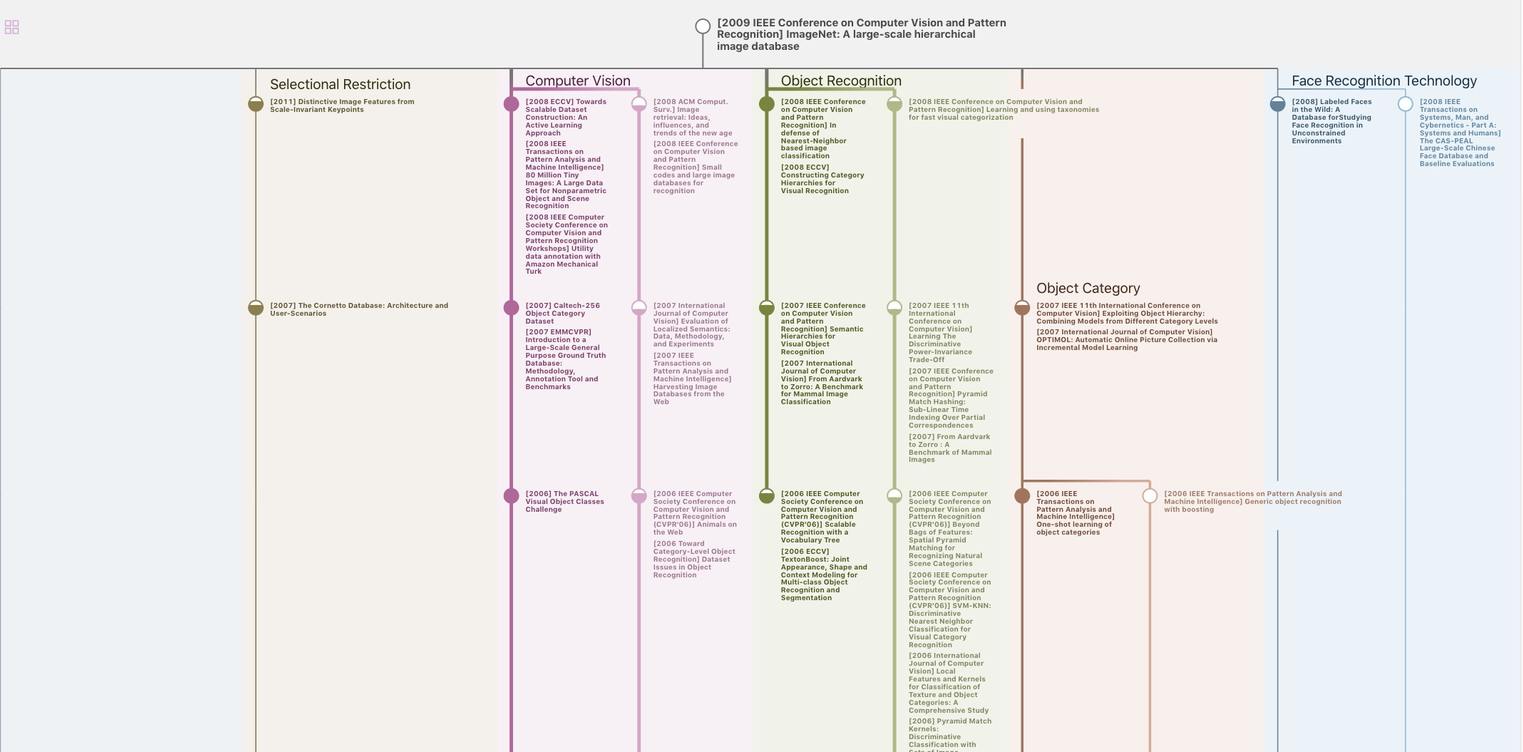
生成溯源树,研究论文发展脉络
Chat Paper
正在生成论文摘要
APPLIED SCIENCES-BASEL(2024)