An Efficient Graph Convolutional RVFL Network for Hyperspectral Image Classification
REMOTE SENSING(2024)
摘要
Graph convolutional networks (GCN) have emerged as a powerful alternative tool for analyzing hyperspectral images (HSIs). Despite their impressive performance, current works strive to make GCN more sophisticated through either elaborate architecture or fancy training tricks, making them prohibitive for HSI data in practice. In this paper, we present a Graph Convolutional RVFL Network (GCRVFL), a simple but efficient GCN for hyperspectral image classification. Specifically, we generalize the classic RVFL network into the graph domain by using graph convolution operations. This not only enables RVFL to handle graph-structured data, but also avoids iterative parameter adjustment by employing an efficient closed-form solution. Unlike previous works that perform HSI classification under a transductive framework, we regard HSI classification as a graph-level classification task, which makes GCRVFL scalable to large-scale HSI data. Extensive experiments on three benchmark data sets demonstrate that the proposed GCRVFL is able to achieve competitive results with fewer trainable parameters and adjustable hyperparameters and higher computational efficiency. In particular, we show that our approach is comparable to many existing approaches, including deep CNN models (e.g., ResNet and DenseNet) and popular GCN models (e.g., SGC and APPNP).
更多查看译文
关键词
graph convolutional network,graph-level classification,hyperspectral image,RVFL network
AI 理解论文
溯源树
样例
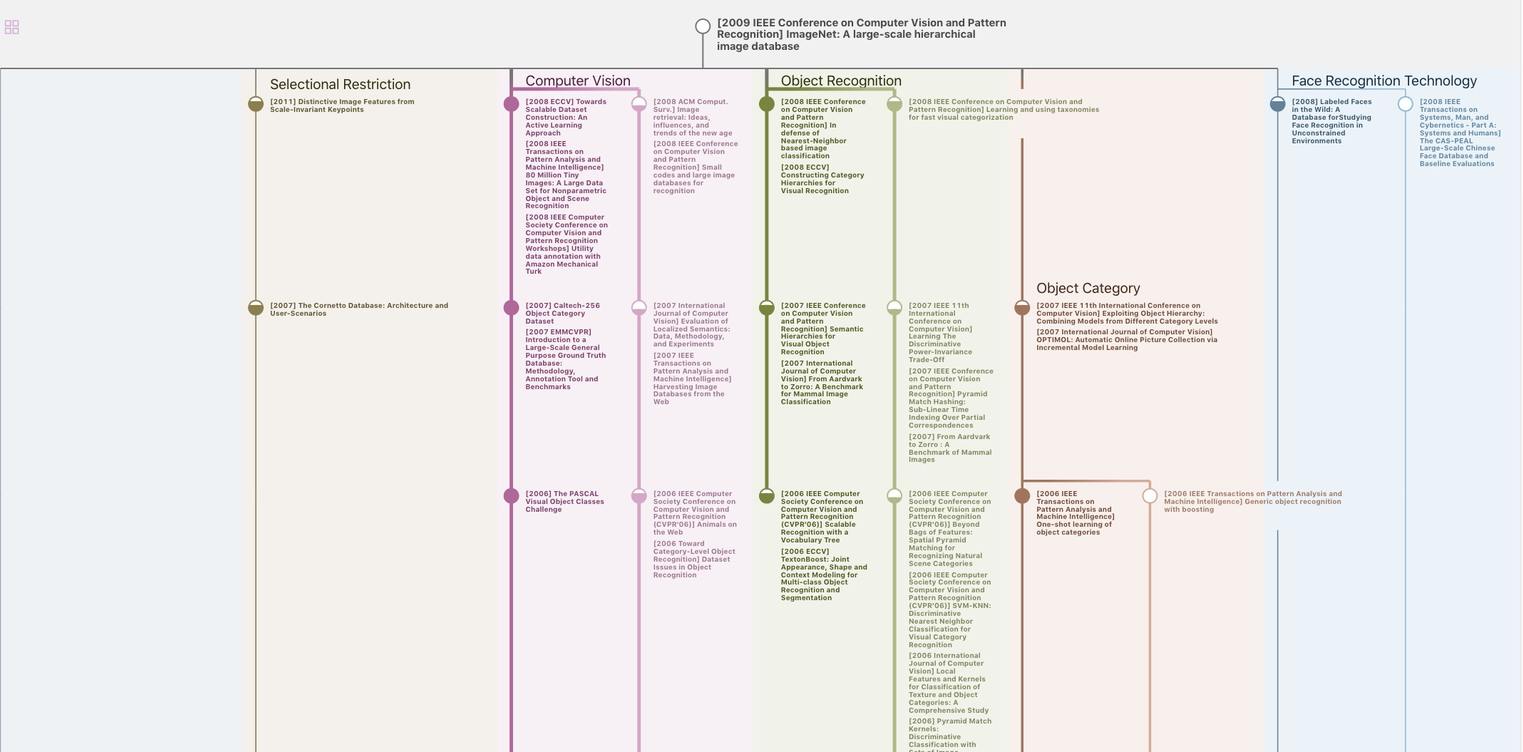
生成溯源树,研究论文发展脉络
Chat Paper
正在生成论文摘要