A Deep Neural Networks-Based Sound Speed Reconstruction with Enhanced Generalization by Training on a Natural Image Dataset
APPLIED SCIENCES-BASEL(2024)
摘要
Sound speed reconstruction has been investigated for quantitative evaluation of tissue properties in breast examination. Full waveform inversion (FWI), a mainstream method for conventional sound speed reconstruction, is an iterative method that includes numerical simulation of wave propagation, resulting in high computational cost. In contrast, high-speed reconstruction of sound speed using a deep neural network (DNN) has been proposed in recent years. Although the generalization performance is highly dependent on the training data, how to generate data for sufficient generalization performance is still unclear. In this study, the quality and generalization performance of DNN-based sound speed reconstruction with a ring array transducer were evaluated on a natural image-derived dataset and a breast phantom dataset. The DNN trained on breast phantom data (BP-DNN) could not reconstruct the structures on natural image data with diverse structures. On the other hand, the DNN trained on natural image data (NI-DNN) successfully reconstructed the structures on both natural image and breast phantom test data. Furthermore, the NI-DNN successfully reconstructed tumour structures in the breast, while the BP-DNN overlooked them. From these results, it was demonstrated that natural image data enables DNNs to learn sound speed reconstruction with high generalization performance and high resolution.
更多查看译文
关键词
breast cancer,deep learning,natural image,numerical simulation,ultrasound computed tomography
AI 理解论文
溯源树
样例
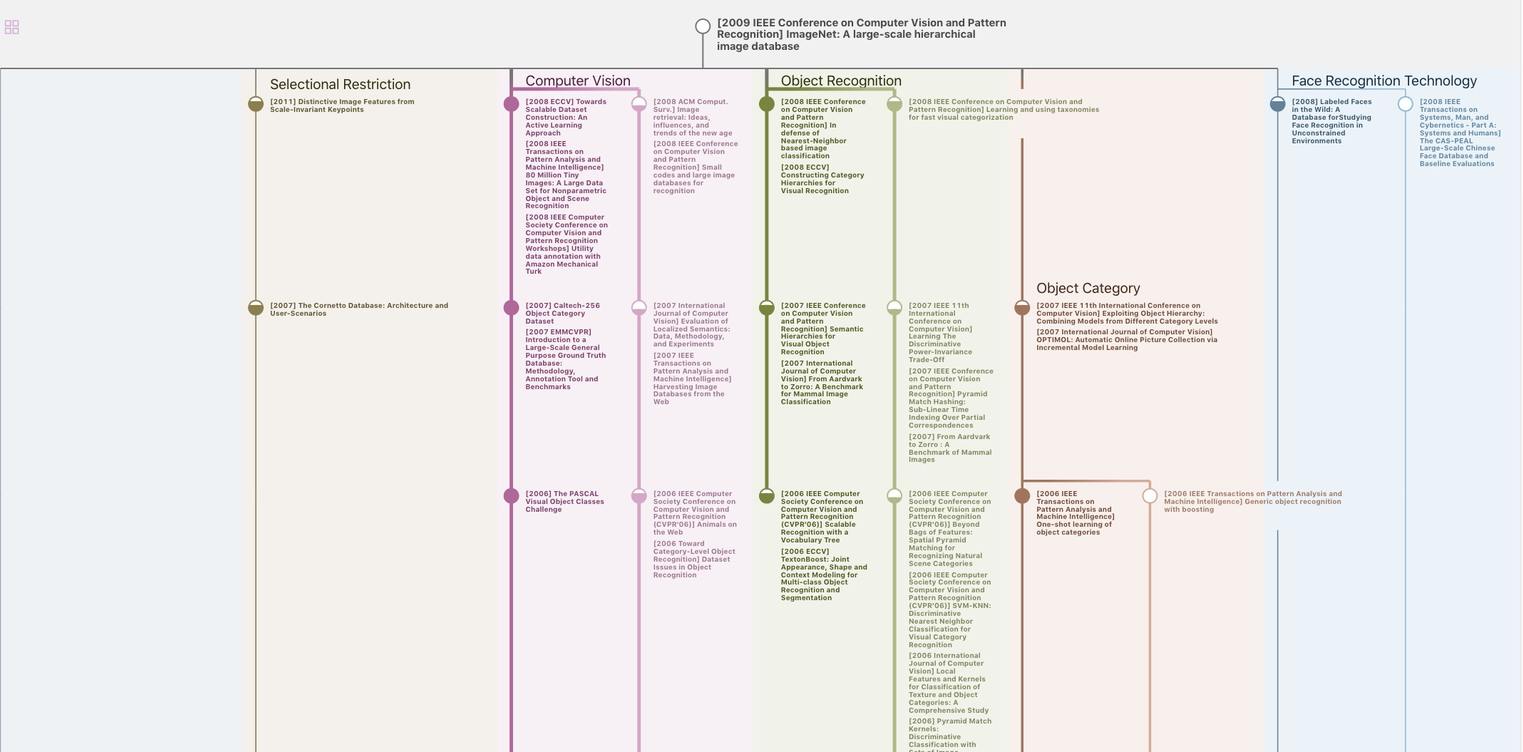
生成溯源树,研究论文发展脉络
Chat Paper
正在生成论文摘要