COVID-19 vaccine prediction based on an interpretable CNN-LSTM model with three-stage feature engineering
Health and Technology(2024)
摘要
Purpose Vaccine supply planning remains a critical and challenging issue for developing countries due to the limited resources and fluctuating vaccine demand, reflected by the sudden increase and decrease in the number of vaccinated people. Therefore, it is essential to accurately predict vaccination rates and interpret the prediction results to anticipate vaccine demand. Previous research focused on the prediction of vaccination rates by considering vaccination data and overlooked the importance of including features that highly affect the prediction results. Methods This research proposes an interpretable Convolutional Neural Network and Long Short-term Memory (CNN-LSTM) model for COVID-19 vaccination rate prediction, specifically for developing countries. The proposed model includes a three-stage feature engineering process that utilizes domain knowledge to construct new features of disease spread and the strictness of government policies. The temporal correlation of the features is captured to improve the prediction accuracy. Results The case studies were conducted on Nigeria, Nepal, and Peru’s datasets to ensure the model’s effectiveness and generalizability. The results indicate the effectiveness of the model, showing an average 30% decrease in the Mean Absolute Percentage Error (MAPE) with the enhanced feature engineering process and 16% additional improvement after combining the CNN with LSTM compared to the standalone LSTM model. Conclusion The interpretability of the CNN-LSTM model is a key aspect considered in this research to explain the prediction results. The model’s interpretability aims to identify important features affecting the prediction results and provide government and vaccine companies with guidelines in the decision-making for vaccine supply planning in developing countries. Graphical Abstract
更多查看译文
关键词
Vaccination rates,Vaccine demand,Hybrid CNN-LSTM,Interpretable model
AI 理解论文
溯源树
样例
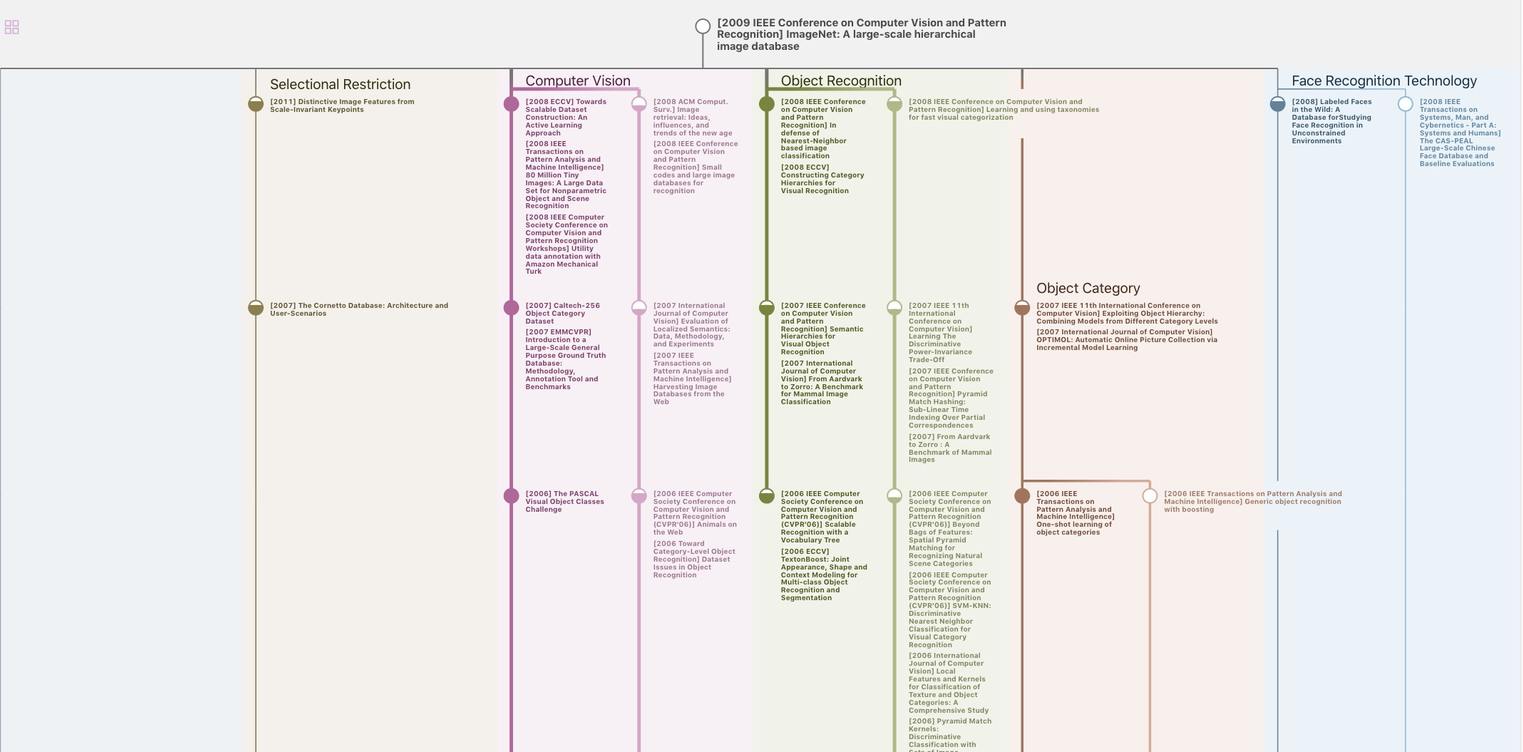
生成溯源树,研究论文发展脉络
Chat Paper
正在生成论文摘要