Stochastic dynamics of aircraft ground taxiing via improved physics-informed neural networks
Nonlinear Dynamics(2024)
摘要
In this paper, the stochastic propagation of the aircraft taxiing under the excitation of uneven runway is investigated based on physics-informed neural networks (PINNs). In particular, we successfully applied the PINNs with layer-wise locally adaptive activation functions (L-LAAF) and the learning rate decay strategy to address the challenging task of parameter identification for some aircraft systems. Specifically, the accuracy and effectiveness of the proposed method in solving the time-dependent Fokker–Planck equation for systems were first demonstrated. Subsequently, the proposed method is effectively utilized to identify the damping coefficient of landing gear and the aircraft body weight. Through numerical experiments and comparisons, we have demonstrated that incorporating L-LAAF and learning rate decay strategies can further enhance the performance of the network. The numerical simulation based on Monte Carlo fully validates the method. The development of physics-based deep learning techniques for aircraft system parameter identification research can help researchers better understand and control the behavior of systems, providing effective solutions for optimizing system design.
更多查看译文
关键词
PINNs,Aircraft ground taxiing model,Fokker–Planck equations,Inverse problem
AI 理解论文
溯源树
样例
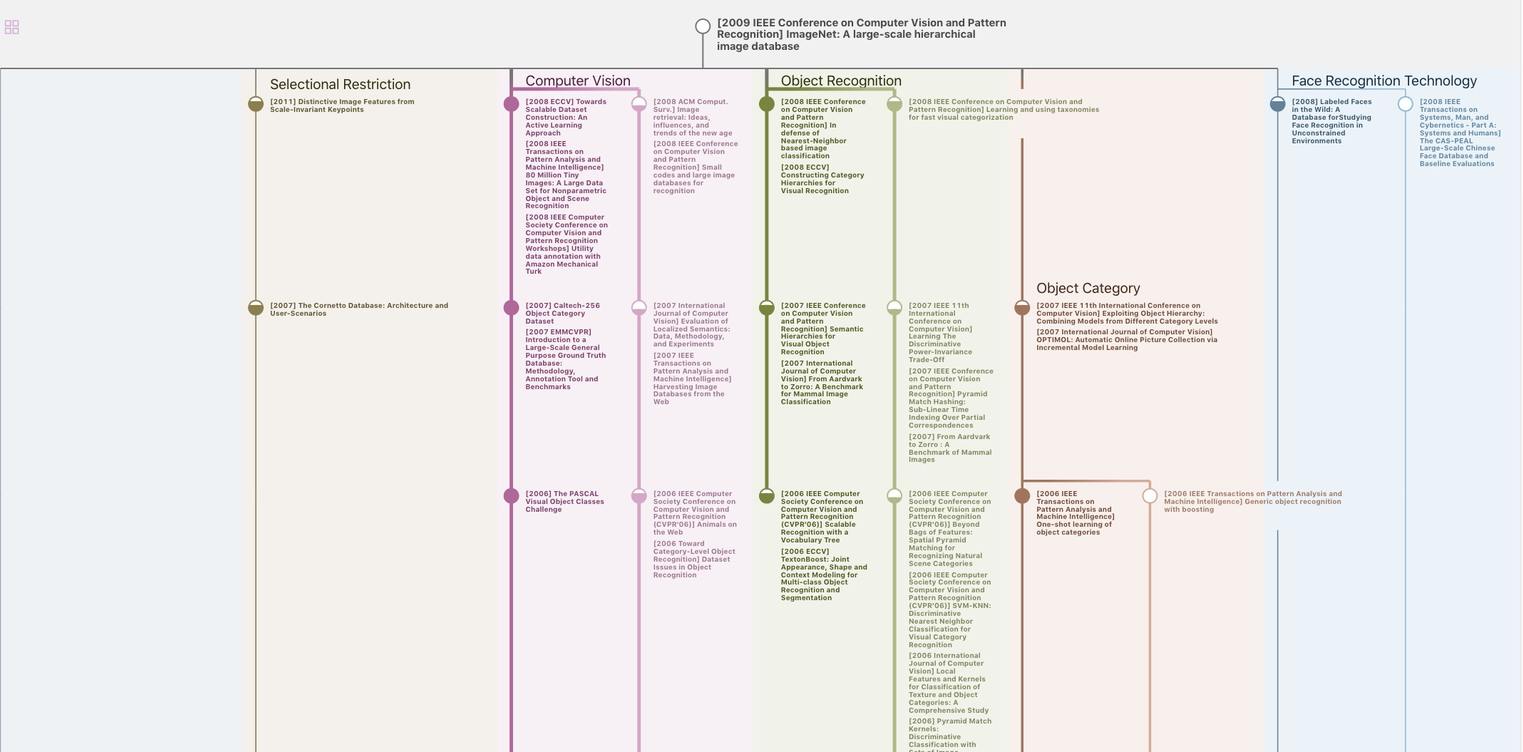
生成溯源树,研究论文发展脉络
Chat Paper
正在生成论文摘要