Unsupervised Coordinate-Based Neural Network for Electrical Impedance Tomography
IEEE TRANSACTIONS ON COMPUTATIONAL IMAGING(2023)
摘要
This study proposes an unsupervised deep learning approach to solve highly ill-posed inverse problems in electrical impedance tomography (EIT). A coordinate-based neural network is utilized to represent the conductivity distribution under investigation in EIT. The input to the network consists of finite element (FE) coordinates that describe the body being imaged. To enhance feature representation, these FE coordinates are projected onto Fourier features before being fed into the neural network. The output of the neural network provides an estimation of the internal conductivity distribution of the body. By its unsupervised nature, the method enables efficient and flexible learning without the need for labeled training data. The effectiveness of the proposed approach is evaluated through simulation and real data experiments, comparing it to state-of-the-art methods. The results demonstrate that the approach can achieve high-quality reconstruction results. This study also includes an ablation study and robustness analysis of the method, considering different hyperparameters, to ensure its reliability and consistency under varying conditions. Additionally, a spectral measurement of the reconstruction process is provided to gain further insights into the proposed approach.
更多查看译文
关键词
Coordinate-based neural representation,electrical impedance tomography,fourier feature projection,inverse problem,unsupervised learning
AI 理解论文
溯源树
样例
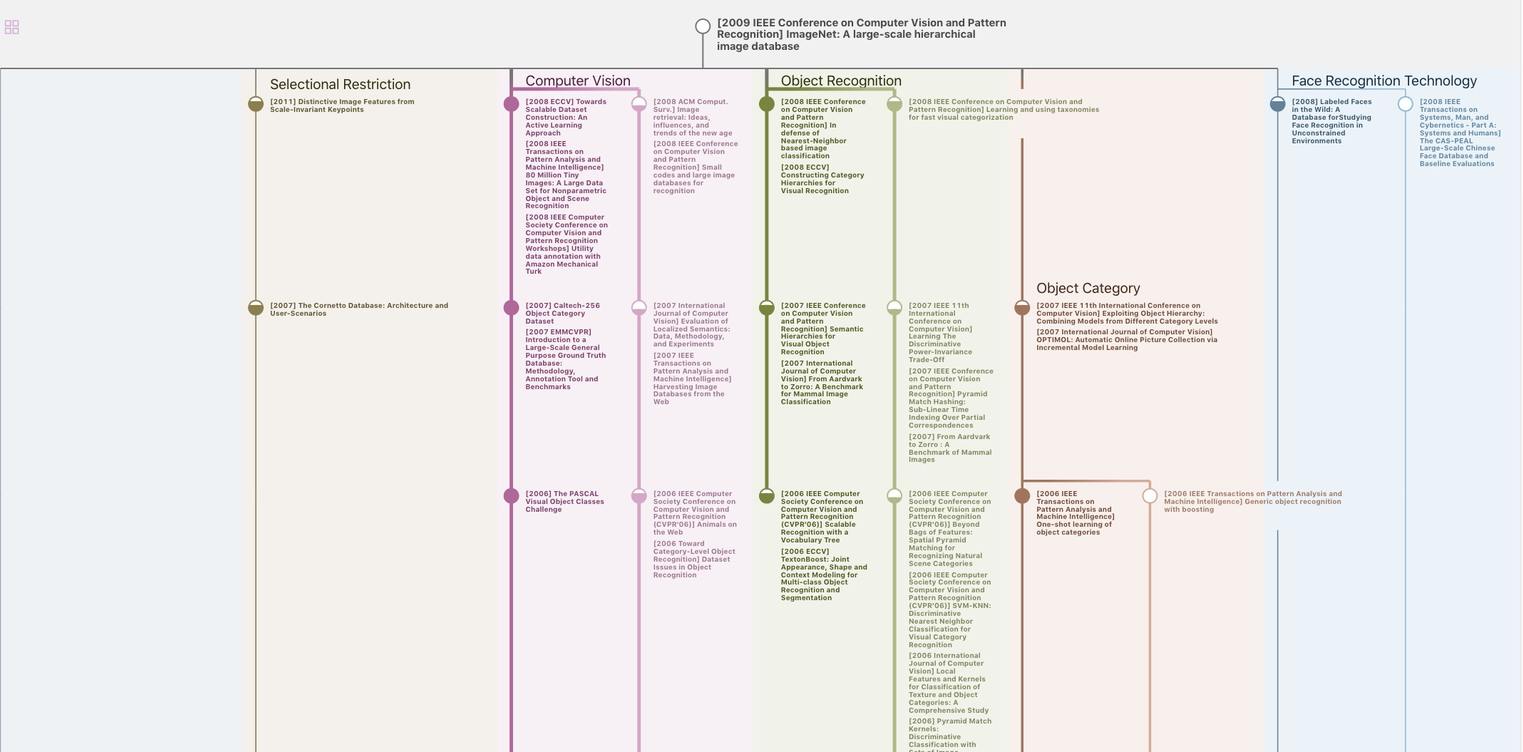
生成溯源树,研究论文发展脉络
Chat Paper
正在生成论文摘要