GenECG: A Synthetic Image-Based ECG Dataset to Augment Artificial Intelligence-Enhanced Algorithm Development
medrxiv(2023)
Abstract
Artificial intelligence-enhanced electrocardiogram (AI-ECG) analysis has the potential to transform care of cardiovascular disease patients. Most algorithms rely on digitised signal data and are unable to analyse paper-based ECGs, which remain in use in numerous clinical settings. An image-based ECG dataset incorporating artefacts common to paper-based ECGs, which are typically scanned or photographed into electronic health records, could facilitate development of clinically useful image-based algorithms. This paper describes the creation of GenECG, a high-fidelity, synthetic image-based dataset containing 21,799 ECGs with artefacts encountered in routine care. Iterative clinical Turing tests confirmed the realism of the synthetic ECGs: expert observer accuracy of discrimination between real-world and synthetic ECGs fell from 63.9% (95% CI 58.0%- 69.8%) to 53.3% (95% CI: 48.6%-58.1%) over three rounds of testing, indicating that observers could not distinguish between synthetic and real ECGs. GenECG is the first publicly available synthetic image-based ECG dataset to pass a clinical Turing test. The dataset will enable image-based AI-ECG algorithm development, ensuring the translation of AI-ECG research developments to the clinical workspace. ### Competing Interest Statement The authors have declared no competing interest. ### Funding Statement The study was funded by a University of Edinburgh Wellcome Trust iTPA award. The authors acknowledge the support of the British Heart Foundation Centre for Research Excellence Award III (RE/18/5/34216). The authors acknowledge the support of the British Heart Foundation (RG/20/4/34803). SEW is supported by the British Heart Foundation (FS/20/26/34952). IK is supported by the British Heart Foundation (FS/CRTF/21/24166). ### Author Declarations I confirm all relevant ethical guidelines have been followed, and any necessary IRB and/or ethics committee approvals have been obtained. Yes The details of the IRB/oversight body that provided approval or exemption for the research described are given below: The Ethics committee/IRB of King's College London gave ethical approval for the clinical Turing tests performed as part of this study (LRS-22/23-38259). I confirm that all necessary patient/participant consent has been obtained and the appropriate institutional forms have been archived, and that any patient/participant/sample identifiers included were not known to anyone (e.g., hospital staff, patients or participants themselves) outside the research group so cannot be used to identify individuals. Yes I understand that all clinical trials and any other prospective interventional studies must be registered with an ICMJE-approved registry, such as ClinicalTrials.gov. I confirm that any such study reported in the manuscript has been registered and the trial registration ID is provided (note: if posting a prospective study registered retrospectively, please provide a statement in the trial ID field explaining why the study was not registered in advance). Yes I have followed all appropriate research reporting guidelines, such as any relevant EQUATOR Network research reporting checklist(s) and other pertinent material, if applicable. Yes The ECG images described in the study were created from the PTB-XL database. The ECG images will be used for a British Heart Foundation Data Science Centre open challenge (). Following this challenge, Dataset A and B will be made publicly available via a Creative Commons license.
MoreTranslated text
AI Read Science
Must-Reading Tree
Example
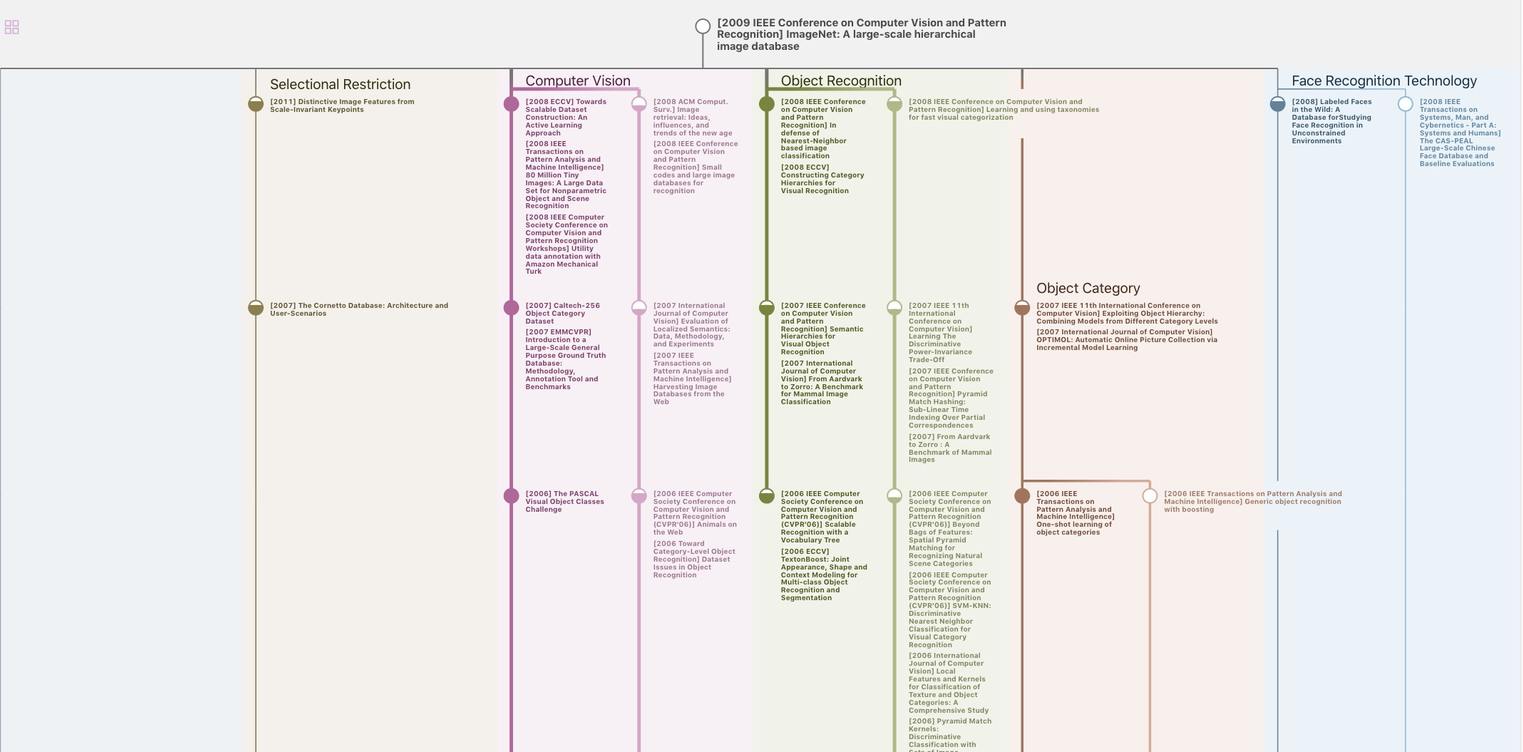
Generate MRT to find the research sequence of this paper
Chat Paper
Summary is being generated by the instructions you defined