Deep Reinforcement Multi-agent Learning framework for Information Gathering with Local Gaussian Processes for Water Monitoring
CoRR(2024)
摘要
The conservation of hydrological resources involves continuously monitoring
their contamination. A multi-agent system composed of autonomous surface
vehicles is proposed in this paper to efficiently monitor the water quality. To
achieve a safe control of the fleet, the fleet policy should be able to act
based on measurements and to the the fleet state. It is proposed to use Local
Gaussian Processes and Deep Reinforcement Learning to jointly obtain effective
monitoring policies. Local Gaussian processes, unlike classical global Gaussian
processes, can accurately model the information in a dissimilar spatial
correlation which captures more accurately the water quality information. A
Deep convolutional policy is proposed, that bases the decisions on the
observation on the mean and variance of this model, by means of an information
gain reward. Using a Double Deep Q-Learning algorithm, agents are trained to
minimize the estimation error in a safe manner thanks to a Consensus-based
heuristic. Simulation results indicate an improvement of up to 24
the mean absolute error with the proposed models. Also, training results with
1-3 agents indicate that our proposed approach returns 20
average estimation errors for, respectively, monitoring water quality variables
and monitoring algae blooms, as compared to state-of-the-art approaches
更多查看译文
AI 理解论文
溯源树
样例
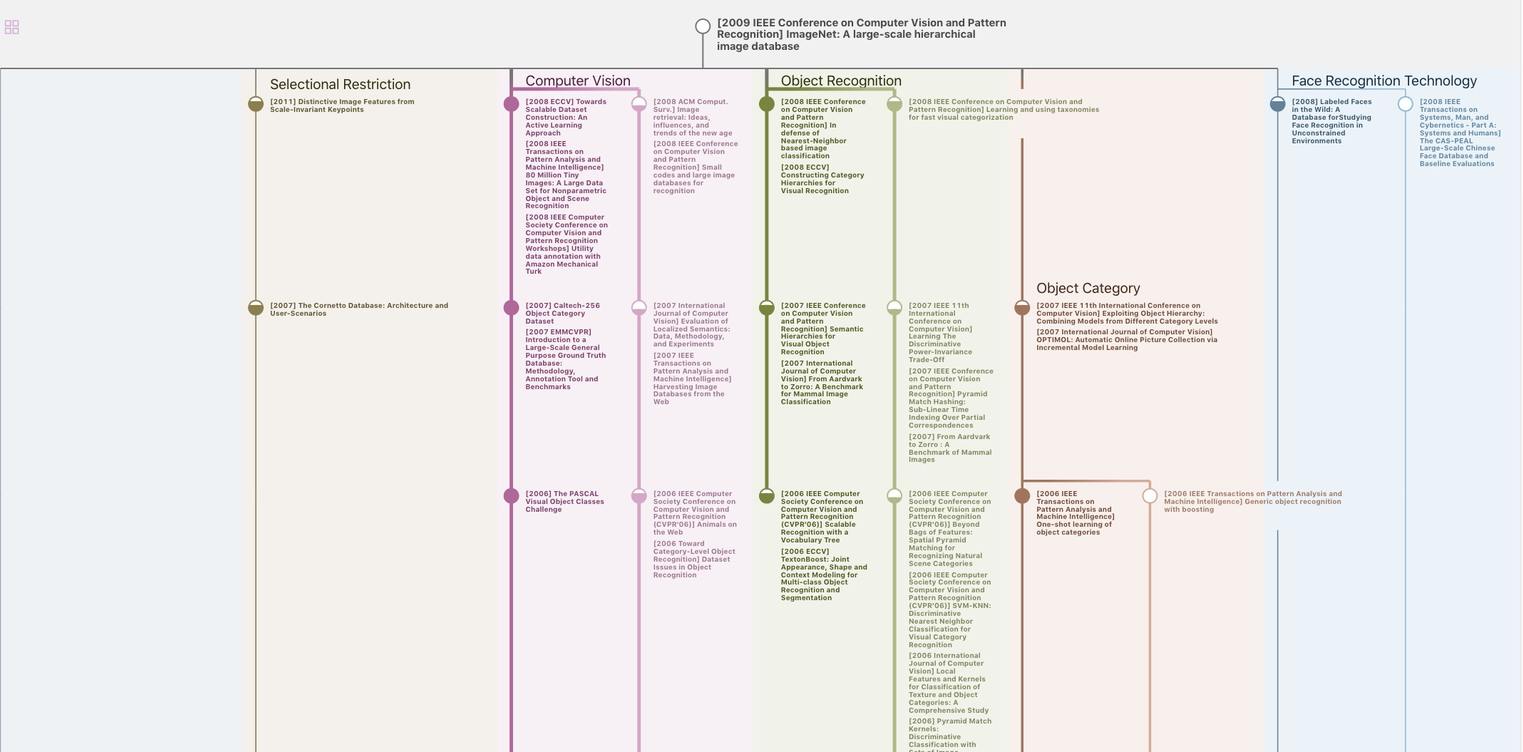
生成溯源树,研究论文发展脉络
Chat Paper
正在生成论文摘要