Cross-Speaker Encoding Network for Multi-Talker Speech Recognition
CoRR(2024)
摘要
End-to-end multi-talker speech recognition has garnered great interest as an
effective approach to directly transcribe overlapped speech from multiple
speakers. Current methods typically adopt either 1) single-input
multiple-output (SIMO) models with a branched encoder, or 2) single-input
single-output (SISO) models based on attention-based encoder-decoder
architecture with serialized output training (SOT). In this work, we propose a
Cross-Speaker Encoding (CSE) network to address the limitations of SIMO models
by aggregating cross-speaker representations. Furthermore, the CSE model is
integrated with SOT to leverage both the advantages of SIMO and SISO while
mitigating their drawbacks. To the best of our knowledge, this work represents
an early effort to integrate SIMO and SISO for multi-talker speech recognition.
Experiments on the two-speaker LibrispeechMix dataset show that the CES model
reduces word error rate (WER) by 8
reduces WER by 10
SOT model.
更多查看译文
关键词
multi-talker speech recognition,speech recognition,overlapped speech,speech separation,multi-speaker ASR
AI 理解论文
溯源树
样例
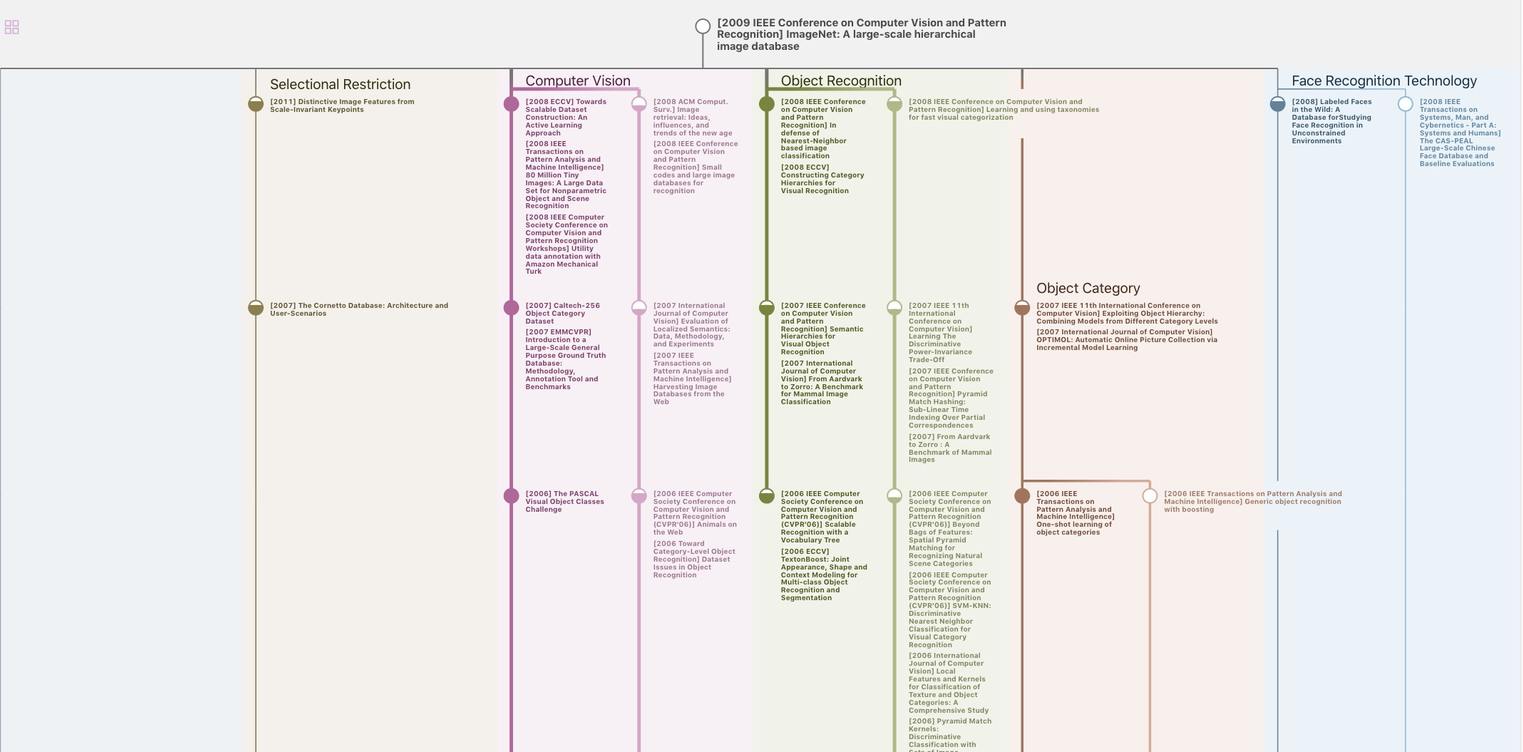
生成溯源树,研究论文发展脉络
Chat Paper
正在生成论文摘要